Open Access
ARTICLE
Federated Learning Model for Auto Insurance Rate Setting Based on Tweedie Distribution
1 State Key Laboratory of Public Big Data, Guizhou University, Guiyang, 550025, China
2 Guizhou Big Data Academy, Guizhou University, Guiyang, 550025, China
3 Key Laboratory of Advanced Manufacturing Technology, Ministry of Education, Guizhou University, Guiyang, 550025, China
4 College of Computer Science and Technology, Guizhou University, Guiyang, 550025, China
5 ChinaDataPay Company, Guiyang, 550025, China
* Corresponding Author: Changgen Peng. Email:
(This article belongs to the Special Issue: Federated Learning Algorithms, Approaches, and Systems for Internet of Things)
Computer Modeling in Engineering & Sciences 2024, 138(1), 827-843. https://doi.org/10.32604/cmes.2023.029039
Received 28 January 2023; Accepted 08 May 2023; Issue published 22 September 2023
Abstract
In the assessment of car insurance claims, the claim rate for car insurance presents a highly skewed probability distribution, which is typically modeled using Tweedie distribution. The traditional approach to obtaining the Tweedie regression model involves training on a centralized dataset, when the data is provided by multiple parties, training a privacy-preserving Tweedie regression model without exchanging raw data becomes a challenge. To address this issue, this study introduces a novel vertical federated learning-based Tweedie regression algorithm for multi-party auto insurance rate setting in data silos. The algorithm can keep sensitive data locally and uses privacy-preserving techniques to achieve intersection operations between the two parties holding the data. After determining which entities are shared, the participants train the model locally using the shared entity data to obtain the local generalized linear model intermediate parameters. The homomorphic encryption algorithms are introduced to interact with and update the model intermediate parameters to collaboratively complete the joint training of the car insurance rate-setting model. Performance tests on two publicly available datasets show that the proposed federated Tweedie regression algorithm can effectively generate Tweedie regression models that leverage the value of data from both parties without exchanging data. The assessment results of the scheme approach those of the Tweedie regression model learned from centralized data, and outperform the Tweedie regression model learned independently by a single party.Keywords
Cite This Article
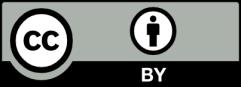