Open Access
ARTICLE
EfficientShip: A Hybrid Deep Learning Framework for Ship Detection in the River
1 School of Computer Engineering, Jingchu University of Technology, Jingmen, 448000, China
2 School of Computer Science, Yangtze University, Jingzhou, 434023, China
3 School of Computing and Mathematic Sciences, University of Leicester, Leicester, LE1 7RH, UK
* Corresponding Author: Yudong Zhang. Email:
Computer Modeling in Engineering & Sciences 2024, 138(1), 301-320. https://doi.org/10.32604/cmes.2023.028738
Received 05 January 2023; Accepted 05 May 2023; Issue published 22 September 2023
Abstract
Optical image-based ship detection can ensure the safety of ships and promote the orderly management of ships in offshore waters. Current deep learning researches on optical image-based ship detection mainly focus on improving one-stage detectors for real-time ship detection but sacrifices the accuracy of detection. To solve this problem, we present a hybrid ship detection framework which is named EfficientShip in this paper. The core parts of the EfficientShip are DLA-backboned object location (DBOL) and CascadeRCNN-guided object classification (CROC). The DBOL is responsible for finding potential ship objects, and the CROC is used to categorize the potential ship objects. We also design a pixel-spatial-level data augmentation (PSDA) to reduce the risk of detection model overfitting. We compare the proposed EfficientShip with state-of-the-art (SOTA) literature on a ship detection dataset called Seaships. Experiments show our ship detection framework achieves a result of 99.63% (mAP) at 45 fps, which is much better than 8 SOTA approaches on detection accuracy and can also meet the requirements of real-time application scenarios.Keywords
Cite This Article
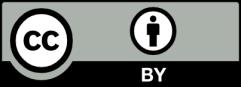
This work is licensed under a Creative Commons Attribution 4.0 International License , which permits unrestricted use, distribution, and reproduction in any medium, provided the original work is properly cited.