Open Access
ARTICLE
Reliability Analysis of HEE Parameters via Progressive Type-II Censoring with Applications
1
Department of Mathematical Sciences, College of Science, Princess Nourah bint Abdulrahman University, P.O. Box 84428,
Riyadh, 11671, Saudi Arabia
2
Department of Statistics, Faculty of Science, King Abdulaziz University, Jeddah, 21589, Saudi Arabia
3
Department of Statistics, Faculty of Commerce, Zagazig University, Zagazig, Egypt
4
Faculty of Technology and Development, Zagazig University, Zagazig, 44519, Egypt
* Corresponding Author: Ahmed Elshahhat. Email:
(This article belongs to the Special Issue: Application of Computer Tools in the Study of Mathematical Problems)
Computer Modeling in Engineering & Sciences 2023, 137(3), 2761-2793. https://doi.org/10.32604/cmes.2023.028826
Received 10 January 2023; Accepted 21 March 2023; Issue published 03 August 2023
Abstract
A new extended exponential lifetime model called Harris extended-exponential (HEE) distribution for data modelling with increasing and decreasing hazard rate shapes has been considered. In the reliability context, researchers prefer to use censoring plans to collect data in order to achieve a compromise between total test time and/or test sample size. So, this study considers both maximum likelihood and Bayesian estimates of the Harris extended-exponential distribution parameters and some of its reliability indices using a progressive Type-II censoring strategy. Under the premise of independent gamma priors, the Bayesian estimation is created using the squared-error and general entropy loss functions. Due to the challenging form of the joint posterior distribution, to evaluate the Bayes estimates, samples from the full conditional distributions are generated using Markov Chain Monte Carlo techniques. For each unknown parameter, the highest posterior density credible intervals and asymptotic confidence intervals are also determined. Through a simulated study, the usefulness of the various suggested strategies is assessed. The optimal progressive censoring plans are also shown, and various optimality criteria are investigated. Two actual data sets, taken from engineering and veterinary medicine areas, are analyzed to show how the offered point and interval estimators can be used in practice and to verify that the proposed model furnishes a good fit than other lifetime models namely: alpha power exponential, generalized-exponential, Nadarajah-Haghighi, Weibull, Lomax, gamma and exponential distributions. Numerical evaluations revealed that in the presence of progressively Type-II censored data, the Bayes estimation method against the squared-error (symmetric) loss is advised for getting the point and interval estimates of the HEE distribution.Keywords
Modelling actual data using generalized distributions is still important today. A variety of generalized distributions have been developed, and their usefulness in various contexts is investigated, see for example the work of Nadarajah et al. [1] and Mahdavi et al. [2]. By combining the exponential and Harris distributions, Pinho et al. [3] created a new three-parameter lifetime model known as the Harris extended-exponential (HEE) distribution. They emphasized that the HEE distribution can be utilized as a substitute of the Weibull and gamma distributions for modelling data with a rising or decreasing failure rate. As a result, it can be applied in a variety of domains, including but not limited to reliability, biology, engineering, insurance and epidemiology, among others.
However, if X is a random variable that follows the HEE distribution, denoted by
and
where
and
Fig. 1a displays different plots of the PDF using various choices of parameters. Similarly, Fig. 1b shows the plots of the HRF. Fig. 1a indicates that the PDF of the HEE distribution can be decreasing or unimodal. On the other hand, its HRF allows for decreasing and increasing shape hazard rates.
Figure 1: Shapes of the PDF and HRF of the HEE distribution
In reliability analysis and life testing studies, the examined items are commonly lost or discarded before failure. The obtained sample is therefore known as a censored sample. To preserve the functional experimental units for future use, shortening the duration of the test, and saving money are some major justifications for removing the experimental units. Various censoring techniques, such as time and failure censoring, are available in the literature; nevertheless, they do not have the ability to permit units to be eliminated at any moment other than the experiment’s endpoint. As a result, a more flexible censoring scheme known as progressive Type-II censoring is offered. For more in-depth analysis, or to be used as test samples in other research, certain items may need to be removed from the experiment.
