Open Access
REVIEW
Comprehensive Survey of the Landscape of Digital Twin Technologies and Their Diverse Applications
1 School of Computer, Jiangsu University of Science and Technology, Zhenjiang, 212003, China
2 Department of Electrical and Computer Engineering, University of Nevada Las Vegas, Las Vegas, NV, 89154, USA
3 Department of Electrical and Computer Engineering, National University of Singapore, 117583, Singapore
* Corresponding Author: Haijian Shao. Email:
Computer Modeling in Engineering & Sciences 2024, 138(1), 125-165. https://doi.org/10.32604/cmes.2023.027834
Received 17 November 2022; Accepted 16 May 2023; Issue published 22 September 2023
Abstract
The concept of the digital twin, also known colloquially as the DT, is a fundamental principle within Industry 4.0 framework. In recent years, the concept of digital siblings has generated considerable academic and practical interest. However, academia and industry have used a variety of interpretations, and the scientific literature lacks a unified and consistent definition of this term. The purpose of this study is to systematically examine the definitional landscape of the digital twin concept as outlined in scholarly literature, beginning with its origins in the aerospace domain and extending to its contemporary interpretations in the manufacturing industry. Notably, this investigation will focus on the research conducted on Industry 4.0 and smart manufacturing, elucidating the diverse applications of digital twins in fields including aerospace, intelligent manufacturing, intelligent transportation, and intelligent cities, among others.Keywords
Digital twin technology is a digital mirroring technology that extends information with 3D digital models throughout the lifecycle, ultimately synchronizing and aligning the virtual and physical worlds. In 2003, Michael Grieves of the University of Michigan [1] introduced the “mirror space model” (later renamed the “information mirror model”) in his product lifecycle management course [2]. According to the concept, the digital representation of a physical product should be able to depict it abstractly and provide testing of the physical product in real-world or simulated environments. This concept was published in 2011 in his book titled “Virtually Perfect: Driving Innovative and Lean Products through Product Lifecycle Management.” This conceptual paradigm was referred to as the “digital twin” by the paper’s co-authors [3]. The literature considers digital twins to be a mathematical model that can be constructed to characterize a physical device by accumulating its biological data. In addition, compare the differences between engineering design and model modification to gain a better understanding of the theoretical design and actual production, thereby enhancing the administratiorefern of the equipment’s entire lifecycle. The development roadmap for digital twins is shown in Fig. 1.
Figure 1: Digital twin development roadmap
With the maturation and industrialization of the Internet of Things and Big Data technologies in the 1910s, digital twins were ready to be applied in practice. NASA adopted in 2010 a flight system simulation analysis model based on the digital twin concept as outlined in the space technology roadmap [4] in order to accomplish comprehensive flight system diagnostics and predictable flight status. Thus, the safety of the entire mission is ensured within the system’s reliable timeframe. In 2010, NASA first defined the digital twin in the context of aerospace, elucidating on its strategic significance to American space science and the Air Force. NASA aims to have a fully operational, adaptable digital twin spacecraft by 2035 [5]. On the other hand, the U.S. Air Force has released several new research findings regarding the use of digital twins in fleet management, feasibility analysis, and flight diagnostics and prediction. Meanwhile, digital twin-driven smart manufacturing became a popular Industry 4.0 direction in 2010. The U.S. Air Force Research Laboratory introduced the digital duplicate in a presentation in March 2011 and began investigating it in the Condition-based Maintenance Plus (CBM+) project [6] that followed. In 2012, NASA published its “Modeling, Simulation, Information Technology, and Processing” road map, and the concept of the digital counterpart entered the public domain [7]. In 2013, the U.S. Air Force published the Global Horizon high-level planning document, which identified digital twin and digital thread as “game-changing” disruptive opportunities [8]; beginning in 2014, the Air Force organized a series of applied research projects for Boeing, General Electric, and other companies. The digital twin theory and technology system was established in this manner. Then, GE began to adapt this military technology for civilian use, employing the digital twin to provide asset management services and to construct an industrial Internet system. The definition of the digital counterpart has been refined and updated in recent years.
The digital twin lacks a universally recognized definition, and the concept is still developing and evolving. Since 2012, the following digital twin has emerged as defined in Table 1:
It is evident from the preceding definition that digital twin technology consists of the following elements:
(1) The relationship between the physical and digital worlds.
(2) Dynamic mapping.
(3) In addition to the physical mapping, logic, behavior, and process mapping, such as production processes, business processes, etc., must also be performed.
(4) The two-way mapping relationship between the physical world and the digital world, i.e., the digital world through calculation, processing, but also to provide instructions, computation.
(5) The entire life cycle, the digital twin used to create a virtual digital model, and the actual physical entity are synchronized to accomplish interactive feedback throughout the entire process.
In the context of “digitizing everything that can be digitized,” the author considers digital twins to be virtual objects generated in the digital virtual space via software definition and data drive. Regarding their shape, state, texture, behavior, and development laws, the items establish a real mapping relationship with their physical counterparts. It enables the physical duplicate to have a mapping relationship with the digital twin that has varying degrees of fidelity (realistic, abstract, etc.). In order to better comprehend the definition of digital twins, a brief introduction to the primary digital thread is provided. Digital strands and digital twins are both interrelated and distinct concepts. The digital twin is a digital representation of a physical product, allowing us to predict potential outcomes for the physical product based on the digital product. This includes technologies such as augmented reality and virtual reality. Digital twins permit the transfer of a complete 3D geometric model of the product definition to the digital production line for transformation into a physical product. During the design and production phases, the simulation analysis model’s parameters are reflected in the final product via an online digital inspection/measurement system, which feeds back into the simulation model. The United States Air Force and Lockheed Martin proposed the digital thread concept during the development of the F-35 [29]. The digital home run, according to them, is an enterprise-level analytical framework that expedites the controlled interaction of authoritative technical data, software, information, and knowledge. It is founded on the Digital System Model template to provide decision-makers with access to, integration of, and transformation of disparate data into actionable information throughout a system’s life cycle [30]. Digital Master Line technology enables unprecedented access to design and manufacturing data, thereby substantially enhancing the fighter jet manufacturing automation.
With the advent of the digital age, an increasing number of businesses are concentrating on the concept of digital threads. According to PTC, “A digital thread creates a closed loop between the digital and physical worlds in order to optimize products, people, processes, and places.”; iBASEt demonstrates that digital thread refers to the communication infrastructure that enables a connected data flow and an integrated view of the asset’s data across traditionally siloed functional perspectives throughout its lifecycle. The digital thread concept raises the threshold for delivering “the right information to the right place at the right time.” According to Cognizant, a digital thread is the flow of information about a product’s performance and use from design to production, sale, use, and disposal or recycling. This provides insights into how consumers utilize products, how those products perform, how those products could be improved, and what new features customers may desire.
Digital strands permeate the entire product lifecycle, particularly the integration between product design, production, operation, and maintenance. Digital twins resemble the intelligent product concept in that they emphasize feedback from product operation and maintenance to design. It is a digital shadow of the physical product, reflecting all of the object’s characteristics from microscopic to macroscopic through the integration of external sensors and illustrating the evolution of the product life cycle. Not only the product, but also the systems that produce the product, such as production apparatus, production lines, and systems in use and maintenance, should be constructed with digital twins on demand.
2 Digital Twin Development Status
This paper uses the WOS core collection database to conduct a statistical analysis of the literature on digital twin technology that has been compiled over the past five years. It generates a knowledge graph using the visual network analysis tool VOSviewer to illustrate recent research on digital twin technology. Included among the categories of literature retrieved are articles, conference papers, conference abstracts, and so on. Using the advanced search tool in WOS, the following search formula was constructed using field identifiers, Boolean operators, brackets, and search result sets: TS = (“digital twin” OR “digital twins”). ANDPY2018-2022, i.e., search for subjects (including titles, abstracts, and keywords) whose publication year falls between 2018 and 2022 and whose subject contains “digital twin” or “digital twins”.
Between 2018 and 2022, 425 documents that were publicly accessible in the WOS Core Collection database were obtained. In 2018, there were only nine publications, compared to twenty in 2019. In 2020, with 67 releases per year, the digital counterpart entered a period of gradual expansion. Fig. 2 indicates that as of November, 154 publications were published in 2021 and 173 in 2022. The number of publications in 2019 represents 40.0% of the previous five years, and the number of publications in 2020 represents 48.26% of the previous five years, indicating a rapid rate of development in the coming years.
Figure 2: Overall trends in digital twin technology development from 2018 to 2022
Fig. 3 shows the co-occurrence of the keywords used in the DT literature based on the WOS search results. The search results were optimized by implementing year constraints (2018–2022) and selecting only English-language journal articles. At least 200 of 1125 terms from a dataset of 425 previously published journal articles were utilized for this analysis. The figure depicts the digital twin-related topics that have emerged in recent years, such as Industry 4.0, big data, machine learning, 5G, 6G, and artificial intelligence.
Figure 3: Co-occurrence of keywords in the literature from 2018 to 2022
3 Digital Twins Related Technologies
3.1 Digital Twin Technology System
The digital twin is an innovative application that combines diverse technologies, including sensing, transmission, computing, modeling, and simulation [31]. As shown in Fig. 4, its architecture consists of a physical, data, model, and functional layer. The tangible layer refers to physical world objects. It is divided into tangible things (such as the human body, physical space, and objects) and intangible things (such as business procedures). The data layer includes data acquisition, transmission, and administration. The model layer is the central component of the digital twin application, employing modeling and other technologies to digitally replicate the representation of natural objects (digital twins). The functional layer is the direct value manifestation of the digital twin. It satisfies the requirements of each application scenario by encapsulating simulation, visualization, etc., results and providing them to business system applications.
Figure 4: Digital twin technology system
Data layer technologies include data acquisition and transmission, PLM-based data management, lifecycle data management, and others. The model construction technology is the core technology of the digital twin in the model layer, and its purpose is to construct a multidimensional model, i.e., the digital twin, using digital technology. According to the dimensions of the model layer, siblings can be classified as geometric, physical, or regular. Following is an overview of sophisticated modeling and simulation techniques, as well as multi-domain multi-scale modeling. The functional layer is then implemented utilizing various interaction and collaboration strategies.
3.2 Key Technologies for Digital Twins
3.2.1 Advanced Modeling and Simulation Technology
Advanced modeling and simulation refers to a multidisciplinary, coupled simulation of a product’s geometry, function, and performance. Connecting physical processes at distinct time scales in order to construct models that accurately represent the shape, behavior, and performance of physical entities such as atoms, molecules, and atoms. Research on advanced modeling and simulation technologies consists primarily of refined geometric modeling, logic modeling, finite element modeling, multi-physics field modeling, multi-disciplinary coupled modeling and simulation experiments, etc. Feature-based 3D modeling technology; SysML-based logic modeling technology; finite element-based multi-physics field coupling simulation technology; multi-disciplinary coupling performance simulation technology; database-based micro-kernel digital twin platform architecture, automatic model generation, and online simulation are the primary methods used to achieve high-fidelity simulation and real-time prediction of physical entities [32].
3.2.2 Multi-Domain and Multi-Scale Fusion Modeling
Under normal and abnormal operating conditions, multi-domain modeling refers to the cross-domain fusion modeling of physical systems from diverse domain perspectives. It combines models from various subject areas into a larger simulation model. Most contemporary modeling techniques conduct model development and maturation within a particular domain. Later, they use methods of integration and data fusion to combine independent models from diverse domains into a comprehensive system-level model [33]. However, this fusion approach lacks sufficient fusion depth and a reasonable explanation, limiting its capacity to profoundly fuse models from distinct domains. The complexity of multi-domain fusion modeling stems from the large degree of freedom in the system equations that results from the fusion of multiple properties. Simultaneously, the data collected by sensors must be highly consistent with the actual system data in order to assure dynamic updating of the model using high-precision sensing measurements. Numerous scientific problems can be simulated using multi-scale modeling, which connects physical processes at various time scales. Multi-scale models can represent fundamental. These computational models are more precise than simulation models with a single-dimensional scale that disregard multi-scale division [34]. The challenges of multi-scale modeling are reflected simultaneously in three dimensions: length, time scale, and coupling range; overcoming these challenges can aid in the development of more accurate digital twin systems.
3.2.3 Data Acquisition Technology Transmission
The acquisition and rapid transmission of data is the foundation of the entire digital twin system. To replicate the state of operation of the physical target system, the performance of each type of sensor, such as temperature, pressure, and vibration, should be optimal. The distribution of sensors and the construction of sensor networks must be rapid, secure, and precise, and distributed sensors must collect data on various physical quantities of the system to characterize its state [35]. Simultaneously, it is crucial to construct a rapid and dependable information transmission network for transmitting the system state information to the host computer in a secure and real-time manner for its application. The digital twin system is a dynamic, surreal, and real-time mapping of the physical entity system. Acquisition, transmission, and update of data in real time are essential for the digital duplicate. Many dispersed high-precision sensors of various types are at the vanguard of the entire twin system and play a crucial role in the twin system’s sensory capabilities. The current level of technological advancement restricts the type, precision, dependability, working environment, and data acquisition method of sensors. Real-time data transmission is crucial, but the current level of technology limits security, network transmission hardware, and network architecture to accommodate a higher transmission rate. In addition to the network security guarantee, the practical application must also take network security into account. Priority should be given to network security in practical applications. As sensor technology advances, an increasing number of microelectromechanical system sensors become more affordable and integrated. Application of high-bandwidth and low-cost wireless transmissions, such as the Internet of Things and other technologies, to enhance the characterization and evaluation of the operating state or abnormalities and malfunctions of the object system. The distance between the construction of cyber-physical systems and intelligent systems must be increased. Sensors are an integral component of the digital companion system and can be indispensable for comprehending how objects interact. A system or platform that integrates sensing, data acquisition, and data transmission at a low cost can be constructed using a variety of new sensing means or modules that are compatible with the existing object system.
