Open Access
ARTICLE
Resource Allocation for IRS Assisted mmWave Wireless Powered Sensor Networks with User Cooperation
1 Network Center, Zhengzhou University, Zhengzhou, 450001, China
2 School of Electrical and Information Engineering, Zhengzhou University, Zhengzhou, 450001, China
* Corresponding Author: Zhengyu Zhu. Email:
(This article belongs to the Special Issue: Recent Advances in Backscatter and Intelligent Reflecting Surface Communications for 6G-enabled Internet of Things Networks)
Computer Modeling in Engineering & Sciences 2024, 138(1), 663-677. https://doi.org/10.32604/cmes.2023.028584
Received 26 December 2022; Accepted 28 March 2023; Issue published 22 September 2023
Abstract
In this paper, we investigate IRS-aided user cooperation (UC) scheme in millimeter wave (mmWave) wireless-powered sensor networks (WPSN), where two single-antenna users are wireless powered in the wireless energy transfer (WET) phase first and then cooperatively transmit information to a hybrid access point (AP) in the wireless information transmission (WIT) phase, following which the IRS is deployed to enhance the system performance of the WET and WIT. We maximized the weighted sum-rate problem by jointly optimizing the transmit time slots, power allocations, and the phase shifts of the IRS. Due to the non-convexity of the original problem, a semidefinite programming relaxation-based approach is proposed to convert the formulated problem to a convex optimization framework, which can obtain the optimal global solution. Simulation results demonstrate that the weighted sum throughput of the proposed UC scheme outperforms the non-UC scheme whether equipped with IRS or not.Keywords
The rapid expansion of the number of mobile devices has brought higher requirements for wireless applications, such as more extensive radio coverage, lower latency, higher data transmission rates and higher security, while the emergence of sixth-generation (6G) wireless technologies can perfectly meet such demands [1,2] describe the vision of future 6G communication and network architecture, which presents emerging technologies such as artificial intelligence, blockchain, quantum communications, integration of wireless information and energy transfer, integrated sensing and communication, intelligent reflecting surface and big data. Millimeter-wave (mmWave) communication has been widely regarded as a key technology of 6G, which owns ultra-wide bandwidth and high transfer speed advantages to meet the enormous capacity demand for 6G wireless communications [3,4]. An exhaustive survey of mmWave wireless communications for future 6G networks is described in [5], where the latest channel measurement, modeling and multiple input multiple output (MIMO) transceiver designs are summarized.
Also, energy harvesting (EH), as one of the main techniques to power future 6G wireless networks, has been introduced as an efficient method that allows wireless devices to capture the energy from external environments [6,7]. Radio frequency (RF) EH technique can enable an aggressive energy supply to the wireless devices (WDs) [8]. As an promising and emerging EH technology, wireless power communication networks (WPCN) are of great interest as they have the potential to provide wireless power to energy-constrained devices such as the internet of things (IoT) and wireless sensor networks (WSN) to ensure their operation [9,10]. Compared to the traditional battery-powered way, WPCN always provides more reliable and sustainable power replenishment. In WPCNs, the placement optimization of power and information access points is researched, where the WDs harvest the RF energy in the downlink and employ the harvested energy to transmit information to a base station in the uplink [11,12].
To improve the radio coverage and signal quality in a green way, an intelligent reflecting surface (IRS) has been proposed to reshape the signal propagation environment via passive reflecting arrays [13,14]. The transmitted signal can be reflected the receiver through the IRS and constitutes the received signal together with the signal transmitted by the direct link [15]. Thus, the reflected signal can be enhanced by properly adjusting the passive components of IRS [16,17].