The schematic representation of the progressive Type-II censoring scheme is as follows: Assume that
where
In literature, different censoring schemes were introduced; however, authors prefer to deal with progressive censoring over other censoring plans because it allows survival items to be withdrawn during the experiment at different stages which is not possible in case of failure (Type-II) censoring. Sultan et al. [5] investigated the classical and Bayesian estimation of inverse Weibull distribution. Dey et al. [6] investigated the maximum likelihood and Bayesian estimations for Marshall–Olkin extended exponential distribution. Kotb et al. [7] studied the inferences for modified Weibull distribution. Bdair et al. [8] analyzed the estimation and prediction for flexible Weibull distribution. Wu et al. [9] discussed the estimation and prediction problems for the Nadarajah–Haghighi parameters. Alotaibi et al. [10] considered the estimation of some reliability indices for alpha power Weibull distribution. Elshahhat et al. [11] considered the Bayesian life analysis of generalized Chen’s parameters. Dey et al. [12] studied various inferences for the Wilson–Hilferty parameters. For additional details regarding the concept of progressive censoring, one can guide Balakrishnan et al. [13].
Despite the flexibility and adaptability of the HEE distribution in modelling different types of data, to the best of our knowledge, there is no existing study on censoring mechanisms that deals with the estimation of parameters and/or reliability characteristics of the HEE distribution under incomplete (censored) sampling, which is of great interest and practical importance in many real-world scenarios. So, this study stands out in that it is the first to investigate the estimation issues for the HEE distribution when using incomplete data collected from a progressively Type-II censoring. Consequently, the main purposes for this study are fourfold:
• First objective explores various point and interval estimation issues of the HEE distribution parameters as well as its reliability characteristics utilizing progressive Type-II censored data, namely: maximum likelihood estimators (MLEs), Bayes estimators, approximate confidence intervals (ACIs) and highest posterior density (HPD) credible intervals.
• In Bayesian analysis, utilizing Markov Chain Monte Carlo (MCMC) techniques, the estimators are acquired by employing the squared-error (SE) and general entropy (GE) loss functions.
• The second objective performs extensive Monte Carlo simulations to compare the performance of the proposed estimation methods on the basis of their simulated root mean squared errors, mean absolute biases, average confidence lengths and coverage probabilities.
• The third objective is to check various optimality criteria to decide the best progressive censoring schemes.
• The last one is to demonstrate the ability of the proposed methods to work in practice by exploring two actual real data sets.
Before going any further, it is important to remember that the limitations of this study are: (i) All inferential methodologies are developed based on the assumption that at least one survival item was removed during the life-test; (ii) We assumed that the HEE parameters
The remaining sections of the study are organized as follows: Section 2 presents MLEs and ACIs. Section 3 investigates the Bayes estimators and HPD credible intervals. The results of the simulation study are included in Section 4. We offer several ways to select the optimum censoring scheme in Section 5. Section 6 highlights the analysis of two real data applications. The paper is finally concluded in Section 7.
In this section, the MLEs of the unknown parameters including the RF and HRF of the HEE distribution are investigated. Moreover, the ACIs based on the asymptotic properties of the MLEs are constructed. Assume that
where
where
and
where
Once the MLEs of
where
After getting the point estimates of
where the main diagonal elements are the estimated variances of
and
Now, the
where
On the other hand, the ACIs of the RF and HRF can be also obtained by approximating the variances of their estimators by using the delta method. See Greene [17] for more details about the delta method. In this case, we can approximate the variances of
and
with the following elements
where
Though the main problem of ACI for a positive parameter is that it may give a negative value in the lower bound. In literature, there are different approaches available to handle this problem. Theoretically, one of them is called the log-transformed maximum likelihood estimator developed by Meeker et al. [18]. Numerically, one can easily verify that the computed confidence intervals contain a lower bound with a positive value if one of them contains a negative lower bound, in which case this value is replaced by zero. Recently, this issue has been examined by Elshahhat et al. [11] and Elshahhat et al. [19].