3.2.4 PLM-Based Data Management
PLM-based data management refers to the formulation of a framework for integrating product information based on the architecture of the platform, so that all product-related data are highly coordinated, integrated, and shared. PLM-based data management technologies primarily consist of object-oriented incorporation and integration technologies with application software. Object modeling technologies that facilitate the modeling and management of product lifecycle data. Data warehouse administration systems facilitate data integration and decision-making [36].
3.2.5 Life-Cycle Data Management
The complex system’s entire life cycle data must be stored and managed in order to support the digital counterpart system. The use of cloud servers for the distributed administration of the system’s massive operational data, which enables high-speed data reading and redundant, secure backup, offers sufficient and trustworthy data sources for intelligent parsing algorithms [37]. By recording its entire life cycle, the system may be capable of historical state replication, structural health deterioration analysis, and intelligent analysis of any historical moment. This can also provide sufficient data for data analysis and presentation. The enormous quantity of historical data also provides abundant examples for data mining. By extracting the data’s practical characteristics and analyzing their correlations, it is possible to obtain a great deal of unknown but potentially valuable information. It is increasing knowledge and comprehension of the system’s mechanism and data characteristics, as well as realizing the fantastic properties of the digital counterpart. The implementation of long-term data storage and management necessitates redundant and distributed server-based storage. For the digital twin system, it is difficult to optimize the data distribution architecture, storage methods, and retrieval methods to achieve real-time and reliable data reading performance. Constructing a data center or data management system with a secure private cloud at its core is currently the more practical technical solution, particularly when considering the data security of industrial enterprises and information protection in the equipment industry.
3.2.6 Interaction and Collaboration Technology
Interaction and collaboration refer to the use of Virtual Reality (VR), Augmented Reality (AR), and Mixed Reality (MR), as well as other immersive experience human-computer interaction technologies, to achieve interaction and collaboration between the digital counterpart and the actual entity. VR technology can provide a hyper-realistic depiction of the system’s manufacturing, operation, and maintenance status. It can provide multi-domain and multi-scale monitoring and evaluation of each complex system’s off-weight subsystem. The superimposition of digital analysis yields a virtual mapping to the constructed twin system while precisely replicating the physical system [38]. VR technology enables users to comprehend and acquire diverse information regarding the target system’s principle, structure, characteristics, and change trends. The structure and status of a system’s various levels will be presented to the user via straightforward clicks and taps. This can be very essential for monitoring and guiding the production of complex equipment. The complexity of VR technology for complex systems resides in the arrangement of numerous high-precision sensors for system operation data collection. The virtual reality technology impediment must be eliminated and enhanced to provide a more realistic virtual reality system experience. These data presentation technologies can be utilized or borrowed by industrial data analysis to improve the efficacy and effectiveness of data analysis visualization.
3.2.7 Secure Interconnection Technology
Secure interconnection technology is the technology to secure and protect the integrity, validity, and confidentiality of digital twin models and data from tampering. Current research includes predicting and obtaining optimal defense strategies for possible attacks on digital twin models and data management systems, organizing and ensuring tamper-proof, traceable, and traceable twin data based on blockchain technology [38].
3.2.8 High-Performance Parallel Computing Technology
High-performance parallel computing refers to the optimization of the data structure and algorithm structure in order to increase the computing speed, transmission network real-time, and digital computing capabilities of the computing platform upon which the digital twin system operates. Real-time efficacy is a crucial performance indicator for digital twin systems. A distributed computing-based cloud server platform is an essential assurance. In order to ensure the system’s real-time performance, it is also essential to optimize the data structure and algorithm structure to enhance task execution speed [39]. An important aspect of its application to the digital twin is designing the optimal system computing architecture to meet the real-time analysis and computing requirements of the system, considering the computing performance of the system’s computing platform, the time delay of the data transmission network, and the computing capacity of the cloud computing platform. The level of digital computing capability of the platform directly influences the overall performance of the system, and its significance as the computing foundation of the entire system is undeniable. The real-time nature of the digital twin system necessitates a high level of computational performance, which is contingent upon the system’s computing platform and structure being optimized. Therefore, advancements in these two areas are required for the advancement of digital twin technology. The quantity of computer development and algorithm design optimization restricts the processing speed of the system. Cloud-based high-performance data analysis and high-performance computing in industrial real-time situations are two approaches that can be considered to meet the high-performance computing requirements.
The proliferation of artificial intelligence fuels the development of digital twin technology, while the rapid evolution and maturation of digital twin technology is stimulated by the proliferation of intelligent manufacturing and industrial intelligence. Current research in data generation, data analysis, and modeling considers the essential differences between big commercial data and big industrial data, such as abnormal or fault state simulation and injection, quantitative analysis of industrial data availability, enhanced deep learning with small or no samples, etc. The research focuses on data generation, data analysis, and modeling, as well as any associated difficulties. Methods and systems for semi-physical simulation, validation, and evaluation are also required for the development of digital twin platforms.
In 1970, NASA launched Apollo 13 and constructed an almost identical physical model of Apollo 13 on the ground [5], which replicated actual operating conditions to simulate behavior in real time. Before the spacecraft executes its mission, its digital duplicate simulates the spacecraft’s mission execution in a virtual simulation environment. In this manner, we are able to comprehend the state, behavior, mission success probability, operation parameters, and some issues that were not considered or estimated during the design phase of the aircraft in its actual use environment, thereby providing a foundation for subsequent task formulation, task parameter determination, and decision-making under abnormal conditions. By modifying the virtual environment’s parameter settings, the operation of the aircraft in various service environments can be simulated; by modifying the mission parameters, the impact of various mission parameters on the mission success rate, aircraft health and lifetime, etc., can be simulated. In addition, it can be used to simulate and validate the efficacy of various failure, degradation, and damage mitigation strategies designed to increase product health and longevity.
The physical model was tasked with reflecting the Apollo 13 spacecraft’s flight conditions as accurately as feasible during the mission. The siblings were then used to recreate various Earthly events in order to assist astronauts in making decisions in life-or-death situations. During NASA’s Apollo program, the twins were implemented in hardware rather than digital form [5]. This method was successful and flourished during the Apollo 13 space mission despite the exceedingly high cost of creating physical twins. After Apollo 13, numerous space programs utilized “physical twins” for decades. Physical twins are of limited utility to the profit-driven manufacturing and civil aviation industries due to the construction process and expense. However, early physical twins demonstrated the significance of the twin concept in managing, diagnosing, and predicting health. Additionally, it enabled the construction of digital twins in the future.
Emerging technology referred to as “digital twins” can provide high-fidelity, real-time virtual copies of aircraft counterparts. Twins capable of gathering, combining, storing, analyzing, and feeding data can be created, allowing for an ongoing evaluation of their corporeal entities. Due to the explosive growth of digital duplicates, the aerospace industry has shifted from reactive maintenance to proactive and predictive maintenance. This is done to improve the operational availability and efficacy of platforms, extend their lifespan, and reduce their lifecycle costs. Using multiphysics modeling and data-driven analytics, a new paradigm called the “digital twin” has been created. The DT is a dynamic representation of a physical system or asset that responds continuously to operational changes based on online data and information and can predict the future of its physical counterpart. The author examines the general framework for developing a digital twin in tandem with industrial IoT technologies to advance the autonomy of aerospace platforms and organizes and summarizes some of the DT’s detailed applications in aerospace.
Tuegel et al. [40–42] proposed a research framework for the use of digital twins as virtual sensors to predict the longevity and structural integrity of an aircraft structure. The research cited above [41,42] defined the airframe digital twin (ADT) as a computational model (specific model) of an individual aircraft. Through the development of customized structural management plans, this methodology can improve the way in which USAF aircraft are managed throughout their lifetimes. Using computational modeling, ADT can provide supervisory makes for each aircraft in the inventory and function as a virtual health sensor to predict future maintenance requirements for individual aircraft. Yang et al. [43] describe an automated image tracking method for obtaining data on fracture tip deformation and crack extension behavior of aluminum alloys and steel for digital twins of aircraft. The acquired data enables the digital twin model to predict the subperiodic fatigue fracture growth mechanisms of aviation materials throughout their lifecycle, thereby reducing production costs and implementation time [44]. Majumdar et al. [11] developed a DT model to predict how multi-physical environments (such as electric fields) cause changes in the microstructure of structural composites, which can affect structural performance. Bielefeldt et al. [45] proposed a digital twin approach for identifying fatigue fractures involving the use of an analytical model of an aircraft wing with embedded composite material alloy particulates in vital sections of the aircraft. To detect changes in structural risk, the authors simulate the stresses applied to the wing of an airplane during flight and then simulate the local particle reactivity in the critical zones. The work presented in [46] used the finite element alternative method (FEAM) to determine stress distribution [47] and a modified moving least squares (MLS) method to determine exhaustion breakage expansion percentages [48] to provide descriptive and predictive capabilities for the DT of an airplane. Thus, fatigue resistance mathematics can identify and predict damaged aircraft structures. Li et al. [49] proposed employing an improved dynamic Bayesian network model to govern the DT of aircraft wing state data. The research described in [50] combined the development of residual stresses modeling, firing on all cylinders analysis of thin-shell frameworks [51], and predictive maintenance [52] on the basis of related concepts to create a computational guidance foundation for residual stresses prediction of full-size laminated composite structures, which was successfully applied to wind turbine blades. Some of the most advanced aircraft DT analyze the response of guided waves to predict damage in real time [53]. Tuegel et al. [40] proposed a model that restructures the aircraft structural life prediction process and illustrates how to use digital twins to predict the life of aircraft structures and ensure their structural integrity by combining the estimation of structural deformation and temperature with the reaction to aircraft systems, the resulting local destruction, and temporal state evolution. After more than a decade of development and with the assistance of expert teams, the digital transformation of the aerospace industry has made remarkable progress in numerous areas. Many designers are now familiar with and increasingly employ digital simulation technology. The aerospace industry is progressively transitioning from traditional system engineering to model-based aerospace system engineering (MBASE) [54], with digital twin as the next frontier. The application of digital twin in the aerospace industry is depicted in Fig. 5.
Figure 5: Digital twin applications and technologies in the aerospace field
Based on multi-domain refinement modeling and simulation technology, construct a demand model, functional model, 3D structure model, circuit simulation analysis model, strength simulation analysis model, aerodynamic thermal simulation analysis model, flight performance simulation model, etc., to exhaustively describe all the characteristics and related information that should be considered during the product’s design phase. At the same time, combined with the same type of historical product data, key index parameters, design constraints, and other references, it adopts critical technologies such as multi-physics field coupled simulation, multi-disciplinary joint simulation, and data mining to carry out model-based collaborative design and virtual closed-loop verification, and the various types of refined digital simulation models resulting from this process are the foundation for constructing the digital twin.
The manufacturing process employs a refined design model derived from the 3D structure model. A production digital twin is created through the development of multiple process-oriented models, such as a process model, production line model, assembly model, material model, and CNC model, in conjunction with CPS data. For intelligent processing, detection, and modeling fusion, 3D scanning, multi-network fusion, process simulation, machine learning, and other virtual reality technologies are utilized. Building test part product models, test equipment models, test fixture models, test scene models, test environment models, and other test process-oriented models, as well as environmental monitoring data, test result data, work condition data, and test data collected based on CPS during the test process, distributed control, process modeling simulation, and other data model fusion, are the pillars of the testing process. During the flight test, digital twin technology is utilized to aid in the verification of reasoning and prediction. In the ground test, process optimization, fault detection, process monitoring, and intelligent evaluation are conducted. The training procedure is based on a refined, experiment-tested design model. It entails constructing training scene models, task flow models, product condition models, etc., adopting interaction and collaboration-key technologies such as VR/AR, developing virtual training mechanisms, and enhancing training plan formulation, training effect tracking, training reminder, and other functions. It also includes simulations of process planning.
The O&M process-oriented models, including product defect diagnosis and life prediction models, are built and combined with product operation data, condition monitoring data, and product quality data. In order to complete the work, machine learning and other data model fusion technologies, VR/AR, and other interaction and collaboration technologies are utilized. Due to the exceptionally long lifecycle of aircraft products, the aerospace industry has worked for decades to enhance product lifecycle management (more than 40 years, including development, production, and use). Despite this, the industry is still experiencing unprecedented stress due to production and service cycle costs, as well as difficulties with profitability in innovation, global competitiveness, and risk management. Contrary to other industries, new product development cycles for aerospace systems continue to expand as product complexity rises. In contrast, aircraft manufacturers and commercial airlines are sensitive to the uncertainties of the global economy and the multiple crises that effect them financially and technically (such as New Crown Pneumonia). This involves employing a prudent management strategy to ensure the overall efficacy of manufacturing processes and aircraft operations, such as optimizing maintenance, repair, and overhaul and enhancing aircraft reliability to reduce unplanned maintenance and increase the effectiveness and cost per flight of planned maintenance.
