Open Access
REVIEW
Review of Recent Trends in the Hybridisation of Preprocessing-Based and Parameter Optimisation-Based Hybrid Models to Forecast Univariate Streamflow
1 Department of Civil Engineering, University of Maysan, Maysan, 57000, Iraq
2 Department of Civil Engineering, Wasit University, Wasit, 52001, Iraq
3 College of Engineering, University of Warith Al-Anbiyaa, Karbala, 56001, Iraq
4 Department of Civil Environmental and Natural Resources Engineering, Lulea University of Technology, Lulea, 971 87, Sweden
5 Department of Computer Science, Faculty of Computer Science and Information Technology, Universiti Putra Malaysia, Serdang, 43400, Malaysia
* Corresponding Author: Nadhir Al-Ansari. Email:
Computer Modeling in Engineering & Sciences 2024, 138(1), 1-41. https://doi.org/10.32604/cmes.2023.027954
Received 23 November 2022; Accepted 23 March 2023; Issue published 22 September 2023
Abstract
Forecasting river flow is crucial for optimal planning, management, and sustainability using freshwater resources. Many machine learning (ML) approaches have been enhanced to improve streamflow prediction. Hybrid techniques have been viewed as a viable method for enhancing the accuracy of univariate streamflow estimation when compared to standalone approaches. Current researchers have also emphasised using hybrid models to improve forecast accuracy. Accordingly, this paper conducts an updated literature review of applications of hybrid models in estimating streamflow over the last five years, summarising data preprocessing, univariate machine learning modelling strategy, advantages and disadvantages of standalone ML techniques, hybrid models, and performance metrics. This study focuses on two types of hybrid models: parameter optimisation-based hybrid models (OBH) and hybridisation of parameter optimisation-based and preprocessing-based hybrid models (HOPH). Overall, this research supports the idea that meta-heuristic approaches precisely improve ML techniques. It's also one of the first efforts to comprehensively examine the efficiency of various meta-heuristic approaches (classified into four primary classes) hybridised with ML techniques. This study revealed that previous research applied swarm, evolutionary, physics, and hybrid metaheuristics with 77%, 61%, 12%, and 12%, respectively. Finally, there is still room for improving OBH and HOPH models by examining different data pre-processing techniques and metaheuristic algorithms.Graphic Abstract
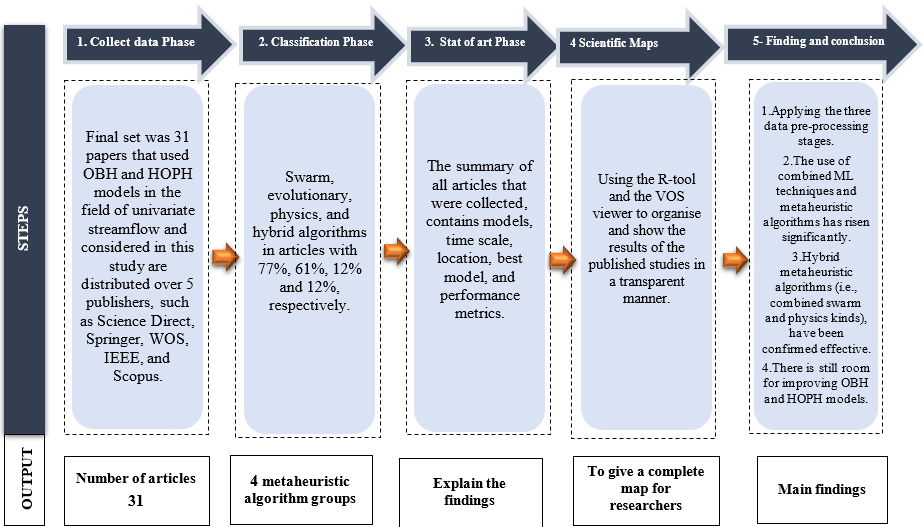
Keywords
Cite This Article
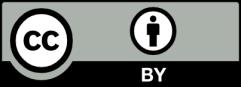
This work is licensed under a Creative Commons Attribution 4.0 International License , which permits unrestricted use, distribution, and reproduction in any medium, provided the original work is properly cited.