In this section, we consider the Bayesian estimation method to derive the Bayesian estimators for
To get the Bayes estimates, we should first derive the joint posterior distribution of the unknown parameters. To obtain the joint posterior distribution, we combine the sample information given by (6) with the prior information about the unknown parameters given by (12) and apply the Bayes theorem. As a result, we can write the joint posterior distribution as follows:
where A is the normalized constant and obtained as
The loss function is crucial to Bayesian analysis because it may be used to characterize overestimation and underestimation in the investigation. Symmetric and asymmetric loss are two often employed loss functions. While the asymmetric loss function offers various weights to overestimation and underestimation, the symmetric loss function treats overestimation and underestimation equally. The asymmetric loss is more practical and advantageous in real-world applications than the symmetric loss see for more details Nassar et al. [21]. One of the most popular symmetric loss functions is the SE loss function, whereas the GE loss function is asymmetric. The SE and GE loss functions are considered in this work to acquire the Bayes estimates. It is generally known that the posterior mean is the Bayes estimator in the case of the SE loss function. On the other hand, the GE loss function provides varying weights for overestimation and underestimation.
Let
where
given that
and
where
and
Even though we have the full conditional distributions for each parameter, they do not have a known form, making it difficult to directly take samples from them. Therefore, we utilize the Metropolis–Hasting (M–H) algorithm to generate samples from these distributions. In order to derive the Bayesian estimates and the HPD credible intervals, we assume the normal distribution as the proposal distribution for the M–H sampling. Using the full conditional distributions given by (17)–(19), the required steps for the MH algorithm are as below
Step 1. Start with
Step 2. Determine the beginning values such that
Step 3. Generate
Step 4. From (18) and (19), generate
Step 5. Use the generated sample
Step 6. Put
Step 7. Replicate Steps 3–6, N times to get
In order to ensure convergence and remove the appeal of initial values, the first B generated variates are discarded. In this instance, we possess
Let
Likewise, the following formula can be applied to obtain the Bayes estimate of
The HPD credible interval specifies a range that covers the majority of the distribution, say
where the highest number less than or equal to
To assess how well the suggested point (or interval) estimators perform for
The proposed schemes S
Figure 2: Autocorrelation (left) and Trace (right) plots for MCMC draws of
Frequentist and Bayes estimates of
and
where
and
where
Following Henningsen et al. [14] and Plummer et al. [23], all numerical evaluations for both classical and Bayesian estimators of
Figure 3: Heatmap plots for the estimation results of
Figure 4: Heatmap plots for the estimation results of
Figure 5: Heatmap plots for the estimation results of
Figure 6: Heatmap plots for the estimation results of
Figure 7: Heatmap plots for the estimation results of
From Figs. 3–7, some observations can be drawn as:
• All offered estimates of the unknown HEE parameters
• All estimated estimations perform satisfactorily as
• Comparing the point estimation methods of
• Comparing the proposed interval estimation methods of
• The Prior-II behaves better than the Prior-I in terms of the lowest values of RMSE, MAB, and ACL, as well as the greatest values of CP, when comparing the Bayes estimates of all unknown parameters based on Priors I and II. This outcome was expected because the associated variance of Prior-II is lower than that of Prior-I.
• Comparing S1 and S3, in terms of the smallest RMSE, MAB and ACL values and highest CP values, it is observed that the proposed estimates of
• Among the calculated estimates, the methods for estimating model parameters or reliability properties show superior performance using S4 which usually provides the best results for all unknown parameters as expected. It is an expected result because the acquired estimates have been obtained based on all information included in the complete sample.
• Finally, when data is acquired from a progressively Type-II censored sampling plan, the Bayes inferences based on the M-H algorithm are advised to estimate the unknown parameters and the reliability measures of the HEE lifespan distribution.
5 Optimal Progressive Censoring Plan
The topic of how to pick a specific progressive censoring scheme naturally arises. Should we select a certain plan just on the basis of convenience or should we consider some statistical factors? The statistical literature has drawn a lot of attention to the topic of selecting the best censoring schemes. Finding the progressive censoring plan that provides the most information about the unknown parameters among all potential progressive censoring plans is necessary for choosing the best sampling approach. Here, probable censoring schemes relate to all
We wish to maximize the trace of the observed FI matrix in terms of criterion I. Additionally, we want to reduce the determinant and trace of
The variance of
In this section, two real-world data sets from the engineering and veterinary industries are investigated to see how the estimating approaches suggested in this study perform in actual use.