From product design to launch, including space science, security and defense, commercial aviation, and aerospace manufacturing, the DT is frequently employed in the aerospace industry. In the aerospace industry, research on digital twins encompasses all scales, from the individual component to the fleet. Aerospace manufacturing, operations, and maintenance (O&M) have been substantially enhanced by DT and related technologies. Engineers can use digital twin technology to detect potential problems with airframes, engines, and other components to ensure the safety of passengers.
In 2015, Professor Rios and his team proposed constructing digital twins for the manufacturing industry, overcoming the restriction that digital twins were only used in the aerospace industry [55]. According to Lee and his team, the use of digital twins in sophisticated manufacturing should not be limited to individual products. Employ big data analytics and cloud platforms on digital siblings as the foundation of “Industry 4.0” [56]. For instance, DT is the foundation of “Industry 4.0”, and extensive data analysis and cloud platforms are applied to DT [56]. Since then, research on the application of DT to manufacturing has persisted and attracted considerable domestic and international attention. DT is a crucial technology for businesses pursuing the strategic objective of smart manufacturing. As the manufacturing industry continues to move toward intelligence, this technology becomes a crucial link between traditional and intelligent manufacturing [57].
4.2.1 Application of Digital Twin in Product Manufacturing
The production mode of modern businesses is progressively shifting from mass production to small-batch, customized production. The comprehensive digitalization of manufactured products is a prerequisite for personalized manufacturing, which has emerged as a significant symbol of intelligent manufacturing. Establishing a digital twin model of a product can reduce the time required for design and production, meet the requirements of customer customization, and manage the entire life cycle of a product to increase the speed with which a business can respond to market demand. Designers who design too many products find it difficult to consider all aspects of product concepts, aesthetics, and the coordination of critical functions, as they must achieve outstanding and rapid client communication [58]. Tao et al. [59] proposed a digital twin-based product design model that incorporates concept design, detailed design, and virtual verification in order to make product design, manufacturing, and service more efficient, intelligent, and sustainable. Soderberg et al. [60] utilized a digital twin model of the product assembly process to rapidly transform products from mass production to individual manufacture. Um et al. [61] studied a CPS-based generic digital twin model. This model permits the transfer of product data through the product simulation, production, and manufacturing phases in order to achieve scalability and high modularity in the product assembly and manufacturing process. Schleich et al. [62] proposed an integrated digital twin reference model for physical products with essential model properties including scalability, interoperability, extensibility, and high fidelity, as well as the ability to combine, decompose, transform, and evaluate the model during the product design and production process. The product digital twin concept provides a real-time mapping of physical entities in virtual space. Through the Internet and the Internet of Things, it is possible to access real-time data on physical objects and a variety of information generated throughout the product’s life cycle [61]. This data and information can be utilized to enhance the design and functionality of the product.
In contrast, the digital twin model of the product can simulate the assembly process of complex products and optimize the design parameters of the product. Zhang et al. [63] proposed a five-dimensional digital twin modeling technique for manufacturing systems that implements the mapping between physical and virtual siblings. In addition, it proposes a framework based on an extensible model and deduces some digital twin dependencies and responsibilities. Using DT, Soderberg et al. [64] studied the geometric motion of product production. They created a DT system for guaranteeing product dimensions by utilizing a digital twin body for product coordinates and the machining trajectory. By simulating the product coordinates and machining trajectory with the digital twin, error analysis and trajectory optimization were performed, thereby improving the positioning and trajectory of the product and ensuring its machining quality.
4.2.2 Digital Twins in Manufacturing Equipment
The fundamental element of the production and processing procedure is manufacturing equipment. Intelligent, digital, and networked transformation of manufacturing equipment is required for the development of intelligent manufacturing. The equipment that digital twins can transform consists primarily of highly digitized equipment that can be wholly or partially automated. DebRoy et al. [65] investigated an additive manufacturing technology based on digital twins in order to partially reduce the number of defects and experiments. The digital twin model incorporates observable physical parameters such as microstructural changes in materials, heating and cooling rates, solidification parameters, residual stresses, and deformations into a numeric framework. Cai et al. [66] developed a digital twin model of a CNC machine tool that incorporates manufacturing and sensor data to enhance the physical machine tool’s reliability and machining capability. Scaglioni et al. [67] used the digital twin model of the MandelliM5 machine tool as a research site to investigate the adaptability of the machine tool’s structural components, cutting process model, drive chain model, and control system model using finite element analysis. Grieves [1] designed a web-based platform for machine tool condition monitoring. The mobile device is able to monitor the 3D model and real-time status of the machine. Simultaneously, augmented reality technology provides users with a visualization of the machine tool machining process in real time. Current research on digital twin-based manufacturing equipment centers on modifying existing automated equipment with digital twins to make production equipment more competitive than traditional automated equipment in terms of fault prediction and maintenance [68]. The majority of what is known about conventional manufacturing machinery comes from geometric and physical failure prediction models [69]. However, these methods rely too heavily on empirical data and have a low response rate to uncertain and episodic events. Through geometric, physical, behavioral, and rule-based modeling, digital twin-based equipment can evaluate the current condition of the equipment. Consequently, the development of manufacturing devices with digital twin capabilities is an excellent impetus for upgrading manufacturing industries to intelligent manufacturing.
The workshop is the basic unit of the manufacturing industry, and obtaining digitalization and intelligence in the workshop is essential for achieving intelligent manufacturing. Rapid progress has been made in real-time data collection, information system development, data integration, and virtual modeling and simulation as a result of the extensive use of information technology in workshops [70]. Building on this foundation, the interconnection and further integration of workshop information and physical space, as depicted in Fig. 6, will be the direction of workshop development and the only way to accomplish intelligent workshop production and control [71]. As a cornerstone of human functioning, physical workshops and their digital counterparts enable better resource management, increased productivity, and enhanced decision-making based on real-time data [72].
Figure 6: Digital twin in the smart workshop
Tao et al. [73,74] proposed the concept of Digital Twin Shop-Floor (DTS) for the interaction and integration of physical and virtual spaces in manufacturing workshops. They separated the digital twin shop into four parts: the physical shop, the virtual shop, the shop service system, and the shop twin data. Zhang et al. [75] proposed for insulating glass manufacturing lines a rapid, individualized design strategy based on digital twins. This method can distribute process data in real-time alongside the system model. Before production, the system can be designed digitally with authority. Small and medium-sized manufacturing companies rarely adopt fully automated data collection methods or systems due to the expense or system complexity. However, collecting data from the manufacturing process is essential for manufacturing companies to effectively adopt digital twins. The dearth of capability and development advantages of small and medium-sized enterprises (SMEs) in developing digital twins has not yet become apparent, resulting in a lack of motivation for SMEs to transform and upgrade the digital twin. Tuegel et al. [6] established a digital twin-based learning factory to meet the learning requirements of SMEs for a user-friendly, scalable, service-oriented control system based on the digital twin. Additionally, Graessler et al. [76] devised an employee-based digital twin production system in order to consider production employees as part of an integrated control system. In addition to including employee skills and experience, it also includes personalized attributes such as employee mood and demeanor, thereby improving the system’s transparency and comfort.
With the intensification of basic technology research, DT technology has attained an increasing number of research outcomes in intelligent manufacturing, demonstrating its significant technical advantages. Nevertheless, DT technology in the field of intelligent manufacturing is still in the experimental verification phase [77], primarily because the fundamental technologies have not yet been fully broken through, data collection and processing are insufficient, multidisciplinary integration is difficult, and DT technical standards are not yet mature. The discipline has not yet developed into a system. Nonetheless, with additional research on digital twins by related businesses and research institutions, a breakthrough in crucial DT technologies will be accomplished, and its application in intelligent manufacturing will expand.
Through research in the aircraft industry, the concept of digital twins was devised and found numerous applications. In the automotive industry, digital twins are primarily used to create fully or partially virtual vehicle models. To provide customer-specific support, the production process must have access to information on vehicle functionality and behavior while evaluating real-world performance. Before production begins, automobile manufacturers use technology to create the ideal automobile product [78]. They simulate and assess potential production and on-road issues. The digital twin can be presented as a model replica or a collection of interwoven components, as shown in Fig. 7 of the simulation. Digital twin technology is not limited to conventional automobile manufacturing, but can also benefit manufacturers of autonomous vehicles. Fig. 8 depicts the four levels where digital twin can be applied in smart driving applications: physical driving layer, twin data layer, twin driving layer, and twin application layer.
Figure 7: Digital twin vehicle simulation
Figure 8: Digital twin in the application of intelligent driving
The physical driving layer includes communication devices, sensing devices, data processing devices, and data storage devices. This layer’s primary responsibilities are to accomplish accurate data sensing and ensure data communication in real time. Digital marking technology is required to accomplish global and standardized digital marking of cars and roads for data acquisition and sensing. Edge computing at the end of the Internet of Things and the Internet of Things can be used to intelligently perceive the operational state data of high-dimensional, multi-node, large-scale complex systems. Data interaction and transmission require communication methods such as fiber optic communication, satellite communication, wireless communication, and 5G, among others.
The twin data layer pools and processes data resources obtained from the physical driving layer to establish a foundation for the twin driving layer. The data consists of vehicle data, environmental data (weather data, geographical data, etc.), and personnel data (various driver behavior data). Because driving data has characteristics such as dispersed sources, large data volumes, and varied structures, data middleware technology can also be applied to digital twin driving. A combination of edge computing and cloud computing can be utilized to avoid the superfluous data processing time caused by cloud computing, thereby guaranteeing the real-time nature and precision of twin driving. The twin driving layer precisely maps and intelligently supports the physical vehicle while simultaneously updating the twin driving model in real-time. This layer is the foundation of digital twin driving. Digital twin grid cannot rely on traditional experience-driven modeling approaches because it employs data-driven technology based on deep learning and data extraction rather than experience-driven modeling. Data model knowledge integration processing technology is required to create a generally intelligent system that combines multiple mechanism models with human knowledge and experience [79]. The twin application layer expands or improves the functional interfaces for physical car driving data analysis and visual interaction on the basis of the digital twin driving model. Big data technology and artificial intelligence technology are primarily responsible for data analysis in the twin application layer, whereas 3D visualization simulation technology meets the real-time nature of twin driving by simulating a driving scenario in multi-physical fields and full-scale, sacrificing partial accuracy for faster simulation technologies such as model downscaling technology.
The Chinese Ministry of Industry and Information Technology’s Digital Twin Application White Paper 2020 [80] identifies the establishment of digital mapping of entities as one of the pillars of constructing a digital twin system of virtual simulation platform included in the automated driving test system that combines virtual simulation with the natural environment. Wang et al. [81,82] utilized Vehicle-to-Cloud (V2C) communication to transmit sensor data to the virtual space via a server, calculate the corresponding model to obtain decision proposals, and provide the driver with real-time feedback. Using feature matching, Pan et al. [83] created a more realistic digital twin in the virtual scene by fusing images with texture features and continuous video segments obtained from the real world. Wu et al. [84] created a mixed reality framework for multi-view real-time monitoring of large-scale scenes by fusing 2D panoramas, satellite textures, and 3D models to produce 3D road scenes with digital duplicates. Li et al. [85] proposed a three-dimensional scene reconstruction method based on the binocular vision for research involving the construction of driving test scenarios for automated vehicles. This method can be used to reconstruct large-scale intelligent driving scenes in real-time, meeting the needs of intelligent driving systems. Tang et al. [86] investigated a method for integrating a simulator into a scenario involving autonomous driving. Simultaneously, the driver controls the background-driving vehicle via a driving simulator to simulate real-world driving behavior, thereby facilitating the simulation testing of autonomous driving vehicles. Song et al. [80] proposed a virtual joint simulation technology platform with a sensor module, a vehicle dynamics module, a scenario module, and a control algorithm module to address complex problems such as all-weather roads, sensor selection design, automatic control algorithm, and driving performance evaluation. This platform enhanced the system’s autonomous driving capability.
The advent of digital twin technology, the linchpin of Industry 4.0, has created unprecedented opportunities for the design and testing of intelligent automobiles. Theoretically, the digital twin provides unlimited reusability for testing under extreme conditions. It will result in safer and more effective vehicles and reduce the cost of complex physical test configurations. In addition, quantitative evaluation of vehicle performance parameters is a possibility for the future of continuous data processing. As artificial intelligence is further incorporated into the framework, it will be possible to evaluate the performance of current scenarios and accurately predict future electric vehicle performance metrics. Overall, the digital twin, as a universal technology for the interactive integration of the physical and informational worlds, is a crucial enabling technology for promoting the application of emerging manufacturing models such as smart manufacturing, industrial Internet, and the integration of two industries. Companies in the automotive industry and their suppliers should prioritize critical challenges and make greater efforts to investigate the application of digital twin technology in other fields. Digital twin technology can pave the way for resilient, cost-effective, safer, and adaptable smart electric vehicles by eliminating potential defects during the design phase.
The digital counterpart is also applicable to energy systems. In 2000, academician Lu Qiang proposed the idea of a digital power system [87]: “The physical structure, physical characteristics, technical performance, economic management, environmental indicators, personnel conditions, and scientific and educational activities of the actual operating power system are described and reproduced digitally, figuratively, and in real-time.” Digital power systems can be used “to improve the safety and stability of the system, to develop and implement economic operation strategies, to implement emergency control and anti-accident control of the power system, etc.”, according to the literature [88]. The digital power system proposed in the literature [89] is the power system’s digital counterpart. Recent studies have applied the digital twin’s technical framework to the online analysis of power systems [90].
