Open Access
ARTICLE
Adaptive H∞ Filtering Algorithm for Train Positioning Based on Prior Combination Constraints
1 Department of Mechanical Engineering, Henan Institute of Technology, Xinxiang, 453000, China
2 School of Electrical and Information Engineering, Dalian Jiaotong University, Dalian, 116028, China
* Corresponding Author: Pengfei Wang. Email:
(This article belongs to the Special Issue: Computer-Aided Uncertainty Modeling and Reliability Evaluation for Complex Engineering Structures)
Computer Modeling in Engineering & Sciences 2024, 138(2), 1795-1812. https://doi.org/10.32604/cmes.2023.030008
Received 18 March 2023; Accepted 20 June 2023; Issue published 17 November 2023
Abstract
To solve the problem of data fusion for prior information such as track information and train status in train positioning, an adaptive H∞ filtering algorithm with combination constraint is proposed, which fuses prior information with other sensor information in the form of constraints. Firstly, the train precise track constraint method of the train is proposed, and the plane position constraint and train motion state constraints are analysed. A model for combining prior information with constraints is established. Then an adaptive H∞ filter with combination constraints is derived based on the adaptive adjustment method of the robustness factor. Finally, the positioning effect of the proposed algorithm is simulated and analysed under the conditions of a straight track and a curved track. The results show that the positioning accuracy of the algorithm with constrained filtering is significantly better than that of the algorithm without constrained filtering and that the algorithm with constrained filtering can achieve better performance when combined with track and condition information, which can significantly reduce the train positioning error. The effectiveness of the proposed algorithm is verified.Keywords
Cite This Article
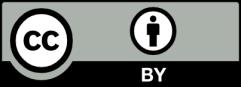
This work is licensed under a Creative Commons Attribution 4.0 International License , which permits unrestricted use, distribution, and reproduction in any medium, provided the original work is properly cited.