Open Access
ARTICLE
Learning Discriminatory Information for Object Detection on Urine Sediment Image
1 College of Computer Science and Technology, Zhejiang University of Technology, Hangzhou, 310023, China
2 The Hefei Institutes of Physical Science, Chinese Academy of Sciences, Hefei, 230071, China
3 Department of Digital Media Technology, Hangzhou Dianzi University, Hangzhou, 310018, China
4 School of Computer Science, University of Nottingham Ningbo China, Ningbo, 315100, China
* Corresponding Author: Hongqiang Wang. Email:
(This article belongs to the Special Issue: Computer Modeling of Artificial Intelligence and Medical Imaging)
Computer Modeling in Engineering & Sciences 2024, 138(1), 411-428. https://doi.org/10.32604/cmes.2023.029485
Received 22 February 2023; Accepted 05 May 2023; Issue published 22 September 2023
Abstract
In clinical practice, the microscopic examination of urine sediment is considered an important in vitro examination with many broad applications. Measuring the amount of each type of urine sediment allows for screening, diagnosis and evaluation of kidney and urinary tract disease, providing insight into the specific type and severity. However, manual urine sediment examination is labor-intensive, time-consuming, and subjective. Traditional machine learning based object detection methods require hand-crafted features for localization and classification, which have poor generalization capabilities and are difficult to quickly and accurately detect the number of urine sediments. Deep learning based object detection methods have the potential to address the challenges mentioned above, but these methods require access to large urine sediment image datasets. Unfortunately, only a limited number of publicly available urine sediment datasets are currently available. To alleviate the lack of urine sediment datasets in medical image analysis, we propose a new dataset named UriSed2K, which contains 2465 high-quality images annotated with expert guidance. Two main challenges are associated with our dataset: a large number of small objects and the occlusion between these small objects. Our manuscript focuses on applying deep learning object detection methods to the urine sediment dataset and addressing the challenges presented by this dataset. Specifically, our goal is to improve the accuracy and efficiency of the detection algorithm and, in doing so, provide medical professionals with an automatic detector that saves time and effort. We propose an improved lightweight one-stage object detection algorithm called Discriminatory-YOLO. The proposed algorithm comprises a local context attention module and a global background suppression module, which aid the detector in distinguishing urine sediment features in the image. The local context attention module captures context information beyond the object region, while the global background suppression module emphasizes objects in uninformative backgrounds. We comprehensively evaluate our method on the UriSed2K dataset, which includes seven categories of urine sediments, such as erythrocytes (red blood cells), leukocytes (white blood cells), epithelial cells, crystals, mycetes, broken erythrocytes, and broken leukocytes, achieving the best average precision (AP) of 95.3% while taking only 10 ms per image. The source code and dataset are available at .Graphic Abstract
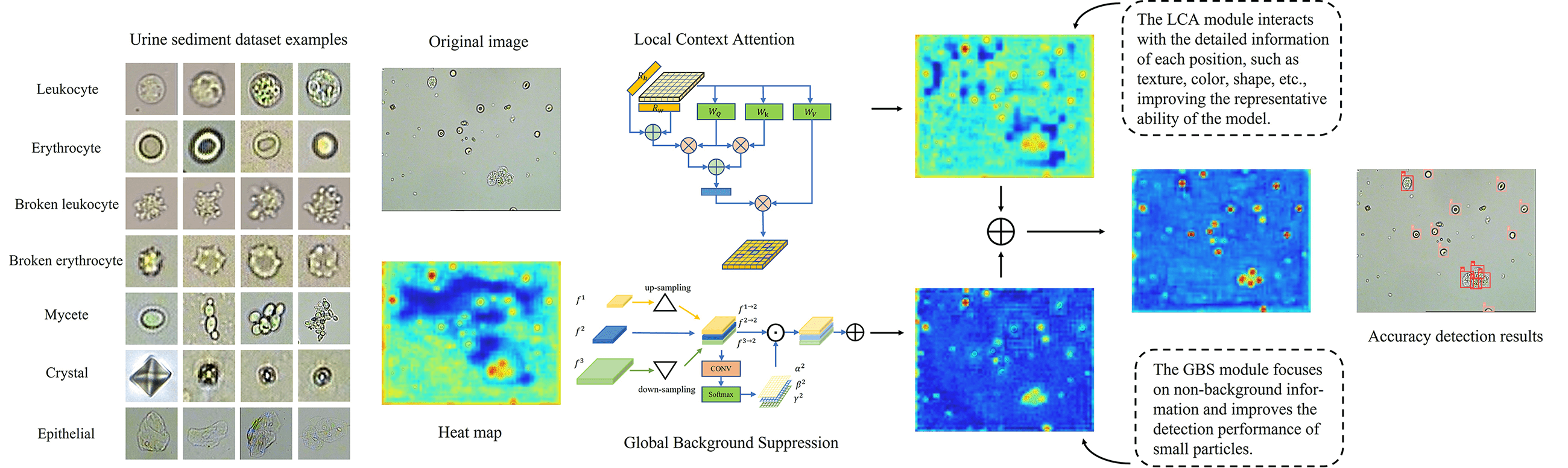
Keywords
Cite This Article
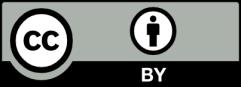
This work is licensed under a Creative Commons Attribution 4.0 International License , which permits unrestricted use, distribution, and reproduction in any medium, provided the original work is properly cited.