The digital twin grid is intended to reflect the entire process of real grid operation, including equipment management, grid dispatching and operation, and electricity service applications in various scenarios. It consists of multidimensional, heterogeneous data from global sensing, historical accumulation, operation monitoring, and other sources. As depicted in Fig. 9, the digital duplicate grid is a complex system that coexists with the real grid and reflects the entire operation of the real grid.
Figure 9: Digital twin grid operation mode
The formation of a region-wide digital identity within the physical entity grid is necessary for accurate information matching, connection, and control between the digital twin grid and the physical entity grid. Data sources for intelligent analysis and decision-making are derived from the physical entity grid and transmission lines to the digital counterpart grid. Sensing and monitoring systems and data collection devices are utilized to precisely sense and collect the state information of the physical entity grid, such as equipment operation and personnel behavior, in real-time. The grid brain is a digital space-based data resource system, digital twin model, and machine intelligence platform. The data resource system provides the foundation for the digital twin grid. By constructing a data center, data is collected, collated, managed, and utilized in a unified manner to provide data for the construction of the digital twin model and the machine intelligence platform for intelligent operation and decision-making.
The basis of the digital twin power grid is the digital twin model with real-time mapping, which creates a multidimensional data space for the power grid. In digital space simulation, it employs a building information model (BIM) and engineering information modeling (EIM) to create a virtual-real mapping of equipment, subsystems, and the power grid. Simulation in digital space forms the apparatus, subsystem, and grid models of digital twins. Intelligent operation decisions are made by the intelligent analysis platform for the digital twin grid. We use artificial intelligence algorithms to analyze and simulate the digital twin model, provide real-time feedback to the digital twin model, optimize the model, and develop a self-optimizing intelligent operation model. This model is realized by constructing a platform for intelligent deep learning analysis that incorporates advanced technologies such as “big cloud, things, mobile, and smart chain.”
The digital twin grid includes “self-learning and self-optimizing” functions in its administration and operation. This function enables reverse control of physical grid equipment and associated topics by providing autonomous diagnostic and early warning, intelligent identification, condition assessment, planning, and decision-making of equipment and power grids (e.g., individuals). This strategy enables prompt problem-solving and the deployment of grid resources in a timely manner, resulting in an efficient grid operation. The essence of the optimal operation of the energy Internet, according to the literature [91], is “the process of fully mobilizing controllable resources of the system (source, network, and load) to achieve the goals of operating cost reduction and energy efficiency improvement based on the system operation law (tidal equation) and considering the operational constraints (adjustable and affordable range of components).” The digital twin of the energy Internet can, among other things, fully utilize the physical model of the network, online measurement data from advanced metering infrastructure, historical operation data from the network, and integrate multidisciplinary knowledge from electrical, fluid, thermal, computer, communication, climate, and economy to simulate various physical quantities, time scales, and probabilities. Integrating energy and digital technology will facilitate the development of a new intelligent energy system that is integrated and intelligent [92]. Digital twin technology in the energy sector is in its infancy as an advanced digital technology, but its application and development are accelerating. Numerous academics and specialists are researching this topic and achieving remarkable results.
Xu et al.’s [93] demonstration of the construction of digital twin models for power plant units illustrated how to accurately reproduce the thermal performance of power plant units operating under different conditions. The study demonstrates that low load operation in coal-fired power plants can reduce plant longevity and increase long-term operation and maintenance expenses. limited load operation can also lead to losses in thermal efficiency and limited capacity elements, which have a negative effect on the overall operating economy. Zhou et al. [94] proposed an online analytic digital twin (OADT) strategy to facilitate the implementation of a novel grid-based online analytic solution architecture. Hwang et al. [95] developed a micro-energy grid reference model by maximizing the use of various energy sources, including electricity, heat, and natural gas, to generate, utilize, and distribute energy efficiently. Yamazaki et al. [96] presented a strategy and data processing framework with analytic infrastructure to provide advantageous and innovative applications and services for future smart grids. Luo et al. [97] introduced a multi-intelligent body system (MAS) and blockchain-based two-layer energy transaction framework. A multi-agent system is designed to enable the prosumer network in the first layer. In the second stratum, a transaction settlement mechanism based on blockchain technology is proposed. This would allow for the secure and trustworthy settlement of electricity trading transactions. Using blockchain technology, numerous signatures, and anonymous cryptographic message flows, Aitzhan et al. [98] were developing an energy trading system that allows users to negotiate energy transactions securely and in secret. Customers could exchange energy-related transactions over a highly secure and encrypted network within an intelligent grid. Yang et al. [99] proposed an extreme learning machine (ELM)-based distributed virtual power plant (VPP) optimal scheduling strategy to maximize the role of DERs (distributed energy resources) within the grid.
Similarly, Abdolrasol et al. [100] developed a VPP optimal dispatch control model that uses the binary backtracking search algorithm (BBSA) to select the best unit to generate the optimal dispatch. The creators of the method created by Camal et al. [101] provided (DER) optimal scheduling for VPP and used a quantile regression forest model to predict the overall output. Dall’Anese et al. [102] utilized a primal-parity type strategy for instantaneous optimization of real-time VPP control. VPP can adjust DER output to preserve grid quality and meet customer and utility objectives. Pal et al. [103] also proposed the precise form of a residential user capable of energy trading between consumers and load-serving enterprises. Zhong et al. [104] proposed a DR model for a V2G mobile energy network in which electric vehicles transmit and store energy between network nodes. The model takes into account the stability of the DR algorithm for controlling the charging and discharging demand of electric vehicles. The experimental results demonstrate that the algorithm is resistant to mobility-induced coupling.
The digital twin technology for intelligent energy systems is utilized throughout energy production, transmission, storage, consumption, and trading, to summarize the preceding. It helps the energy sector overcome its time and space constraints by fostering comprehensive integration and unified dispatch management of multiple industries; It horizontally integrates the businesses of energy market participants and improves energy efficiency. The digital twin technological ecosystem of the smart energy sector (see Fig. 10) is segmented into six sections based on the entire lifetime of the energy system: energy production, energy transmission, energy distribution, energy consumption, energy storage, and the energy market. As the interaction between the various components intensifies, the sustainable growth of the smart energy sector facilitated by digital twin technology is progressively realized.
Figure 10: Digital twin technology ecosystem of the smart energy industry
Rather than a detailed numerical simulation of physical systems, conventional state sensing, straightforward AI, machine learning, or other data analysis, the digital twin is an organic combination of all three technologies. The digital twin digitally imitates the energy system and establishes an informational link between the digital and physical worlds; First, it uses complete information and precise mechanisms to predict the future, then it evolves to speculate the future based on incomplete information and uncertain mechanisms, and finally, it realizes the twin co-intelligent state of shared intelligence and co-evolution among the digital twins of the energy system [105].
As a digitally sophisticated technology, the application of digital twin technology in the energy sector is still in its infancy, but it is accelerating. Combining energy and digital technologies enables the creation of a new intelligently integrated energy system. The development of such a system has become a major trend in the energy sector. Digital twin technology can establish precise connections between the physical and digital worlds, thereby aiding in the resolution of technical obstacles impeding the development of smart energy and enabling accurate modeling and management of energy interconnection networks from multiple perspectives.
The primary application of DT in healthcare is the creation of virtual versions of physical entities or working processes based on real multidimensional and diverse data, such as digital representations of patient states, anatomical structures, or virtual environments resembling actual institutions. In layman’s terms, a precise model is constructed in the virtual world and then observed and analyzed for changes in the stimulus in response to circumstances, such as the feedback generated by the introduction of a new drug or a new treatment. In this procedure, data from electronic medical records, historical disease registry databases, daily behavior databases, and medical wearable devices are used to construct a model.
In the healthcare industry, the DT is equivalent to using digital means to replicate corporeal objects or services. It offers a safe environment for testing the efficacy of the involved systems. In terms of practical applications, it can provide doctors with information on surgical success rates, assist them in making judgments regarding treatment options, and manage chronic diseases in patients. The WuXi AppTec team has effectively developed a DT model using the machine learning platform and the Alzheimer’s disease model, paving the way for the application of DT technology in the healthcare sector.
As illustrated in Fig. 11, the construction of human digital twins has generated excitement in the medical and clinical fields, as it provides insight into what is occurring within the physical twin. The digital twin can predict the onset of illness by analyzing the personal history and current circumstances of the physical counterpart, such as location, time, and activity [106]. This will result in a shift from “one-size-fits-all” medical treatment to individualized treatment based on the individual’s “material assets,” which are determined by the individual’s structural, physical, biological, and historical characteristics. The virtual physiological person (VPH) [107] was introduced as a precursor to the human digital counterpart two decades ago. It is a comprehensive computer model designed to “collaboratively study the human body as a single complex system.” In order to evaluate the efficacy of treatment strategies, physicians and researchers can customize VPH for any patient. For example, the VPH could be utilized as a “virtual human laboratory” to facilitate electronic clinical trials or evaluations [108]. In 2015, French software developer Dassault Systèmes published The Living Heart [101]. The initial DT of the organ considers all aspects of organ function, including blood flow, mechanics, and electrical impulses. The software requires the entry of 2D images, which are then converted into a reliable 3D organ model. Cardiologists at the Heidelberg University Hospital (HUH) in Germany are actively testing and researching Siemens Healthineers’ [109] second cardiac DT. Siemens Healthineers utilizes a vast database containing over 250 million annotated images, reports, and operational data. The artificial intelligence-based DT model is trained to create 3D images from data on the heart’s electrical properties, physical characteristics, and structure.
Figure 11: Digital twin in healthcare
The Computational Biofluidics and Biomechanics Laboratory (CBBL) at Oklahoma State University created a digital duplicate of the human airway system [110–114]. The “Virtual Human V1” prototype was the first of its kind and featured a high-resolution virtual model of a 47-year-old male’s respiratory system, lung chambers, and body shell. By simulating this personalized digital twin using ANSYS computational fluid dynamics, as depicted in Fig. 12, researchers were able to conduct subject-specific health risk analyses for factors such as occupational silicon exposure. This includes evaluating the risk of lung deposition, epidermis absorption, and ventilation models in real time. The personalized treatment of intracranial aneurysms is a last example of digital organ duplication. Siemens assists neurological healthcare professionals in selecting the optimal implant size for aneurysms. It also allows physicians to create digital twins of aneurysms and arterial trees based on rotational angiography scans of individual patients. An aneurysm is a blood vessel protrusion caused by arterial wall weakness. A frighteningly small percentage of these aneurysms can cause blood clots, strokes, or mortality. Siemens developed the DT, which received regulatory approval, to aid surgeons in processing 3D rotational angiography images to construct a DT (representing a 3D model) of the aneurysm and adjacent blood arteries in order to select the appropriate implant. The customized DT enables simulations and provides the surgeon with insight into the interaction between the implant and the aneurysm.
Figure 12: Digital twin human respiratory simulation
In addition to these studies on digital twins of organs, researchers are examining the application of digital twins in healthcare. Using a UR5 robotic arm, Mathiassen et al. [115] created an ultrasound imaging system. In their application, the robot was used to isolate the physician from the ultrasound probe in order to prevent musculoskeletal disorders and discomfort. In [116], the system consists of a robotic limb connected via a 4G mobile network to an HTC VR system. Due to the lack of virtual reality system functionality and robotic input, the device cannot be used for remote surgery. Nonetheless, it can be used to research and perform remote surgery. Liu and colleagues [117] proposed a digital twin healthcare-based cloud-based healthcare system architecture. This is a novel, adaptable, and scalable cloud-based framework for monitoring, diagnosing, and predicting the health condition of individuals using wearable medical devices, with the goal of achieving personal health management, particularly for the elderly. Zhang et al. [118] presented MedMon, a medical safety monitor designed to identify problems with implanted medical devices. Ren et al. [119] proposed a Markov-based dynamic AD model that regulates the training data pattern using sliding windows. Home Automation Watcher (HAWatcher), a semantically aware ad hoc system [120] is used to implement intelligent dwellings. In [121], Smart Hospital recommends AD and intrusion detection systems to identify uncommon patient health-related events. Medicine has recently begun to investigate digital siblings. Future medical testing, scanning, and wearable technology may enable us to accurately recreate a digital body and trace the movement changes of each digital body component in order to improve health monitoring and management. Telemedicine will also become more prevalent as a result of transmission technologies like 5G. The first 5G-based remote human surgery for the implantation of a “brain pacemaker” for Parkinson’s disease has been completed. This has significant implications for the depletion of high-quality medical resources and the realization of automated diagnosis and treatment.
In a smart city, the DT functions as a bridge between the physical and virtual worlds. It transforms static city data into dynamic city data and maps the original two-dimensional city information system to three dimensions. Ideally, the DT of a smart city can simulate the system used to coordinate and administer the city as a whole. It centralizes city data for integrated city management while maintaining each municipal subsystem’s decentralized autonomy. Current urban subsystems employ highly developed digital duplicates. Siemens has a DT for water filtration at the largest desalination facility in the Middle East, for instance. BP has used digital twins to monitor and sustain its oil and gas facilities [122], among others [123], and [122] introduced digital twins for urban roads and traffic. In contrast, the entire city-level digital twin is still in the exploration phase; technical challenges include accumulating and processing heterogeneous urban data and considering operational costs, government support, transition plans, and other non-technical concerns. A few researchers have developed comprehensive conceptual concepts for city-level digital twins, including [124], who proposed a digital earth twin for Vienna [125] and [124], and [125], who created a digital twin for the West Cambridge campus.