Recently, some research on IRS-aided wireless powered sensor networks (WPSN) has been studied in [18–38]. For maximizing the sum throughput in an IRS-aided WPCN, the closed-form solution for the phase shifts of the wireless information transfer has been derived, where a low complexity scheme was proposed to reduce the computational complexity of the semidefinite programming (SDP) scheme [18]. Considering user cooperation (UC) in an IRS-assisted WPCN, the maximum throughput performance has been derived by jointly optimizing the power allocations, IRS phase shifts and the transmit time [19]. By obtaining a closed-form solution by the Lagrange dual method and the Karush-Kuhn-Tucker conditions in an IRS-aided WPSN, Chu et al. [20] optimally designed the bandwidth allocation and the transmit time schedule. Li et al. [21] have maximized the minimum throughput performance for IRS-aided UC in a WPCN. To simultaneously improve the performance of uplink information transmission and downlink energy transfer, Lyu et al. [22] investigated a hybrid-relaying self-sustainable IRS WPCN. Zhai et al. [23] has considered multicast transmissions in a game-theoretic way in an IRS-aided WPCN, where the closed-form optimal phase shifts have been derived. For a backscatter-assisted WPCN with an IRS, Ramezani et al. [24] have studied the maximization of total network throughput through a two-stage algorithm. In an IRS-assisted multiuser MISO WPCN, Zheng et al. [25] have maximized the weighted sum rate of all the energy-harvesting users by applying a block-structured optimization method. A novel dynamic IRS beamforming framework has been proposed to boost the sum throughput of an IRS-aided WPCN in [26]. In order to strictly guarantee device quality-of-service (QoS) requirements, Zeng et al. [27] have considered the non-linear energy harvesting in an IRS WPCN. In a multi-user IRS WPCN, Cao et al. [28] have maximized the system throughput by optimizing jointly the energy beamforming, phase shift and time allocation. To maximize the sum throughput by applying the Lagrange dual method, a closed-form solution has been derived for the optimal power allocation, transmission time slots and phase shift for IRS-aided wireless powered IoT system [29].
To tackle the issue of the uplink transmission of WDs in WPCNs depending on the harvested energy from the downlink, Wang et al. [30] proposed an energy efficient optimization method in IRS-assisted WPCN by the variable-substitution approach. In order to ensure fairness, Zeng et al. [31] studied a weighted sum throughput maximization in an active IRS-aided WPCN by applying three beamforming setups. Using the semidefinite relaxation method, Lyu et al. [32] considered a sum rate maximization for the IRS-aided WPCN by jointly iteratively optimizing the time scheduling and the phase shift. In the presence of eavesdropper, Cao et al. [33] proposed three secure modes in physical layer security of an IRS-aided WPC system, where asymptotic ergodic secrecy capacity is analyzed and closed-form expression is obtained. A new transmission policy for an IRS-assisted WPSN with certain phase shifts is proposed by maximizing the system sum throughput in [34], in which IRS can collect energy to achieve its self-sustainability. In a MISO transmission form, a practical IRS can work in EH with a power budget constraint, and then utilize the harvesting energy to sustain its operations in the signal reflecting phase [35]. Considering cognitive loT devices, two transmission policies are studied with a successive interference cancellation technique [36], where the interaction between primary and secondary networks is characterized by a Stackelberg game strategy. An IRS-aided wireless powered over-the-air computation Internet-of-Things network is investigated to propose a joint design of energy beamforming at the access point, downlink and uplink phase-shift matrices, as well as transmit power, in order to minimize the mean-squared error [37]. With the aid of an IRS, in wireless powered non-orthogonal multiple access (NOMA) IoT networks, by jointly optimizing the time allocation and phase shift matrices of wireless energy transfer and wireless information transfer, a novel resource allocation approach is proposed by maximizing the sum throughput in [38].
Integrating the IRS technology with sensor networks can achieve a self-sustaining system, specifically by embedding the IRS to the existing WPSN to reflect energy and information signals to improve the wireless energy transfer (WET) and wireless information transfer (WIT) capabilities of the WPSN. Also, to achieve a better performance with the IRS-assisted scheme, we consider a mmWave WPSN UC system in the uplink, i.e., one user not only completes his own signal transmission task, but also assists other users in completing signal transmission as a relay. Different from the existing works on the IRS-aided WPCN system, the research of transmission strategies in an IRS-assisted WPSN systems with UC in the mmWave band has not been made yet. We summarize our contributions as follows:
1. First, an IRS has been used to improve the performance of the WPSN system with UC in the mmWave channel, where two single-antenna wireless devices can harvest energy radiated by a hybrid access point (AP) to support the separate information transmission to the AP. Here, the IRS play a role in promoting the system performance of the downlink wireless energy harvesting and the uplink wireless information transmission by energy and information reflection for the IRS, respectively.
