Open Access
ARTICLE
Attention-Based Residual Dense Shrinkage Network for ECG Denoising
1 Hunan Provincial Key Laboratory of Intelligent Processing of Big Data on Transportation, Changsha University of Science and Technology, Changsha, 410114, China
2 School of Computer and Communication Engineering, Changsha University of Science and Technology, Changsha, 410114, China
3 School of Computer and Artificial Intelligence, Huaihua University, Huaihua, 418000, China
4 School of Computer, National University of Defense Technology, Changsha, 410073, China
5 New Energy Centralized Control Center, CHN Energy Hunan Power New Energy Co., Ltd., Changsha, 410000, China
* Corresponding Author: Lebing Zhang. Email:
(This article belongs to the Special Issue: Computer Modeling of Artificial Intelligence and Medical Imaging)
Computer Modeling in Engineering & Sciences 2024, 138(3), 2809-2824. https://doi.org/10.32604/cmes.2023.029181
Received 06 February 2023; Accepted 02 August 2023; Issue published 15 December 2023
Abstract
Electrocardiogram (ECG) signal is one of the noninvasive physiological measurement techniques commonly used in cardiac diagnosis. However, in real scenarios, the ECG signal is susceptible to various noise erosion, which affects the subsequent pathological analysis. Therefore, the effective removal of the noise from ECG signals has become a top priority in cardiac diagnostic research. Aiming at the problem of incomplete signal shape retention and low signal-to-noise ratio (SNR) after denoising, a novel ECG denoising network, named attention-based residual dense shrinkage network (ARDSN), is proposed in this paper. Firstly, the shallow ECG characteristics are extracted by a shallow feature extraction network (SFEN). Then, the residual dense shrinkage attention block (RDSAB) is used for adaptive noise suppression. Finally, feature fusion representation (FFR) is performed on the hierarchical features extracted by a series of RDSABs to reconstruct the de-noised ECG signal. Experiments on the MIT-BIH arrhythmia database and MIT-BIH noise stress test database indicate that the proposed scheme can effectively resist the interference of different sources of noise on the ECG signal.Keywords
Cite This Article
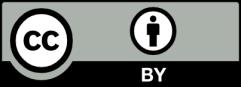