The concept of a “digital twin city” was introduced for the first time in the Xiongan [126], a new area planning, digital map of the physical world. A visible, manageable, and controllable digital twin city is created using urban data such as people, cars, things, and spaces that encompass the entire area. Singapore introduced the “Singapore Smart” initiative in 2015. Singapore launched the “Singapore Smart Nation” initiative in 2015 and began to perceive itself as a smart nation [127]. The following year, Singapore collaborated with the Massachusetts Institute of Technology (MIT) in the United States to develop City Scope, a customized urban operations simulation system for Singapore’s urban planning. With an EU€8 million grant, Smart Santander in Spain has extensively deployed sensors in cities to sense the operation of the urban environment, traffic, water, etc., and aggregate the data in a smart city platform dashboard. The platform initially served as a prototype for a digital twin city and eventually became one of Europe’s replicable models.
The digital twin smart city architecture is intended to create a virtual representation of the physical city by integrating various urban physical entities and intelligent sensing devices in order to achieve sensing, monitoring, and the accumulation of urban data and information. This architecture is comprised of four layers: physical layer, interaction layer, middleware layer, and application layer [128], as shown in Fig. 13.
Figure 13: Digital twin smart city architecture
The physical layer is in charge of sensing, monitoring, and accumulating data from diverse physical entities and sensors. This layer serves as the basis for the complete architecture of the digital twin smart city. The interaction layer is responsible for transmitting collected data for further analysis and processing in a secure and efficient manner. It functions as a “bridge” between the physical space of the metropolis and the virtual space of the digital network.
Five sub-layers comprise the middleware layer: the resource layer, the virtualization layer, the object layer, the data layer, and the simulation layer. The resource layer offers automated configuration and deployment solutions for cloud resources, whereas the virtualization layer constructs the architecture design of distributed applications by decomposing them into independent, loosely coupled, and independently deployable services. To digitize the processes, elements, and life cycles of the city’s physical space, the object layer identifies objects, the classes into which they are categorized, and the relationships between objects. The data layer extracts data from data repositories, evaluates the condition of physical objects or systems to predict future failures and maintenance needs, and reports the results to smart city system administrators. The simulation layer enables the simulation of physical objects and visualization of the digital twin through simulation tools and design tools, as shown in Fig. 14.
Figure 14: Digital twin city simulation
The application layer provides a simulation environment that is identical to the physical space and provides city-centric planning and management, operation and control, defect diagnosis, and service operation capabilities. This stratum represents the experience and application phase of the digital twin technology and concept. Future-focused digital twin cities involve more than a simple mapping and replication of digital elements and the city’s physical area; they also involve the development and creation of the city under digital management.
The data twin is indispensable for secure and efficient perception calculation, decision feedback, and rapid and uniform data aggregation. Through the use of GIS and intelligent cloud computing fusion of Spatio-temporal correlation of large databases and “city hub”, it is possible to achieve comprehensive integration of physical and digital cities as well as collaborative field management. It is simpler for city managers to conduct urban simulation, early warning intervention of events, etc., and then to optimize urban planning, administration, and citizen services. Data is the most important strategic asset utilized by digital twin technology. As mobile data consumption increases, it becomes more difficult to provide adaptable, scalable, and flexible platforms that can address new challenges for little money and power. Ham et al. [129] proposed a novel architecture for integrating realistic information based on crowdsourced visual data into three-dimensional (3D) virtual cities for the dynamic, immersive presentation of model modifications. During Hurricane Harvey in 2017, Fan et al. [130] integrated textual and visual geography framework for social sensing technologies proved effective. Tianhu et al. [131] investigated the current state of digital twin technology and developed a digital twin model based on the development of smart cities. A platform for self-aware, self-determining, self-organizing, self-executing, and self-adaptive urban management and maintenance is constructed using mapping technology, IoT sensing, collaborative computing, simulation, and deep learning. The Digital Buildings UK Centre at the University of Cambridge is developing a road map for the digital twin initiative [132]. The road map outlines the necessary components and stakeholders for the successful implementation of a digital counterpart in the built environment.
Due to the complexity of cities, urban intelligence is a difficult process. Instead of being an automated system that is simple to comprehend and predict, the city is an evolving living system. Physical structures, economic and political activities, social and cultural environments, and ecosystems undergo daily changes and advancements. The concept of smart cities, which incorporates physical, social, and intellectual infrastructure, has supplanted the control of a city’s physical expansion. The coronavirus pandemic of 2019 has negatively impacted education, transportation, and amusement, making the development of smart cities and the digitization of services even more important. According to Kumar et al. [133], the four primary pillars of this revolutionary technology research and application are planning, physical infrastructure, information and communication technology (ICT) infrastructure, and smart solutions. The evolution of cities into smart cities hinges on these four factors. Urban digital siblings can support urban planning decisions and improve city management. Schrotter et al. [134] outlined how 3D spatial data and its models could enhance the current spatial data infrastructure. The 3D models consist of terrain, block, and roof models. Austin et al. [135] presented an intelligent architecture for urban digital twins that incorporates machine learning and semantic modeling. This architecture is complementary and supportive with respect to data acquisition and processing, event detection, and automated decision making. Dou et al. [136] developed a Spatio-temporal big data visualization platform to process, analyze, integrate, and display data from multiple sources in cities. The use of open-source software by Dembski et al. [137] enabled cities to make their data and software accessible to the public and experts, allowing them to participate in the evaluation and creation of the city’s digital counterpart. In addition, they created models of traffic and air pollution to assess their impact on urban planning [138]. Nochta et al. [139] utilized the urban-scale digital twin model, a recently developed urban study instrument, to demonstrate the evaluation of various electric vehicle charging station scenarios and their cross-sectoral repercussions.
Digital twin cities are a new technology path for building new smart cities, given the shift from quantitative to qualitative change of urban accumulation data and the significant advances in information technology, such as perception modeling and artificial intelligence. It is a cutting-edge advanced model of urban intelligence and sustainable operation; it is also a city-level innovation platform that attracts high-end intellectual resources to collaborate, from local applications to global optimization, and is continuously updated iteratively. Its fundamental value: Establish a new system based on closed-loop data integration and generate a global digital virtual image space for the city. Using a digital approach, virtualized interactions, modular assembly, and splicing to create a software-defined city, Real and virtual data-driven decisions entirely integrate the digital twin city body, allowing urban operation, management, and services to be modeled, simulated, evolved, and manipulated in the virtual space. On this basis, it can transform and promote the optimal allocation of urban resource elements in physical space from virtual to real, as well as introduce a new model for the construction and administration of new smart cities.
The DT city is a complex and integrated technology system that supports the construction of a new type of smart city, an advanced model for continuous innovation of intelligent urban operation, and a future development form of the city in which the physical city in the physical dimension and the virtual city in the information dimension coexist and blend with the real and the virtual. The development of DT began with perception and control technology and flourished with the innovation of integrated comprehensive technology. On the one hand, the DT city faithfully reflects the physical city’s condition. On the other hand, it precisely manipulates and intelligently optimizes the real city, which will drastically alter the appearance of the city, reconfigure the urban infrastructure, and create a new form of urban development through the interaction between the real and virtual worlds.
4.7 Other Application and Digital Twins Development Platforms
In addition to the applications mentioned previously, digital twins have the potential to be applied in agriculture, construction, environment, and security, such as real-time tracking of farm livestock and crop health diagnosis, quality assessment of housing construction, and optimal water and forest management. Digital twin technology enables IT managers to improve the efficacy of asset management and monitoring management, and to actualize new-generation data center operations and management that are three-dimensional and visualized. Adopting digital twin technology could, for instance, enable a virtual simulation of data centers and allow managers to intuitively comprehend adequate IT operations data. Digital twin technology enables IT managers to improve the efficacy of asset management and monitoring management, and to actualize new-generation data center operations and management that are three-dimensional and visualized. Adopting digital twin technology could, for instance, facilitate a virtual simulation of data centers and allow managers to intuitively comprehend effective information in IT operations.
Various applications of digital twins indicate extensive market potential. According to Grand View Research, the global market for digital twins will reach $7.48 billion in 2021 and is projected to expand at a CAGR of 39.1% between 2022 and 2030. OnePlan, a company founded in 2019, is a leader in digital twin technology for venues. The organization will develop data-driven “digital twins” for all main competition venues at the Paris Games. OnePlan’s digital counterpart and geoinformation software mapping tools generate digital venues that reflect a given venue’s area space and capacity, enabling staff to view venues from any angle, in any light or weather. The software allows personnel to view the venue from any angle, regardless of lighting or weather conditions. The software enables a variety of functions, including predicting how lighting and sound quality will affect the venue and allowing staff to determine where to position barricades, barriers, transportation, teams, and volunteers.
OnePlan is one of many companies to launch a digital twin for venues, as digital twin technology has been introduced to stadiums on multiple occasions in the past. A good example is the partnership between FC Barcelona and the Barcelona Supercomputing Center (BSC). In 2019, FC Barcelona announced a partnership with the BSC, one of the most significant supercomputing centers in Europe, to develop IoTwins. This novel system employs Internet of Things and artificial intelligence technologies. This initiative is also supported by the “Horizon 2020” (EU research and innovation funding) program. One objective of the IoTwins project is to create a digital twin that will initially function as a decision-making tool for the new stadium project of Barca Espai Barca. This significant stadium renewal initiative involves the expansion and renovation of Camp Nou as well as the construction of numerous new venues. It also accumulates anonymous data and creates computer simulations of the most common crowd movement patterns. The system can provide information to aid staff in making real-time decisions, arranging for smooth pedestrian movement, predicting foot traffic, organizing emergency equipment, and arranging venues spatially.
Obviously, the digital twin platform is applied to more than just the construction of venues; various platforms have varying application scenarios. Ansys Twin Builder’s three fundamental functions are model modeling, validation, and deployment. The fundamental research and application areas of the platform can be categorized into five categories: digital twin production equipment, digital twin in the design phase, digital twins in the operation and maintenance phase, digital twins based on empirical formulas, and digital twins based on simulation. The most recent version of Ansys Twin Builder facilitates inter- and multi-disciplinary system simulation and digital twin by integrating physical and virtual sensors in depth. It expedites the export and deployment of digital twins in order to actualize value-added services like predictive operation, maintenance, and performance optimization. AVEVA Unified Engineering, AVEVA Unified Operations Center, AVEVA Asset Performance Management, and AVEVA PI incorporate engineering, operations, and performance-related functions. AVEVA’s digital twin solutions span the entire lifetime, from design and construction to operations and maintenance. The digital twin solution from AVEVA can be utilized to create discrete digital twins, composite digital twins, and organizational digital twins. Digital twins can be created for individual assets, products, people, and process tasks, or they can be combined to form more complex collections of processes, functions, and assets.
ESI Group proposes the Hybrid Twin digital twin concept, which is a combination of virtual and digital, with the primary benefit being the creation of an additional, complementary virtual model that must be physically based and describe cause-and-effect relationships. ESI Group now offers the Hybrid Twin technology, which can combine physical models and real-time data to create digital duplicates. ESI Group’s Hybrid Twin technology has increased the range of electric vehicles by 40 percent while providing the highest level of passenger safety and comfort. Based on the Predix platform, GE Digital offers services that integrate Proficy, APM, OPM, iFIX, Historian, and other software services, as well as combine equipment mechanism models and data-driven analysis to create a digital counterpart. Specifically, the GE Proficy digital factory software family considers the stability and security of industrial applications while integrating the most advanced IT industry technologies, enabling users to construct and implement digital twins more effectively. GE overlays numerous extant asset and equipment data and models in order to provide a standard catalog of digital twin models via the Predix platform, including multiple industrial data analysis models and over 300 asset and process models. Using existing standard models for model construction, simulation, and training, users can rapidly create digital twins that can be executed in the field or in the cloud at scale. Users are able to submit models to the user side and then send the resulting data back to the cloud. GE Digital, Siemens, PTC, Ansys, Dassault Systèmes, AVEVA, Rockwell Automation, SAP, Altair, ESI Group, Microsoft, Maplesoft, Bentley, and Unity are among the major international providers of digital twin solutions. Some international vendors, including GE’s Predix, Siemens’ COMOS Platform, PTC’s ThingWorx, and Bentley’s iTwin Platform, have developed comprehensive platforms for developing digital twin applications using their respective enabling technologies. Other international vendors, including Dassault Systèmes with ABB, Ansys and Rockwell Automation, Altair and ACROME, and Microsoft and Ansys, have partnered with multiple vendors to offer the complete solution required for the digital counterpart.