2. Second, it aims to maximize the weighted sum-rate through jointly optimizing the power, the time slots allocation and the IRS phase shift of the WET and WIT is used to evaluate the performance of the proposed system model. Since the original problem is non-convex with respect to the coupled variables of the power and the IRS phase shift, it is hard to solve directly.
3. Finally, to deal with the non-convexity of the original formulated problem, the semidefinite programming relaxation (SDR) technique is applied to convert the weighted sum-rate maximization (WSRM) problem to a convex optimization framework, which can obtain the global optimal solution. Simulation results proved that the IRS and the UC can improve the system performance compared with the two latest benchmarks.
The rest of the paper is organized as follows. Section 2 introduces the system model of an IRS-aided WPSN system with mmWave channel. In Section 3, a weighted throughput maximization problem with user cooperation is discussed carefully, and we develop a SDR-based algorithm to solve the original problem. In Section 4, our simulation results demonstrate the effectiveness of the proposed SDR algorithm. In Section 5, the paper is summarized.
As shown in Fig. 1, we investigate an IRS-aided mmWave WPSN system, which contains a single-antenna AP and two single-antenna wireless users (WUs). Due to the constrained energy of devices, the wireless communication links (WCLs) of harvesting energy in the UL are established between AP and WUs. In the downlink (DL), information signals also can be transmitted through these links. Without loss of generality, we assume that WU2 is used as a relay between WU1 and AP in the meantime. An IRS with
Figure 1: The IRS-aided mmWave WPSN system model
For the mmWave channel of AP to IRS, IRS to WUk,
where
where
where
where
The time of the WIT phase is
Figure 2: System time allocation of WET and WIT
1. In the first sub-phase, i.e.,
2. In the second sub-phase, i.e.,
3. In the third sub-phase, i.e.,
In the first sub-phase, the harvested energy obtained in WET is used in WET and the transmit power of WU1 is
Then, the information signal received at AP and WU2 are expressed respectively as
where
In the second sub-phase, the signal received at AP given by
where
The information signals transmitted from WU1 to AP have four different paths, where the signal of AP in the first path is the direct link from WU
where
In the third sub-phase, the information signal received at AP is written as
where
The energy obtained by WU2 in
According to the above transmission process, the time period
3 Weighted Throughput Maximization with User Cooperation
Using the derivation and definition from the previous step, a WSRM problem is formulated based on a UC scheme and then solved by jointly optimizing the phase shift matrices, time allocation, and power allocation. First, the WSRM problem is given by
where
For the non-convexity of problem (P1), we can introduce some transformation forms for the phase shift matrices to tackle it. So, we have
where
where
Then, introducing auxiliary variables
where
Define
We further define
Accordingly, by introducing another auxiliary variable
Due to the rank-one constraint causing the problem to be non-convex, so we use the SDR approach to remove this constraint, then (P1) can be reformulated as
Here, the problem (34) is now a convex optimization problem. Thus, the global optimal solution can be obtained by CVX in Matlab [40,41], which is denoted as (
Combined with the above derivation, we can further obtain the optimal values as
The rank-one solution may be obtained from the relaxed WSRM (P3). So we employ the eigenvalue decomposition for
The simulation results are presented to evaluate the performance of the user cooperation scheme in IRS-aided mmWave WPSN. The illustration of the system is shown in Fig. 3. We assume that AP and WUk are on the same level, and the distance between the IRS and this horizontal line is 4 meters and the distance between AP and IRS is
Figure 3: Simulation model
1. IRS+UC: IRS helps WUs to harvest energy through reflection in the WET phase. In the WIT phase, IRS reflects signals from WUs to AP, and WU2 can receive and secondary transmit signals from WU1 to AP as a relay in the UC scheme.
2. Non IRS+UC: Following [12], WUs only harvest energy through the direct link from AP in the WET phase and the UC scheme is considered in the WIT.
3. IRS+Non UC: Following [26], Only IRS works and UC scheme is canceled.
4. Non IRS+Non UC: There are no IRS and UC schemes in the system.
We first evaluate the sum throughput vs. the distance
Figure 4: Sum throughput vs. d1
In order to observe the sum throughput improvement with increasing
Figure 5: Sum throughput vs. P0
Finally, the impact of the elements number
Figure 6: Sum throughput vs. N
In this paper, we proposed a UC scheme for IRS-aided mmWave WPSN. The WSRM problem is formulated to maximize the weighted sum throughout by jointly optimizing the phase shift matrix, time slots, and power allocation. Some efficient variable substitutions and SDP relaxation are introduced to convert the original non-convex problem into a convex problem that is easy to tackle. Finally, numerical results confirm that the weighted sum throughput of our proposed UC scheme is greater than that of non UC whether equipped with IRS or not.
