Open Access
ARTICLE
An Intelligent Sensor Data Preprocessing Method for OCT Fundus Image Watermarking Using an RCNN
1 College of Biomedical Information and Engineering, Hainan Medical University, Haikou, 571199, China
2 TJ-YZ School of Network Science, Haikou University of Economics, Haikou, 571127, China
3 Department of Earth System Science, Ministry of Education Key Laboratory for Earth System Modeling, Institute for Global Change Studies, Tsinghua University, Beijing, 100084, China
* Corresponding Author: Qiong Chen. Email:
(This article belongs to the Special Issue: AI-Driven Intelligent Sensor Networks: Key Enabling Theories, Architectures, Modeling, and Techniques)
Computer Modeling in Engineering & Sciences 2024, 138(2), 1549-1561. https://doi.org/10.32604/cmes.2023.029631
Received 28 February 2023; Accepted 29 May 2023; Issue published 17 November 2023
Abstract
Watermarks can provide reliable and secure copyright protection for optical coherence tomography (OCT) fundus images. The effective image segmentation is helpful for promoting OCT image watermarking. However, OCT images have a large amount of low-quality data, which seriously affects the performance of segmentation methods. Therefore, this paper proposes an effective segmentation method for OCT fundus image watermarking using a rough convolutional neural network (RCNN). First, the rough-set-based feature discretization module is designed to preprocess the input data. Second, a dual attention mechanism for feature channels and spatial regions in the CNN is added to enable the model to adaptively select important information for fusion. Finally, the refinement module for enhancing the extraction power of multi-scale information is added to improve the edge accuracy in segmentation. RCNN is compared with CE-Net and MultiResUNet on 83 gold standard 3D retinal OCT data samples. The average dice similarly coefficient (DSC) obtained by RCNN is 6% higher than that of CE-Net. The average 95 percent Hausdorff distance (95HD) and average symmetric surface distance (ASD) obtained by RCNN are 32.4% and 33.3% lower than those of MultiResUNet, respectively. We also evaluate the effect of feature discretization, as well as analyze the initial learning rate of RCNN and conduct ablation experiments with the four different models. The experimental results indicate that our method can improve the segmentation accuracy of OCT fundus images, providing strong support for its application in medical image watermarking.Keywords
Cite This Article
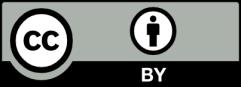
This work is licensed under a Creative Commons Attribution 4.0 International License , which permits unrestricted use, distribution, and reproduction in any medium, provided the original work is properly cited.