In the age of 5G IoT, the digital companion is also a crucial scenario application. In 5G network scenarios, eMBB, mMTC, and URLLC are supported. Based on these three, many application scenarios and models are derived. eMBB can significantly increase network performance and improve network experience. To actualize the interconnection of all things, mMTC links more intelligent terminals. Due to its high reliability and low latency, URLLC is utilized in industries with stringent precision requirements, such as autonomous driving and mobile healthcare. Digital siblings derive from the aforementioned application scenarios. In digital twin production, industry, and cities, the combination of 5G and digital twin applications offers a vast array of integration points and development opportunities [140]. Consider the “European 5G Industrial Park” in Aachen, Germany, as an illustration of the consolidation and development trend in production and industry. Beginning in 2020, the Fraunhofer Institute for Production Technology IPT and the Swedish mobile network provider Ericsson developed the concept for the “European 5G Industrial Park”, the first comprehensive research network for 5G industrial applications. The park inaugurated its 5G network on May 12, 2020, and operates Europe’s largest 5G research network with an area of nearly one square kilometer, 19 5G antennas, and 10G bits per second of bandwidth. With the concurrent introduction of future-oriented digital technologies and manufacturing process equipment, such as digital twins and additive manufacturing, the park is establishing a globally unique industrial ecosystem. The facilities are outfitted with state-of-the-art IT and manufacturing systems, creating a one-of-a-kind infrastructure for researching and developing the next iteration of Industry 4.0 technologies based on 5G and incorporating digital twins and additive manufacturing.
Networked Adaptive Production is enabled by 5G networks, digital twins, and additive manufacturing, making the value chain for producing complex and mass-customized products more flexible and efficient than ever before. 5G brings the digital counterpart to vibrant life. With 5G high speed, augmented reality (AR) and virtual reality (VR), as well as other technologies, can be applied to the digital counterpart, and data has a superior presentation form and state. Real-time transmission of virtual and accurate data is enabled by 5G’s minimal latency, and the data is transmitted under improved conditions. Massive IoT dynamic data can be coupled with static data on the basis of an extensive connection, and the data has a richer heterogeneous variety as a result of better, quicker, and more diverse data. Beyond traditional industries, the digital counterpart has expanded to include construction, energy, healthcare, transportation, government management, parks, and cities.
The digital twin technology presents market opportunities for both the software and hardware industries. As the implementation of digital twin technology requires the incorporation of numerous new technologies and interdisciplinary knowledge, a complex and collaborative engineering system is required. Digital twin modeling technology has progressed from simple “component assembly” modeling of physical objects to multidimensional deep integration modeling of complex entities. As digital twin technology generates vast amounts of data, it poses significant data storage and computational challenges. To address this issue, advancements have been made in data storage and management systems, such as cloud computing and big data analytics, which facilitate the efficient administration and processing of vast quantities of data. The development of sensors and other monitoring devices, as well as the extension of the hardware industry, have contributed to the expansion of digital twin technology applications. In conclusion, the digital twin technology offers immense potential for both the software and hardware industries, but its successful implementation will require collaboration between diverse disciplines of expertise. Digital twin technology imposes substantial requirements on processing processors, data platforms, and devices. The digital twin model and data are extensive, comprising data and models of the fundamental elements, the entire business, and the process, which must be continuously updated throughout the modeling object’s lifetime. For real-time processing efficacy in model simulation and data analysis, this requires computer systems with massive processing capacity. On the basis of real-time model guidelines and data analysis results, feedback control methods to the physical space necessitate powerful computer equipment or hardware. Digital siblings have more interactive, immersive, and explicit requirements for terminal devices, necessitating devices with superior data transmission and display technology. Processing processors and data platforms become essential conditions for promoting the efficient, high-speed, and high-quality operation of the digital twin.
Digital twin technology refers to the construction of a virtual model or replica of a physical entity (such as a product, system, or environment) that is updated in real-time using data from sensors and other sources. The real-time synchronization of the virtual model and physical entity enables a two-way mapping between them, allowing for enhanced monitoring and control of the physical entity based on the virtual model’s insights. Connecting and transferring data between the virtual model and the corporeal entity is one of the fundamental components of digital twins. By obtaining complete synchronization between the two digital twins, technology can be used for higher-level purposes such as data analysis and product and device optimization. Advances in artificial intelligence, big data processing techniques, cloud computing, high-dimensional data coding, sensor technologies, and the Internet of Things have facilitated the development of digital twin technology. With the proliferation of DT technology, several data transfer technologies have emerged and been implemented in a variety of disciplines, including manufacturing, systems engineering, robotics, healthcare, and medicine. Overall, digital twin technology has the potential to benefit businesses and contribute to society.
We respond to two primary research questions: What is the currently accepted definition of DT? What are the application domains for DT systems? There is currently no universal definition of DT due to the general imprecision of the theoretical foundations of DT and the fact that DT is applied to a wide variety of disciplines and purposes. However, the paper identifies several applications of DT, including aerospace, intelligent manufacturing, intelligent transportation, energy, healthcare, and smart cities. We also observe that DT may be applicable in other areas, and future research will investigate new application trends and technological advancements in DT. The paper concludes by outlining a framework for investigating the various applications of DT and emphasizing the need for continued research and exploration in this area.
Acknowledgement: The authors wish to express their appreciation to the reviewers for their helpful suggestions which greatly improved the presentation of this paper.
Funding Statement: This research is supported by National Natural Science Foundation of China (No. 61902158).
Author Contributions: The authors confirm contribution to the paper as follows: study conception, design and data collection: Haiyu Chen, Haijian Shao; data collection, analysis and interpretation of results: Xing Deng; draft manuscript preparation: Lijuan Wang, Xia Wang. All authors reviewed the results and approved the final version of the manuscript.
Availability of Data and Materials: This paper is a review paper that primarily synthesizes existing methods and literature results. All data utilized in this study is derived from publicly available datasets. Readers can access these datasets through the sources cited in the references section of this paper. As the data originates from publicly accessible repositories, there are no restrictions on its availability. Therefore, all materials and data used in this review are readily accessible to interested readers.
Conflicts of Interest: The authors declare that they have no conflicts of interest to report regarding the present study.
References
1. Grieves, M. W. (2005). Product lifecycle management: The new paradigm for enterprises. International Journal of Product Development, 2(1–2), 71–84. [Google Scholar]
2. Grieves, M. W., Vickers, J. H. (2017). Digital twin: Mitigating unpredictable, undesirable emergent behavior in complex systems. In: Transdisciplinary perspectives on complex systems: New findings and approaches, pp. 85–113. Cham: Springer. [Google Scholar]
3. Grieves, M. W., Tanniru, M. R. (2008). PLM, process, practice and provenance: Knowledge provenance in support of business practices in product lifecycle management. International Journal of Product Lifecycle Management, 3(1), 37–53. [Google Scholar]
4. Piascik, B., Vickers, J. H., Lowry, D. W., Scotti, S., Stewart, J. et al. (2012). Materials, structures, mechanical systems, and manufacturing roadmap. National Aeronautics and Space Administration, Washington DC. [Google Scholar]
5. Rosen, R., Von Wichert, G., Lo, G., Bettenhausen, K. D. (2015). About the importance of autonomy and digital twins for the future of manufacturing. IFAC-PapersOnLine, 48(3), 567–572. [Google Scholar]
6. Tuegel, E. J., Ingraffea, A. R., Eason, T., Spottswood, S. M. (2011). Reengineering aircraft structural life prediction using a digital twin. International Journal of Aerospace Engineering, 2011(3), 1–14. [Google Scholar]
7. Glaessgen, E., Stargel, D. (2012). The digital twin paradigm for future NASA and US air force vehicles. 53rd AIAA/ASME/ASCE/AHS/ASC Structures, Structural Dynamics and Materials Conference, 20th AIAA/ASME/AHS Adaptive Structures Conference 14th AIAA, 1818. Honolulu, Hawaii, USA. [Google Scholar]
8. United States (2013). Global horizons: United States air force global science and technology vision, appendix. https://books.google.com/books/about/Global_Horizons.html?id=jIsdygEACAAJ [Google Scholar]
9. Tuegel, E. (2012). The airframe digital twin: Some challenges to realization. 53rd AIAA/ASME/ASCE/AHS/ASC Structures, Structural Dynamics and Materials Conference, 20th AIAA/ASME/AHS Adaptive Structures Conference 14th AIAA, 1812. Williamsburg, VA, USA. [Google Scholar]
10. Lee, J., Lapira, E., Bagheri, B., Kao, H. (2013). Recent advances and trends in predictive manufacturing systems in big data environment. Manufacturing Letters, 1(1), 38–41. [Google Scholar]
11. Majumdar, P. K., FaisalHaider, M., Reifsnider, K. (2013). Multi-physics response of structural composites and framework for modeling using material geometry. 54th AIAA/ASME/ASCE/AHS/ASC Structures, Structural Dynamics, and Materials Conference, 1577. Boston, Massachusetts, USA. [Google Scholar]
12. Grieves, M. (2014). Digital twin: Manufacturing excellence through virtual factory replication. White Paper, 1, 1–7. [Google Scholar]
13. Defense Acquisition University (2015). Digital system model. In: Glossary of defense acquisition acronyms and terms. Fort Belvoir: DAU Press. [Google Scholar]
14. Boschert, S., Rosen, R. (2016). Digital twin—the simulation aspect. In: Mechatronic futures: Challenges and solutions for mechatronic systems and their designers, pp. 59–74. Cham: Springer. [Google Scholar]
15. Schluse, M., Rossmann, J. (2016). From simulation to experimentable digital twins: Simulation-based development and operation of complex technical systems. 2016 IEEE International Symposium on Systems Engineering (ISSE), pp. 1–6. Edinburgh, Scotland, IEEE. [Google Scholar]
16. Negri, E., Fumagalli, L., Macchi, M. (2017). A review of the roles of digital twin in CPS-based production systems. Procedia Manufacturing, 11, 939–948. [Google Scholar]
17. Zheng, Y., Yang, S., Cheng, H. (2018). An application framework of digital twin and its case study. Journal of Ambient Intelligence and Humanized Computing, 10(3), 1141–1153. [Google Scholar]
18. Kritzinger, W., Karner, M., Traar, G., Henjes, J., Sihn, W. (2018). Digital twin in manufacturing: A categorical literature review and classification. IFAC-PapersOnLine, 51(11), 1016–1022. [Google Scholar]
19. Demkovich, N., Yablochnikov, E. I., Abaev, G. (2018). Multiscale modeling and simulation for industrial cyber-physical systems. 2018 IEEE Industrial Cyber-Physical Systems (ICPS), pp. 291–296. Saint Petersburg, Russia. [Google Scholar]
20. Renjen, P. (2018). Industry 4.0: Are you ready. Deloitte Review, 2018, 8–11. [Google Scholar]
21. Tao, F., Cheng, J., Qi, Q., Zhang, M., Zhang, H. et al. (2018). Digital twin-driven product design, manufacturing and service with big data. The International Journal of Advanced Manufacturing Technology, 94(9–12), 3563–3576. [Google Scholar]