Acknowledgement: This work was supported in part by the open research fund of National Mobile Communications Research Laboratory, Southeast University (No. 2023D11), in part by Sponsored by Program for Science & Technology Innovation Talents in Universities of Henan Province (23HASTIT019), in part by Natural Science Foundation of Henan Province (232300421097), in part by the Project funded by China Postdoctoral Science Foundation (2023T160596, 2020M682345), in part by the Henan Postdoctoral Foundation (202001015).
Funding Statement: This work was supported in part by the open research fund of National Mobile Communications Research Laboratory, Southeast University (No. 2023D11), in part by Sponsored by program for Science & Technology Innovation Talents in Universities of Henan Province (23HASTIT019), in part by Natural Science Foundation of Henan Province (20232300421097), in part by the project funded by China Postdoctoral Science Foundation (2020M682345), in part by the Henan Postdoctoral Foundation (202001015).
Author Contributions: The authors confirm contribution to the paper as follows: study conception and design: Yonghui Lin, Zhengyu Zhu; data collection: Yonghui Lin, Zhengyu Zhu; analysis and interpretation of results: Yonghui Lin, Zhengyu Zhu; draft manuscript preparation: Yonghui Lin. All authors reviewed the results and approved the final version of the manuscript.
Availability of Data and Materials: This statement should make clear how readers can access the data used in the study and explain why any unavailable data cannot be released.
Conflicts of Interest: The authors declare that they have no conflicts of interest to report regarding the present study.
References
1. Alsabah, M., Naser, M. A., Mahmmod, B. M., Abdulhussain, S. H., Eissa, M. R. et al. (2021). 6G wireless communications networks: A comprehensive survey. IEEE Access, 9, 148191–148243. [Google Scholar]
2. Chowdhury, M. Z., Shahjalal, M., Ahmed, S., Jang, Y. M. (2020). 6G wireless communication systems: Applications, requirements, technologies, challenges, and research directions. IEEE Open Journal of the Communications Society, 1, 957–975. [Google Scholar]
3. Roh, W., Seol, J. Y., Park, J., Lee, B., Lee, J. et al. (2014). Millimeter-wave beamforming as an enabling technology for 5G cellular communications: Theoretical feasibility and prototype results. IEEE Communications Magazine, 52(2), 106–113. [Google Scholar]
4. Rappaport, T. S., Xing, Y., MacCartney, G. R., Molisch, A. F., Mellios, E. et al. (2017). Overview of millimeter wave communications for fifth-generation (5G) wireless networks-with a focus on propagation models. IEEE Transactions on Antennas and Propagation, 65(12), 6213–6230. [Google Scholar]
5. Xiao, M., Mumtaz, S., Huang, Y., Dai, L., Li, Y. et al. (2017). Millimeter wave communications for future mobile networks. IEEE Journal on Selected Areas in Communications, 35(9), 1909–1935. [Google Scholar]
6. Zhu, Z., Huang, S., Chu, Z., Zhou, F., Zhang, D. et al. (2019). Robust designs of beamforming and power splitting for distributed antenna systems with wireless energy harvesting. IEEE Systems Journal, 13(1), 30–41. [Google Scholar]
7. Lu, X., Wang, P., Niyato, D., Kim, D. I., Han, Z. (2015). Wireless networks with RF energy harvesting: A contemporary survey. IEEE Communications Surveys & Tutorials, 17(2), 757–789. [Google Scholar]
8. Zhu, Z., Wang, N., Hao, W., Wang, Z., Lee, I. (2021). Robust beamforming designs in secure MIMO SWIPT IoT networks with a non-linear channel model. IEEE Internet Things Journal, 8(3), 1702–1715. [Google Scholar]
