Open Access
ARTICLE
A Differential Privacy Federated Learning Scheme Based on Adaptive Gaussian Noise
1
College of Automation and Information Engineering, Sichuan University of Science and Engineering, Yibin, 644000, China
2
Sanjiang Research Institute of Artificial Intelligence and Robotics, Yibin University, Yibin, 644000, China
3
College of Mechanical Engineering, Sichuan University of Science and Engineering, Yibin, 644000, China
* Corresponding Author: Lecai Cai. Email:
(This article belongs to the Special Issue: Federated Learning Algorithms, Approaches, and Systems for Internet of Things)
Computer Modeling in Engineering & Sciences 2024, 138(2), 1679-1694. https://doi.org/10.32604/cmes.2023.030512
Received 10 April 2023; Accepted 12 July 2023; Issue published 17 November 2023
Abstract
As a distributed machine learning method, federated learning (FL) has the advantage of naturally protecting data privacy. It keeps data locally and trains local models through local data to protect the privacy of local data. The federated learning method effectively solves the problem of artificial Smart data islands and privacy protection issues. However, existing research shows that attackers may still steal user information by analyzing the parameters in the federated learning training process and the aggregation parameters on the server side. To solve this problem, differential privacy (DP) techniques are widely used for privacy protection in federated learning. However, adding Gaussian noise perturbations to the data degrades the model learning performance. To address these issues, this paper proposes a differential privacy federated learning scheme based on adaptive Gaussian noise (DPFL-AGN). To protect the data privacy and security of the federated learning training process, adaptive Gaussian noise is specifically added in the training process to hide the real parameters uploaded by the client. In addition, this paper proposes an adaptive noise reduction method. With the convergence of the model, the Gaussian noise in the later stage of the federated learning training process is reduced adaptively. This paper conducts a series of simulation experiments on real MNIST and CIFAR-10 datasets, and the results show that the DPFL-AGN algorithm performs better compared to the other algorithms.Graphic Abstract
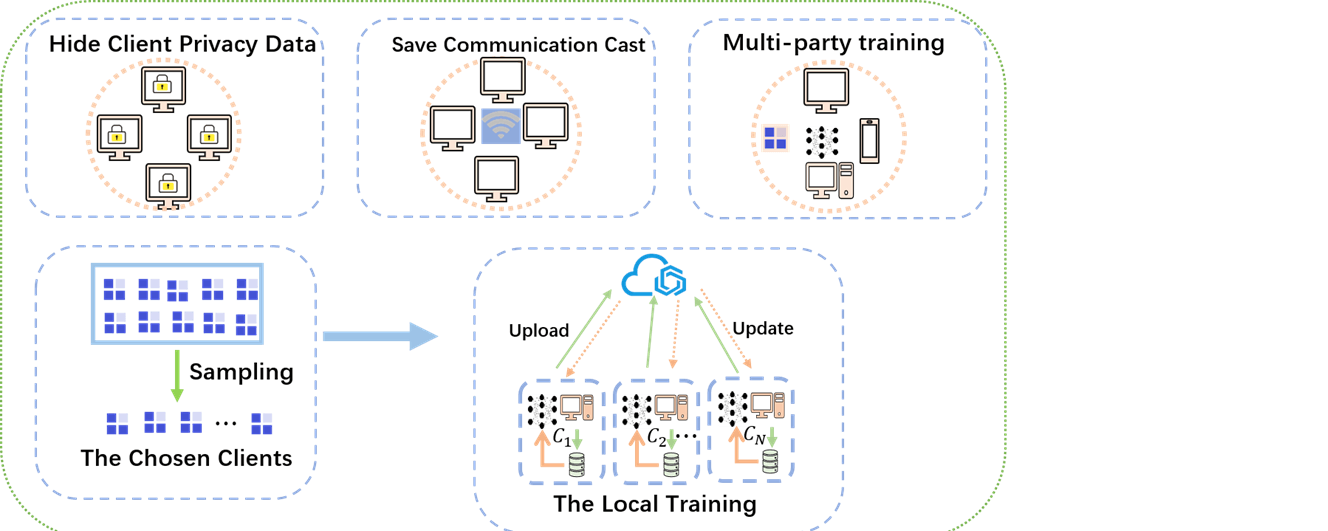
Keywords
Cite This Article
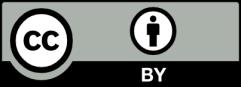
This work is licensed under a Creative Commons Attribution 4.0 International License , which permits unrestricted use, distribution, and reproduction in any medium, provided the original work is properly cited.