22. Borth, M., Verriet, J., Muller, G. (2019). Digital twin strategies for SoS 4 challenges and 4 architecture setups for digital twins of SoS. 2019 14th Annual Conference System of Systems Engineering (SoSE), pp. 164–169. Anchorage, AK, USA. [Google Scholar]
23. Liu, Q., Liu, B., Wang, G., Zhang, C. (2019). A comparative study on digital twin models. AIP Conference Proceedings, vol. 2073, no. 1, 020091. Wuhan, China, AIP Publishing LLC. [Google Scholar]
24. Zhuang, C., Miao, T., Liu, J., Xiong, H. (2021). The connotation of digital twin, and the construction and application method of shop-floor digital twin. Robotics and Computer-Integrated Manufacturing, 68(3), 102075. [Google Scholar]
25. Fotland, G., Haskins, C., Rølvåg, T. (2020). Trade study to select best alternative for cable and pulley simulation for cranes on offshore vessels. Systems Engineering, 23(2), 177–188. [Google Scholar]
26. Liu, M., Fang, S., Dong, H., Xu, C. (2021). Review of digital twin about concepts, technologies, and industrial applications. Journal of Manufacturing Systems, 58, 346–361. [Google Scholar]
27. Peng, Y., Zhao, S., Wang, H. (2020). A digital twin based estimation method for health indicators of DC-DC converters. IEEE Transactions on Power Electronics, 36(2), 2105–2118. [Google Scholar]
28. Aheleroff, S., Xu, X. W., Zhong, R. Y., Lu, Y. (2021). Digital twin as a service (DTaaS) in Industry 4.0: An architecture reference model. Advanced Engineering Informatics, 47(2), 101225. [Google Scholar]
29. Kinard, D. A. (2018). F-35 production—advanced manufacturing and the digital thread. 2018 Aviation Technology, Integration, and Operations Conference, Atlanta, Georgia. [Google Scholar]
30. Leng, J., Liu, Q., Ye, S. F., Jing, J., Wang, Y. et al. (2020). Digital twin-driven rapid reconfiguration of the automated manufacturing system via an open architecture model. Robotics and Computer-Integrated Manufacturing, 63, 101895. [Google Scholar]
31. Rong, K., Ding, H., Kong, X., Huang, R., Tang, J. (2021). Digital twin modeling for loaded contact pattern-based grinding of spiral bevel gears. Advanced Engineering Informatics, 49(4), 101305. [Google Scholar]
32. Datong, L. (2018). Review and prospect of digital twin technology. Chinese Journal of Scientific Instrument, 39(11), 10 (in Chinese). [Google Scholar]
33. Hu, L., Nguyen, N., Tao, W., Leu, M. C., Liu, X. et al. (2018). Modeling of cloud-based digital twins for smart manufacturing with MT connect. Procedia Manufacturing, 26(2), 1193–1203. [Google Scholar]
34. Jiang, Y., Pjesivac-Grbovic, J., Cantrell, C., Freyer, J. P. (2005). A multiscale model for avascular tumor growth. Biophysical Journal, 89(6), 3884–3894. [Google Scholar] [PubMed]
35. Xiao, L., Boyd, S. P., Lall, S. (2005). A scheme for robust distributed sensor fusion based on average consensus. Fourth International Symposium on Information Processing in Sensor Networks, pp. 63–70. Los Angeles, California, USA. [Google Scholar]
36. Joyce, C., Michael, N., Chris, J., Ezra, K., Peter, A. (2013). Big data in life cycle assessment. Journal of Industrial Ecology, 17(6), 796–799. [Google Scholar]
37. Mandolla, C., Petruzzelli, A. M., Percoco, G., Urbinati, A. (2019). Building a digital twin for additive manufacturing through the exploitation of blockchain: A case analysis of the aircraft industry. Computers in Industry, 109(2), 134–152. [Google Scholar]
38. Bonnell, D. N., Tatarinov, K. L., Picard, M. W. (1997). System for monitoring and managing computer resources and applications across a distributed computing environment using an intelligent autonomous agent architecture. US, US05655081A. https://portal.unifiedpatents.com/patents/patent/US-5655081-A [Google Scholar]
39. Mohammadi, N., Taylor, J. E. (2017). Smart city digital twins. 2017 IEEE Symposium Series on Computational Intelligence (SSCI), pp. 1–5. Honolulu, Hawaii, USA. [Google Scholar]
40. Tuegel, E. J., Kobryn, P., Zweber, J. V., Kolonay, R. M. (2017). Digital thread and twin for systems engineering: Design to retirement. 55th AIAA Aerospace Sciences Meeting, 876. Grapevine, Texas, USA. [Google Scholar]
41. Tuegel, E. J., Bell, R. P., Berens, A. P., Brussat, T., Cardinal, J. W. et al. (2013). Aircraft structural reliability and risk analysis handbook volume 1: Basic analysis methods. Air Force Research Lab Wright-Patterson AFB OH Aerospace Systems DIR. [Google Scholar]
42. Tuegel, E. J. (2003). Investigation of the effect of corrosion pitting on fatigue life of aluminum structure. Air Force Research Lab Wright-Patterson AFB OH Analytical Structural Mechanics Branch. Wright-Patterson Air Force Base, OH, USA. [Google Scholar]
43. Yang, J., Zhang, W., Liu, Y. (2013). Subcycle fatigue crack growth mechanism investigation for aluminum alloys and steel. 54th AIAA/ASME/ASCE/AHS/ASC Structures, Structural Dynamics, and Materials Conference, 1499. Boston, Massachusetts, USA. [Google Scholar]
44. Kraft, E. M. (2016). The air force digital thread/digital twin-life cycle integration and use of computational and experimental knowledge. 54th AIAA Aerospace Sciences Meeting, 0897. San Diego, California, USA. [Google Scholar]
45. Bielefeldt, B., Hochhalter, J., Hartl, D. (2015). Computationally efficient analysis of SMA sensory particles embedded in complex aero-structures using a substructure approach. In: Smart materials, adaptive structures and intelligent systems, vol. 57298, V001T02A0071. Colorado Springs, Colorado, USA, American Society of Mechanical Engineers. [Google Scholar]
46. Wang, H. K., Haynes, R., Huang, H. Z., Dong, L., Atluri, S. N. (2015). The use of high-performance fatigue mechanics and the extended Kalman/particle filters, for diagnostics and prognostics of aircraft structures. Computer Modeling in Engineering & Sciences, 105(1), 1–24. https://doi.org/10.3970/cmes.2015.105.001 [Google Scholar] [CrossRef]
47. Liao, C. Y., Atluri, S. N. (1991). A finite element alternating method for evaluation of stress intensity factors FPR part-circular cracks subjected to arbitrary loadings. Computer Methods in Applied Mechanics and Engineering, 91(1–3), 1253–1270. [Google Scholar]
48. Dong, L., Haynes, R., Atluri, S. N. (2015). On improving the celebrated Paris’ power law for fatigue, by using moving least squares. Computers, Materials & Continua, 45(1), 1–16. https://doi.org/10.3970/cmc.2015.045.001 [Google Scholar] [CrossRef]
49. Li, C., Mahadevan, S., Ling, Y., Choze, S., Wang, L. (2017). Dynamic Bayesian network for aircraft wing health monitoring digital twin. AIAA Journal, 55(3), 930–941. [Google Scholar]
50. Bazilevs, Y., Deng, X., Korobenko, A., Scalea, F. L., Todd, M. D. et al. (2015). Isogeometric fatigue damage prediction in large-scale composite structures driven by dynamic sensor data. Journal of Applied Mechanics, 82(9), 091008. [Google Scholar]
51. Kiendl, J., Bletzinger, K., Linhard, J., Wüchner, R. (2009). Isogeometric shell analysis with Kirchhoff-Love elements. Computer Methods in Applied Mechanics and Engineering, 198(49–52), 3902–3914. [Google Scholar]
52. Farrar, C. R., Czarnecki, J. J., Sohn, H., Hemez, F. M. (2002). A review of structural health monitoring literature 1996–2001. The 3rd World Conference on Structural Control, Como, Italy. [Google Scholar]
53. Seshadri, B. R., Krishnamurthy, T. (2017). Structural health management of damaged aircraft structures using digital twin concept. 25th AIAA/AHS Adaptive Structures Conference, 1675. Grapevine, Texas, USA. [Google Scholar]
54. Latif, H. H., Starly, B. (2020). A simulation algorithm of a digital twin for manual assembly process. Procedia Manufacturing, 48(1), 932–939. [Google Scholar]
55. Ríos, J., Hernandez, J. C., Oliva, M., Mas, F. (2015). Product avatar as digital counterpart of a physical individual product: Literature review and implications in an aircraft. Transdisciplinary Lifecycle Analysis of Systems, 657–666. [Google Scholar]
56. Lee, J., Kao, H., Yang, S. (2014). Service innovation and smart analytics for Industry 4.0 and big data environment. Procedia CIRP, 16(4), 3–8. [Google Scholar]
57. Tao, F., Zhang, H., Liu, A., Nee, A. Y. (2019). Digital twin in industry: State-of-the-art. IEEE Transactions on Industrial Informatics, 15(4), 2405–2415. [Google Scholar]
58. Ezhilarasu, C. M., Skaf, Z., Jennions, I. K. (2019). Understanding the role of a digital twin in integrated vehicle health management (IVHM). 2019 IEEE International Conference on Systems, Man and Cybernetics (SMC), pp. 1484–1491. Bari, Italy, IEEE. [Google Scholar]
59. Tao, F., Sui, F., Liu, A., Qi, Q., Zhang, M. et al. (2019). Digital twin-driven product design framework. International Journal of Production Research, 57(12), 3935–3953. [Google Scholar]
60. Söderberg, R., Wärmefjord, K., Madrid, J., Lorin, S., Forslund, A. et al. (2018). An information and simulation framework for increased quality in welded components. CIRP Annals-Manufacturing Technology, 67(1), 165–168. [Google Scholar]
61. Um, J., Weyer, S., Quint, F. (2017). Plug-and-simulate within modular assembly line enabled by digital twins and the use of automationML. IFAC-PapersOnLine, 50(1), 15904–15909. [Google Scholar]
62. Schleich, B. R., Anwer, N., Mathieu, L., Wartzack, S. (2017). Shaping the digital twin for design and production engineering. CIRP Annals-Manufacturing Technology, 66(1), 141–144. [Google Scholar]
63. Zhang, C., Xu, W., Liu, J., Liu, Z., Zhou, Z. et al. (2019). A reconfigurable modeling approach for digital twin-based manufacturing system. Procedia CIRP, 83, 118–125. [Google Scholar]
64. Söderberg, R., Wärmefjord, K., Carlson, J. S., Lindkvist, L. (2017). Toward a digital twin for real-time geometry assurance in individualized production. CIRP Annals-Manufacturing Technology, 66(1), 137–140. [Google Scholar]
65. DebRoy, T., Zhang, W., Turner, J. A., Babu, S. S. (2017). Building digital twins of 3D printing machines. Scripta Materialia, 135, 119–124. [Google Scholar]
66. Cai, Y., Starly, B., Cohen, P. H., Lee, Y. (2017). Sensor data and information fusion to construct digital-twins virtual machine tools for cyber-physical manufacturing. Procedia Manufacturing, 10(3), 1031–1042. [Google Scholar]
67. Scaglioni, B., Ferretti, G. (2018). Towards digital twins through object-oriented modelling: A machine tool case study. IFAC-PapersOnLine, 51(2), 613–618. [Google Scholar]
68. Rosen, R., Boschert, S., Sohr, A. (2018). Next generation digital twin: Öko-system für simulations basierte Lösungen im Betrieb. ATP Magazin, 60(10), 86–96. [Google Scholar]
69. Botkina, D., Hedlind, M., Olsson, B., Henser, J., Lundholm, T. (2018). Digital twin of a cutting tool. Procedia CIRP, 72(3), 215–218. [Google Scholar]
70. Tao, F., Zhang, M., Liu, Y., Nee, A. Y. (2018). Digital twin driven prognostics and health management for complex equipment. CIRP Annals-Manufacturing Technology, 67(1), 169–172. [Google Scholar]
71. Li, W., Pan, C. W., Zhang, R., Ren, J. P., Ma, Y. X. et al. (2019). AADS: Augmented autonomous driving simulation using data-driven algorithms. Science Robotics, 4(28), eaaw0863. [Google Scholar] [PubMed]
72. Shangguan, D., Chen, L., Su, C., Ding, J., Liu, C. (2022). A triple human-digital twin architecture for cyber-physical systems. Computer Modeling in Engineering & Sciences, 131(3), 1557–1578. https://doi.org/10.32604/cmes.2022.018979 [Google Scholar] [CrossRef]
73. Tao, F., Zhang, M. (2017). Digital twin shop-floor: A new shop-floor paradigm towards smart manufacturing. IEEE Access, 5, 20418–20427. [Google Scholar]
74. Luo, W., Hu, T., Zhang, C., Wei, Y. (2018). Digital twin for CNC machine tool: Modeling and using strategy. Journal of Ambient Intelligence and Humanized Computing, 10(3), 1129–1140. [Google Scholar]
75. Zhang, H., Liu, Q., Chen, X., Zhang, D., Leng, J. (2017). A digital twin-based approach for designing and multi-objective optimization of hollow glass production line. IEEE Access, 5, 26901–26911. [Google Scholar]
76. Graessler, I., Poehler, A. (2018). Intelligent control of an assembly station by integration of a digital twin for employees into the decentralized control system. Procedia Manufacturing, 24(2), 185–189. [Google Scholar]
77. Magargle, R., Johnson, L., Mandloi, P., Davoudabadi, P., Kesarkar, O. et al. (2017). A simulation-based digital twin for model-driven health monitoring and predictive maintenance of an automotive braking system. Proceedings of the 12th International Modelica Conference, no. 132, pp. 35–46. Prague, Czech Republic: Linköping University Electronic Press. [Google Scholar]
78. Luo, W., Hu, T., Ye, Y., Zhang, C., Wei, Y. (2020). A hybrid predictive maintenance approach for CNC machine tool driven by digital twin. Robotics and Computer-Integrated Manufacturing, 65(1), 101974. [Google Scholar]
79. Liu, R., Li, H., Lv, Z. (2023). Modeling methods of 3D model in digital twins. Computer Modeling in Engineering & Sciences, 136(2), 985–1022. https://doi.org/10.32604/cmes.2023.023154 [Google Scholar] [CrossRef]
80. Song, J., Huang, R., Zhang, W., Liu, Q. (2020). Automatic driving joint simulation technology and platform design. Proceedings of China SAE Congress 2018: Selected Papers, pp. 393–404. Singapore, Springer. [Google Scholar]