9. Bi, S., Ho, C. K., Zhang, R. (2015). Wireless powered communication: Opportunities and challenges. IEEE Communications Magazine, 53(4), 117–125. [Google Scholar]
10. Niu, H., Chu, Z., Zhou, F., Zhu, Z., Zhang, M. et al. (2021). Weighted sum secrecy rate maximization using intelligent reflecting surface. IEEE Transactions on Communications, 69(9), 6170–6184. [Google Scholar]
11. Bi, S., Zhang, R. (2016). Placement optimization of energy and information access points in wireless powered communication networks. IEEE Transactions on Wireless Communications, 15(3), 2351–2364. [Google Scholar]
12. Chu, Z., Zhou, F., Xiao, P., Zhu, Z., Mi, D. (2019). Resource allocation for secure wireless powered integrated multicast and unicast services with full duplex self-energy recycling. IEEE Transactions on Wireless Communications, 18(1), 620–636. [Google Scholar]
13. Niu, H., Chu, Z., Zhou, F., Zhu, Z., Zhen, L. et al. (2022). Robust design for intelligent reflecting surface assisted secrecy SWIPT network. IEEE Transactions on Wireless Communications, 21(6), 4133–4149. [Google Scholar]
14. Zhu, Z., Ma, M., Sun, G., Hao, W., Liu, P. et al. (2022). Secrecy rate optimizations in nonlinear energy harvesting model-based mmWave IoT systems with SWIPT. IEEE Systems Journal, 16(4), 5939–5949. [Google Scholar]
15. Niu, H., Chu, Z., Zhou, F., Zhu, Z. (2021). Simultaneous transmission and reflection reconfigurable intelligent surface assisted secrecy MISO networks. IEEE Communications Letters, 25(11), 3498–3502. [Google Scholar]
16. Zhu, Z., Xu, J., Sun, G., Hao, W., Chu, Z. et al. (2022). Robust beamforming design for IRS-aided secure SWIPT Terahertz systems with non-linear EH model. IEEE Wireless Communications Letters, 11(4), 746–750. [Google Scholar]
17. Liu, H., Li, G., Li, X., Huang, G., Liu, Y. et al. (2022). Effective capacity analysis of STAR-RIS assisted NOMA networks. IEEE Wireless Communications Letters, 11(9), 1930–1934. [Google Scholar]
18. Chu, Z., Zhu, Z., Zhou, F., Zhang, M., Al-Dhahir, N. (2021). Intelligent reflecting surface assisted wireless powered sensor networks for internet of things. IEEE Transactions on Communications, 69(7), 4877–4889. [Google Scholar]
19. Zheng, Y., Bi, S., Zhang, J., Quan, Z., Wang, H. (2020). Intelligent reflecting surface enhanced user cooperation in wireless powered communication networks. IEEE Wireless Communications Letters, 9(6), 901–905. [Google Scholar]
20. Chu, Z., Zhu, Z., Li, X., Zhou, F., Zhen, L. et al. (2022). Resource allocation for IRS assisted wireless powered FDMA IoT networks. IEEE Internet of Things Journal, 9(11), 8774–8785. [Google Scholar]
21. Li, G., Liu, H., Huang, G., Li, X., Raj, B. et al. (2021). Effective capacity analysis of reconfigurable intelligent surfaces aided NOMA network. EURASIP Journal on Wireless Communications and Networking, 198(1), 1–16. [Google Scholar]
22. Lyu, B., Ramezani, P., Hoang, D. T., Gong, S., Yang, Z. et al. (2021). Optimized energy and information relaying in self-sustainable IRS-empowered WPCN. IEEE Transactions on Communications, 69(1), 619–633. [Google Scholar]
23. Zhai, L., Zou, Y., Zhu, J., Guo, H. (2022). A stackelberg game approach for IRS-aided WPCN multicast systems. IEEE Transactions on Wireless Communications, 21(5), 3249–3262. [Google Scholar]
24. Ramezani, P., Jamalipour, A. (2021). Backscatter-assisted wireless powered communication networks empowered by intelligent reflecting surface. IEEE Transactions on Vehicular Technology, 70(11), 11908–11922. [Google Scholar]
25. Zheng, Y., Bi, S., Zhang, Y. J. A., Lin, X., Wang, H. (2021). Joint beamforming and power control for throughput maximization in IRS-assisted MISO WPCNs. IEEE Internet Things Journal, 8(10), 8399–8410. [Google Scholar]
