Open Access
ARTICLE
Gradient Optimizer Algorithm with Hybrid Deep Learning Based Failure Detection and Classification in the Industrial Environment
1 Department of Electrical and Computer Engineering (ECE), King Abdulaziz University, Jeddah, 21589, Saudi Arabia
2 Center of Excellence in Intelligent Engineering Systems (CEIES), King Abdulaziz University, Jeddah, 21589, Saudi Arabia
3 Department of Nuclear Engineering, King Abdulaziz University, Jeddah, 21589, Saudi Arabia
4 Department of Electrical and Electronic Engineering, Rajshahi University of Engineering and Technology, Rajshahi, Bangladesh
* Corresponding Author: Ibrahim M. Mehedi. Email:
(This article belongs to the Special Issue: Failure Detection Algorithms, Methods and Models for Industrial Environments)
Computer Modeling in Engineering & Sciences 2024, 138(2), 1341-1364. https://doi.org/10.32604/cmes.2023.030037
Received 20 March 2023; Accepted 06 July 2023; Issue published 17 November 2023
Abstract
Failure detection is an essential task in industrial systems for preventing costly downtime and ensuring the seamless operation of the system. Current industrial processes are getting smarter with the emergence of Industry 4.0. Specifically, various modernized industrial processes have been equipped with quite a few sensors to collect process-based data to find faults arising or prevailing in processes along with monitoring the status of processes. Fault diagnosis of rotating machines serves a main role in the engineering field and industrial production. Due to the disadvantages of existing fault, diagnosis approaches, which greatly depend on professional experience and human knowledge, intellectual fault diagnosis based on deep learning (DL) has attracted the researcher’s interest. DL reaches the desired fault classification and automatic feature learning. Therefore, this article designs a Gradient Optimizer Algorithm with Hybrid Deep Learning-based Failure Detection and Classification (GOAHDL-FDC) in the industrial environment. The presented GOAHDL-FDC technique initially applies continuous wavelet transform (CWT) for preprocessing the actual vibrational signals of the rotating machinery. Next, the residual network (ResNet18) model was exploited for the extraction of features from the vibration signals which are then fed into the HDL model for automated fault detection. Finally, the GOA-based hyperparameter tuning is performed to adjust the parameter values of the HDL model accurately. The experimental result analysis of the GOAHDL-FDC algorithm takes place using a series of simulations and the experimentation outcomes highlight the better results of the GOAHDL-FDC technique under different aspects.Keywords
Cite This Article
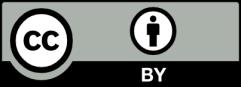
This work is licensed under a Creative Commons Attribution 4.0 International License , which permits unrestricted use, distribution, and reproduction in any medium, provided the original work is properly cited.