The time-to-failure (10
We fit the HEE distribution and compare it to other seven lifetime distributions as its competitors, including the alpha power exponential (APE), generalized-exponential (GE), Nadarajah-Haghighi (NH), Weibull (W), G, Lomax (L), and exponential (E) distributions. For
Several model selection criteria, including negative log–likelihood (NL), Akaike’s (A), Bayesian (B), consistent Akaike’s (CA), and Hannan–Quinn (HQ) information criteria, are utilized to show the HEE distribution’s utility in comparison to its rival models. Three alternative goodness–of–fit statistics, namely, Anderson–Darling (AD), Cramér–von Mises (CvM), and Kolmogorov–Smirnov (KS) (with its p-value) statistics are also used to assess the validity of the HEE model in comparison to other competitor models. Based on these measures, the best distribution corresponds to the lowest value of A, B, CA, HQ, AD, CvM and KS statistics as well as to the highest p-value via
Figure 8: The quantile-quantile plots of the HEE distribution and other competing models using turbochargers data
Figure 9: Estimated densities and reliability functions of the HEE distribution and other competing models using turbochargers data
From the complete turbochargers data, three different progressively Type-II censored samples with
Since no prior information is available for HEE parameters
In MCMC iterations, the convergence of simulated chains of each unknown parameter must be checked. Therefore, based on the data set of
Figure 10: Trace plots (left) and Histogram plots (right) for simulated MCMC samples of
On the basis of the data from the turbochargers, the problem of selecting the optimal censoring scheme out of all available censoring methods is also examined. The recommended optimum criteria in Table 1 are determined from the generated samples reported in Table 6 and listed in Table 10. It is clear that the progressive censoring plan
In this application, from veterinary medicine field, we shall provide an analysis for the survival times (in days) of 72 guinea-pigs infected with virulent tubercle bacilli. This data set was originally discussed and reported by Bjerkedal [35] and recently also explained by Chhetri et al. [36]. For computational convenience, each lifetime point in the original guinea-pigs data set is divided by one hundred. In Table 11, the new transformed survival times are reported in ascending order.
Again, to illustrate how the HEE distribution can be used effectively to provide a better fit than the other distributions, the guinea-pigs data set is also analyzed for this purpose. From Table 11, the MLEs (with their St.Errs) of all considered distributions (given in Table 3) are calculated and reported in Table 12. Furthermore, the different goodness-of-fit criteria are also computed and reported in Table 13. It is clear, from Table 13, that the HEE distribution is the best statistical model compared to other fitted models for fitting guinea-pigs data set because it has the lowest values of the different goodness-of-fit measures and the highest p-value. Fig. 11 shows the quantile-quantile plots for the considered distributions. Furthermore, the histogram and the different fitted densities as well as the empirical and fitted reliability functions are displayed in Figs. 12a and 12b, respectively. Figs. 11 and 12 support the same findings reported in Table 13. Now, from the complete guinea-pigs data, different progressively Type-II censored samples with fixed
Figure 11: The quantile-quantile plots of the HEE distribution and other competing models using guinea-pigs data
Figure 12: Estimated densities and reliability functions of the HEE distribution and other competing models using guinea-pigs data
Using the generated sample
Figure 13: Trace plots (top panel) and Histogram plots (bottom panel) for simulated MCMC samples of
In this work, we have acquired both classical and Bayesian estimations of the Harris extended-exponential distribution in the existence of progressively Type-II censoring samples. The maximum likelihood approach is taken into account in the context of classical estimation in order to get the point and interval estimations of the unknown parameters, reliability, and hazard rate functions. On the other hand, under the premise of independent gamma priors, the Bayesian estimation is created based on both squared and general entropy loss functions. Due to the problematic presentation of the posterior distribution, the Markov Chain Monte Carlo technique is employed to get the Bayes estimates as well as the highest posterior density credible intervals. Monte Carlo simulations are implemented to compare the performance of the diverse point and interval estimators while accounting for various sample sizes and censoring procedures. Some optimality criteria are investigated to discover the best progressive censoring scheme. We examined two data sets for guinea pigs and turbochargers to show how the suggested estimators perform in practical environments. These applications showed also that the Harris extended-exponential distribution provides a better fit than other seven distributions in the literature. To be more specific, the numerical investigations showed that the Bayesian MCMC technique yields more accurate estimates compared to others and is recommended when the progressively Type-II censored Harris extended-exponential data exist. In future work, it may be preferable to reuse the proposed estimation methods to include competing risks or accelerated tests data.