26. Wu, Q., Zhou, X., Chen, W., Li, J., Zhang, X. (2022). IRS-aided WPCNs: A new optimization framework for dynamic IRS beamforming. IEEE Transactions on Wireless Communications, 21(7), 4725–4739. [Google Scholar]
27. Zeng, P., Wu, Q., Qiao, D. (2021). Energy minimization for IRS-aided WPCNs with non-linear energy harvesting model. IEEE Wireless Communications Letters, 10(11), 2592–2596. [Google Scholar]
28. Cao, H., Li, Z., Chen, W. (2021). Resource allocation for IRS-assisted wireless powered communication networks. IEEE Wireless Communications Letters, 10(11), 2450–2454. [Google Scholar]
29. Zhu, Z., Li, Z., Chu, Z., Sun, G., Hao, W. et al. (2022). Resource allocation for intelligent reflecting surface assisted wireless powered IoT systems with power splitting. IEEE Transactions on Wireless Communications, 21(5), 2987–2998. [Google Scholar]
30. Wang, Q., Gao, Z., Xu, Y., Xie, H. (2021). Energy-efficient optimization for IRS-assisted wireless-powered communication networks. IEEE 93rd Vehicular Technology Conference, Helsinki, Finland. [Google Scholar]
31. Zeng, P., Qiao, D., Wu, Q., Wu, Y. (2022). Throughput maximization for active intelligent reflecting surface-aided wireless powered communications. IEEE Wireless Communications Letters, 11(5), 992–996. [Google Scholar]
32. Lyu, B., Hoang, D. T., Gong, S., Yang, Z. (2020). Intelligent reflecting surface assisted wireless powered communication networks. IEEE Wireless Communications and Networking Conference Workshops (WCNCW), Seoul, Korea (South). [Google Scholar]
33. Cao, K., Ding, H., Li, W., Lv, L., Gao, M. et al. (2022). On the ergodic secrecy capacity of intelligent reflecting surface aided wireless powered communication systems. IEEE Wireless Communications Letters, 11(11), 2275–2279. [Google Scholar]
34. Chu, Z., Xiao, P., Mi, D., Hao, W., Khalily, M. et al. (2021). A novel transmission policy for intelligent reflecting surface assisted wireless powered sensor networks. IEEE Journal of Selected Topics in Signal Processing, 15(5), 1143–1158. [Google Scholar]
35. Zou, Y., Gong, S., Xu, J., Cheng, W., Hoang, D. T. et al. (2020). Wireless powered intelligent reflecting surfaces for enhancing wireless communications. IEEE Transactions on Vehicular Technology, 69(10), 12369–12373. [Google Scholar]
36. Chu, Z., Xiao, P., Mi, D., Chen, H., Hao, W. (2020). Intelligent reflecting surfaces enabled cognitive internet of things based on practical pathloss model. China Communications, 17(12), 1–16. [Google Scholar]
37. Wang, Z., Shi, Y., Zhou, Y., Zhou, H., Zhang, N. (2021). Wireless-powered over-the-air computation in intelligent reflecting surface-aided IoT networks. IEEE Internet of Things Journal, 8(3), 1585–1598. [Google Scholar]
38. Li, X., Xie, Z., Chu, Z., Menon, V. G., Mumtaz, S. et al. (2022). Exploiting benefits of IRS in wireless powered NOMA networks. IEEE Transactions on Green Communications and Networking, 6(1), 175–186. [Google Scholar]
39. Rusek, F., Persson, D., Lau, B. K. (2013). Scaling up MIMO: Opportunities and challenges with very large arrays. IEEE Signal Processing Magazine, 30(1), 40–60. [Google Scholar]
40. Boyd, S., Vandenberghe, L. (2004). Convex optimization. Cambridge, UK: Cambridge University Press. [Google Scholar]
41. Grant, M., Boyd, S. (2012). CVX: Matlab software for disciplined convex programming. http://cvxr.com/cvx/ [Google Scholar]
42. Zhang, Y., He, W., Li, X., Peng, H., Rabie, K. M. et al. (2022). Covert communication in downlink NOMA systems with channel uncertainty. IEEE Sensors Journal, 22(19), 19101–19112. [Google Scholar]
Cite This Article
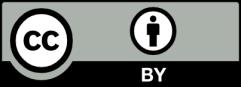