81. Wang, Z., Liao, X., Zhao, X., Han, K., Tiwari, P. et al. (2020). A digital twin paradigm: Vehicle-to-cloud based advanced driver assistance systems. 2020 IEEE 91st Vehicular Technology Conference (VTC2020-Spring), pp. 1–6. IEEE. [Google Scholar]
82. Wang, Z., Han, K., Tiwari, P. (2020). Augmented reality-based advanced driver-assistance system for connected vehicles. 2020 IEEE International Conference on Systems, Man, and Cybernetics (SMC), pp. 752–759. Toronto, Ontario, Canada. [Google Scholar]
83. Pan, C., Chen, Y., Wang, G. (2016). Virtual-real fusion with dynamic scene from videos. 2016 International Conference on Cyberworlds (CW), pp. 65–72. Chongqing, China, IEEE. [Google Scholar]
84. Wu, Y., Liu, C., Lan, S., Yang, M. (2014). Real-time 3D road scene based on virtual-real fusion method. IEEE Sensors Journal, 15(2), 750–756. [Google Scholar]
85. Li, Y. L., Zhou, Z. K., Bai, L. (2019). Reconstruction of large-scale intelligent driving scene based on binocular image. Computer Science, 46(S2), 251–254 (in Chinese). [Google Scholar]
86. Tang, H., Wang, L., Li, Z. (2020). Application of driving simulator in automatic driving system. AutoMotive Abstracts, 1, 28–31 (in Chinese). [Google Scholar]
87. Qiang, L. (2000). Digital power system. Automation of Electric Power Systems, 24, 1–4 (in Chinese). [Google Scholar]
88. Alam, K. M., El Saddik, A. (2017). C2PS: A digital twin architecture reference model for the cloud-based cyber-physical systems. IEEE Access, 5, 2050–2062. [Google Scholar]
89. Damjanovic-Behrendt, V. (2018). A digital twin-based privacy enhancement mechanism for the automotive industry. 2018 International Conference on Intelligent Systems (IS), pp. 272–279. Funchal, Madeira Island, IEEE. [Google Scholar]
90. Tao, F., Zhang, M., Cheng, J., Qi, Q. (2017). Digital twin workshop: A new paradigm for future workshop. Computer Integrated Manufacturing Systems, 23(1), 1–9. [Google Scholar]
91. Shao, C., Wang, X., Wang, X., Wang, B. (2016). Probe into analysis and planning of multi-energy systems. Proceedings of the CSEE, 36(14), 3817–3828. [Google Scholar]
92. Tao, F., Zhang, M., Nee, A. Y. (2019). Five-dimension digital twin modeling and its key technologies. Digital Twin Driven Smart Manufacturing (in Chinese). [Google Scholar]
93. Xu, B., Wang, J., Wang, X., Liang, Z., Cui, L. et al. (2019). A case study of digital-twin-modelling analysis on power-plant-performance optimizations. Clean Energy, 3(3), 227–234. [Google Scholar]
94. Zhou, M., Yan, J., Feng, D. (2019). Digital twin framework and its application to power grid online analysis. CSEE Journal of Power and Energy Systems, 5(3), 391–398. [Google Scholar]
95. Hwang, T., Choi, M., Kang, S., Lee, I. (2012). Design of application-level reference models for micro energy grid in IT perspective. 2012 8th International Conference on Computing and Networking Technology (INC, ICCIS and ICMIC), pp. 180–183. IEEE. [Google Scholar]
96. Yamzaki, J., Fukuhara, H., Itoh, Y., Mursawa, M., Tsai, J. et al. (2014). Data processing framework with analytic infrastructure for future smart grid. 2014 International Conference on Renewable Energy Research and Application (ICRERA), pp. 241–244. Milwaukee, USA, IEEE. [Google Scholar]
97. Luo, F., Dong, Z. Y., Liang, G., Murata, J., Xu, Z. (2018). A distributed electricity trading system in active distribution networks based on multi-agent coalition and blockchain. IEEE Transactions on Power Systems, 34(5), 4097–4108. [Google Scholar]
98. Aitzhan, N. Z., Svetinovic, D. (2018). Security and privacy in decentralized energy trading through multi-signatures, blockchain and anonymous messaging streams. IEEE Transactions on Dependable and Secure Computing, 15(5), 840–852. [Google Scholar]
99. Yang, H., Yi, D. X., Zhao, J., Luo, F., Dong, Z. Y. (2014). Distributed optimal dispatch of virtual power plant based on ELM transformation. Journal of Industrial and Management Optimization, 10(4), 1297–1318. [Google Scholar]
100. Abdolrasol, M. G., Hannan, M. A., Mohamed, A., Amiruldin, U. A., Abidin, I. Z. et al. (2018). An optimal scheduling controller for virtual power plant and microgrid integration using the binary backtracking search algorithm. IEEE Transactions on Industry Applications, 54(3), 2834–2844. [Google Scholar]
101. Camal, S., Michiorri, A., Kariniotakis, G. (2018). Optimal offer of automatic frequency restoration reserve from a combined PV/Wind virtual power plant. IEEE Transactions on Power Systems, 33(6), 6155–6170. [Google Scholar]
102. Dall’Anese, E., Guggilam, S. S., Simonetto, A., Chen, Y. C., Dhople, S. V. (2018). Optimal regulation of virtual power plants. IEEE Transactions on Power Systems, 33(2), 1868–1881. [Google Scholar]
103. Pal, S., Kumar, R. (2018). Electric vehicle scheduling strategy in residential demand response programs with neighbor connection. IEEE Transactions on Industrial Informatics, 14(3), 980–988. [Google Scholar]
104. Zhong, W., Yu, R., Xie, S., Zhang, Y., Yau, D. K. (2018). On stability and robustness of demand response in V2G mobile energy networks. IEEE Transactions on Smart Grid, 9(4), 3203–3212. [Google Scholar]
105. Laboratory of Digital Twins, Pera Global Technology Co., Ltd. (2019). White paper on digital twin technology. Beijing, China: Laboratory of Digital Twins, Pera Global Technology Co., Ltd. [Google Scholar]
106. Bruynseels, K., Santoni de Sio, F., van den Hoven, J. (2018). Digital twins in health care: Ethical implications of an emerging engineering paradigm. Frontiers in Genetics, 9, 31. [Google Scholar] [PubMed]
107. Viceconti, M., de Vos, M., Mellone, S., Geris, L. (2023). From the digital twins in healthcare to the virtual human twin: A moon-shot project for digital health research. arXiv preprint arXiv:2304.06678. [Google Scholar]
108. Viceconti, M., Henney, A. M., Morley-Fletcher, E. (2016). In silico clinical trials: How computer simulation will transform the biomedical industry. International Journal of Clinical Trials, 3(2), 37–46. [Google Scholar]
109. Kamel Boulos, M. N., Zhang, P. (2021). Digital twins: From personalised medicine to precision public health. Journal of Personalized Medicine, 11(8), 745. [Google Scholar] [PubMed]
110. Chen, X., Feng, Y., Zhong, W., Kleinstreuer, C. (2017). Numerical investigation of the interaction, transport and deposition of multicomponent droplets in a simple mouth-throat model. Journal of Aerosol Science, 105, 108–127. [Google Scholar]
111. Feng, Y., Zhao, J., Chen, X., Lin, J. (2017). An in silico subject-variability study of upper airway morphological influence on the airflow regime in a tracheobronchial tree. Bioengineering, 4(4), 90. [Google Scholar] [PubMed]
112. Chen, X., Feng, Y., Zhong, W., Sun, B., Tao, F. (2018). Numerical investigation of particle deposition in a triple bifurcation airway due to gravitational sedimentation and inertial impaction. Powder Technology, 323, 284–293. [Google Scholar]
113. Feng, Y., Zhao, J., Kleinstreuer, C., Wang, Q., Wang, J. et al. (2018). An in silico inter-subject variability study of extra-thoracic morphology effects on inhaled particle transport and deposition. Journal of Aerosol Science, 123(8), 185–207. [Google Scholar]
114. Tan, H. Y., Wang, N., Lam, W., Guo, W., Feng, Y. et al. (2018). Targeting tumour microenvironment by tyrosine kinase inhibitor. Molecular Cancer, 17(1), 1–15. [Google Scholar]
115. Mathiassen, K., Fjellin, J. E., Glette, K., Hol, P. K., Elle, O. J. (2016). An ultrasound robotic system using the commercial robot UR5. Frontiers in Robotics AI, 3, 1. [Google Scholar]
116. Liu, Y., Zhang, L., Yang, Y., Zhou, L., Ren, L. et al. (2019). A novel cloud-based framework for the elderly healthcare services using digital twin. IEEE Access, 7, 49088–49101. [Google Scholar]
117. Laaki, H., Miché, Y., Tammi, K. (2019). Prototyping a digital twin for real time remote control over mobile networks: Application of remote surgery. IEEE Access, 7, 20325–20336. [Google Scholar]
118. Zhang, M., Raghunathan, A., Jha, N. K. (2013). MedMon: Securing medical devices through wireless monitoring and anomaly detection. IEEE Transactions on Biomedical Circuits and Systems, 7(6), 871–881. [Google Scholar] [PubMed]
119. Ren, H., Ye, Z., Li, Z. (2017). Anomaly detection based on a dynamic Markov model. Information Sciences, 411(2), 52–65. [Google Scholar] [PubMed]
120. Fu, C., Zeng, Q., Du, X. (2021). Hawatcher: Semantics-aware anomaly detection for appified smart homes. 30th {USENIX} Security Symposium ({USENIX} Security 21). https://www.usenix.org/conference/usenixsecurity21/presentation/fu-chenglong [Google Scholar]
121. Said, A. M., Yahyaoui, A., Abdellatif, T. (2021). Efficient anomaly detection for smart hospital IoT systems. Sensors, 21(4), 1026. [Google Scholar] [PubMed]
122. BritishPetroleum (2018). Twin win for oil and gas production| news and insights. https://www.bp.com/en/global/corporate/news-and-insights/reimagining-energy/apex-digital-system.html [Google Scholar]
123. Chen, X., Kang, E., Shiraishi, S., Preciado, V. M., Jiang, Z. (2018). Digital behavioral twins for safe connected cars. Proceedings of the 21th ACM/IEEE International Conference on Model Driven Engineering Languages and Systems, pp. 144–153. Copenhagen, Denmark. [Google Scholar]
124. Lehner, H., Dorffner, L. (2020). Digital geoTwin Vienna: Towards a digital twin city as geodata hub. PFG–Journal of Photogrammetry, Remote Sensing and Geoinformation Science, 88(1), 63–75. [Google Scholar]
125. Lu, Q., Parlikad, A. K., Woodall, P., Don Ranasinghe, G., Xie, X. et al. (2020). Developing a digital twin at building and city levels: Case study of West Cambridge campus. Journal of Management in Engineering, 36(3), 5020004. [Google Scholar]
126. China Academy of Information and Communication (2018). What would digital twin city of Xiongan be like in the future? Let’s take a look at the sample in reality (in Chinese). https://www.sohu.com/a/285056469_468661 [Google Scholar]
127. Cavada, M., Tight, M. R., Rogers, C. D. (2019). A smart city case study of Singapore—Is Singapore truly smart? In: Smart city emergence, pp. 295–314. Amsterdam: Elsevier. [Google Scholar]
128. Tian, F. (2021). Immersive 5G virtual reality visualization display system based on big-data digital city technology. Mathematical Problems in Engineering, 2021(12), 1–9. [Google Scholar]
129. Ham, Y., Kim, J. (2020). Participatory sensing and digital twin city: Updating virtual city models for enhanced risk-informed decision-making. Journal of Management in Engineering, 36(3), 4020005. [Google Scholar]
130. Fan, C., Jiang, Y., Mostafavi, A. (2020). Social sensing in disaster city digital twin: Integrated textual-visual–geo framework for situational awareness during built environment disruptions. Journal of Management in Engineering, 36(3), 04020002. [Google Scholar]
131. Deng, T., Zhang, K., Shen, Z. J. M. (2021). A systematic review of a digital twin city: A new pattern of urban governance toward smart cities. Journal of Management Science and Engineering, 6(2), 125–134. [Google Scholar]
132. Enzer, M., Bolton, A., Boulton, C., Byles, D., Cook, A. et al. (2019). Roadmap for delivering the information management framework for the built environment. https://www.repository.cam.ac.uk/items/5644aa11-2ec3-48d4-84d6-8e09ff219b90 [Google Scholar]
133. Kumar, H., Singh, M., Gupta, M. P., Madaan, J. K. (2020). Moving towards smart cities: Solutions that lead to the smart city transformation framework. Technological Forecasting and Social Change, 153(1), 119281. [Google Scholar]
134. Schrotter, G., Hürzeler, C. (2020). The digital twin of the city of Zurich for urban planning. PFG–Journal of Photogrammetry, Remote Sensing and Geoinformation Science, 88(1), 99–112. [Google Scholar]
135. Austin, M. A., Delgoshaei, P., Coelho, M., Heidarinejad, M. (2020). Architecting smart city digital twins: Combined semantic model and machine learning approach. Journal of Management in Engineering, 36(4), 4020026. [Google Scholar]
136. Dou, S., Zhang, H. H., Zhao, Y. Q., Wang, A., Xiong, Y. et al. (2020). Research on construction of spatio-tempopal data visualization platform for GIS and BIM fusion. ON International Archives of the Photogrammetry, Remote Sensing and Spatial Information Sciences, 42, 555–563. [Google Scholar]
137. Dembski, F., Wössner, U., Letzgus, M., Ruddat, M., Yamu, C. (2020). Urban digital twins for smart cities and citizens: The case study of Herrenberg, Germany. Sustainability, 12(6), 2307. [Google Scholar]
138. Dembski, F., Yamu, C., Wössner, U. (2019). Digital twin, virtual reality and space syntax: Civic engagement and decision support for smart, sustainable cities. Proceedings of the 12th Space Syntax Symposium, pp. 316.1–316.13. University of Groningen. [Google Scholar]
139. Nochta, T., Wan, L., Schooling, J. M., Parlikad, A. K. (2020). A socio-technical perspective on urban analytics: The case of city-scale digital twins. Journal of Urban Technology, 28(1–2), 263–287. [Google Scholar]
140. Nguyen, H. X., Trestian, R., To, D., Tatipamula, M. (2021). Digital twin for 5G and beyond. IEEE Communications Magazine, 59(2), 10–15. [Google Scholar]
Cite This Article
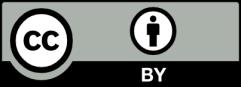