Acknowledgement: The authors would desire to express their gratitude to the editor and the anonymous referees for useful advice and helpful comments. The authors would also like to express their full thanks to Princess Nourah bint Abdulrahman University, Riyadh, Saudi Arabia, for supporting this study.
Funding Statement: This research was funded by Princess Nourah bint Abdulrahman University Researchers Supporting Project Number (PNURSP2023R175), Princess Nourah bint Abdulrahman University, Riyadh, Saudi Arabia.
Conflicts of Interest: The authors declare that they have no conflicts of interest to report regarding the present study.
References
1. Nadarajah, S., Haghighi, F. (2011). An extension of the exponential distribution. Statistics, 45(6), 543–558. [Google Scholar]
2. Mahdavi, A., Kundu, D. (2017). A new method for generating distributions with an application to exponential distribution. Communications in Statistics-Theory and Methods, 46(13), 6543–6557. [Google Scholar]
3. Pinho, L. G. B., Cordeiro, G. M., Nobre, J. S. (2015). The Harris extended exponential distribution. Communications in Statistics-Theory and Methods, 44(16), 3486–3502. [Google Scholar]
4. Marshall, A. W., Olkin, I. (1997). A new method for adding a parameter to a family of distributions with application to the exponential and Weibull families. Biometrika, 84(3), 641–652. [Google Scholar]
5. Sultan, K. S., Alsadat, N. H., Kundu, D. (2014). Bayesian and maximum likelihood estimations of the inverse Weibull parameters under progressive Type-II censoring. Journal of Statistical Computation and Simulation, 84(10), 2248–2265. [Google Scholar]
6. Dey, S., Nassar, M., Maurya, R. K., Tripathi, Y. M. (2018). Estimation and prediction of Marshall–Olkin extended exponential distribution under progressively Type-II censored data. Journal of Statistical Computation and Simulation, 88(12), 2287–2308. [Google Scholar]
7. Kotb, M. S., Raqab, M. Z. (2019). Statistical inference for modified Weibull distribution based on progressively type-II censored data. Mathematics and Computers in Simulation, 162(6), 233–248. [Google Scholar]
8. Bdair, O. M., Awwad, R. R., Abufoudeh, G. K., Naser, M. F. M. (2020). Estimation and prediction for flexible Weibull distribution based on progressive type II censored data. Communications in Mathematics and Statistics, 8(3), 255–277. [Google Scholar]
9. Wu, M., Gui, W. (2021). Estimation and prediction for nadarajah-haghighi distribution under progressive Type-II censoring. Symmetry, 13(6), 999. [Google Scholar]
10. Alotaibi, R., Nassar, M., Rezk, H., Elshahhat, A. (2022). Inferences and engineering applications of alpha power weibull distribution using progressive Type-II censoring. Mathematics, 10(16), 2901. https://doi.org/10.3390/math10162901 [Google Scholar] [CrossRef]
11. Elshahhat, A., Rastogi, M. K. (2022). Bayesian life analysis of generalized chen’s population under progressive censoring. Pakistan Journal of Statistics and Operation Research, 18(3), 675–702. [Google Scholar]
12. Dey, S., Elshahhat, A. (2022). Analysis of Wilson–Hilferty distribution under progressive Type-II censoring. Quality and Reliability Engineering International, 38(7), 3771–3796. [Google Scholar]
13. Balakrishnan, N., Cramer, E. (2014). The art of progressive censoring. Birkhäuser, New York: Springer. [Google Scholar]
14. Henningsen, A., Toomet, O. (2011). maxLik: A package for maximum likelihood estimation in R. Computational Statistics, 26(3), 443–458. [Google Scholar]
15. Panahi, H. (2017). Estimation of the Burr type III distribution with application in unified hybrid censored sample of fracture toughness. Journal of Applied Statistics, 44(14), 2575–2592. [Google Scholar]
16. Panahi, H. (2017). Estimation methods for the generalized inverted exponential distribution under type ii progressively hybrid censoring with application to spreading of micro-drops data. Communications in Mathematics and Statistics, 5(2), 159–174. [Google Scholar]
17. Greene, W. H. (2000). Econometric analysis, 4th edition. New York, NY, USA: Prentice-Hall. [Google Scholar]
18. Meeker, W. Q., Escobar, L. A. (2014). Statistical methods for reliability data. NY, USA: John Wiley & Sons. [Google Scholar]
19. Elshahhat, A., Muse, A. H., Egeh, O. M., Elemary, B. R. (2022). Estimation for parameters of life of the Marshall-Olkin generalized-exponential distribution using progressive Type-II censored data. Complexity, 2022, 36, 8155929. https://doi.org/10.1155/2022/8155929 [Google Scholar] [CrossRef]
20. Dey, S., Elshahhat, A., Nassar, M. (2022). Analysis of progressive type-II censored gamma distribution. Computational Statistics, 38, 481–508. https://doi.org/10.1007/s00180-022-01239-y [Google Scholar] [CrossRef]
21. Nassar, M., Alotaibi, R., Okasha, H., Wang, L. (2022). Bayesian estimation using expected LINEX loss function: A novel approach with applications. Mathematics, 10(3), 436. [Google Scholar]
22. Calabria, R., Pulcini, G. (1994). An engineering approach to Bayes estimation for the Weibull distribution. Microelectronics Reliability, 34(5), 789–802. [Google Scholar]
23. Plummer, M., Best, N., Cowles, K., Vines, K. (2006). CODA: Convergence diagnosis and output analysis for MCMC. R News, 6(1), 7–11. [Google Scholar]
24. Elshahhat, A., Rastogi, M. K. (2021). Estimation of parameters of life for an inverted Nadarajah–Haghighi distribution from Type–II progressively censored samples. Journal of the Indian Society for Probability and Statistics, 22(1), 113–154. [Google Scholar]
25. Ng, H. K. T., Chan, C. S., Balakrishnan, N. (2004). Optimal progressive censoring plans for the Weibull distribution. Technometrics, 46(4), 470–481. [Google Scholar]
26. Kundu, D. (2008). Bayesian inference and life testing plan for the Weibull distribution in presence of progressive censoring. Technometrics, 50(2), 144–154. [Google Scholar]
27. Pradhan, B., Kundu, D. (2009). On progressively censored generalized exponential distribution. Test, 18, 497–515. [Google Scholar]
28. Guerra, R. R., Peña-Ramírez, F. A., Cordeiro, G. M. (2021). The Weibull Burr XII distribution in lifetime and income analysis. Anais da Academia Brasileira de Ciências, 93(3), 1–28. [Google Scholar]
29. Xu, K., Xie, M., Tang, L. C., Ho, S. L. (2003). Application of neural networks in forecasting engine systems reliability. Applied Soft Computing, 2(4), 255–268. [Google Scholar]
30. Gupta, R. D., Kundu, D. (2001). Generalized exponential distribution: Different method of estimations. Journal of Statistical Computation and Simulation, 69(4), 315–337. [Google Scholar]
31. Weibull, W. (1951). A statistical distribution function of wide applicability. Journal of Applied Mechanics, 18, 293–297. [Google Scholar]
32. Johnson, N., Kotz, S., Balakrishnan, N. (1994). Continuous univariate distributions, 2nd edition. NY, USA: John Wiley and Sons. [Google Scholar]
33. Lomax, K. S. (1954). Business failures: Another example of the analysis of failure data. Journal of the American Statistical Association, 49, 847–852. [Google Scholar]
34. Marinho, P. R. D., Silva, R. B., Bourguignon, M., Cordeiro, G. M., Nadarajah, S. (2019). AdequacyModel: An R package for probability distributions and general purpose optimization. PLoS One, 14, e0221487. [Google Scholar] [PubMed]
35. Bjerkedal, T. (1960). Acquisition of resistance in guinea pies infected with different doses of virulent tubercle bacilli. American Journal of Hygiene, 72(1), 130–148. [Google Scholar] [PubMed]
36. Chhetri, S., Mdziniso, N., Ball, C. (2022). Extended Lindley distribution with applications. Revista Colombiana de Estadística, 45(1), 65–83. [Google Scholar]
Table S1: The average estimates (1st column), RMSEs (2nd column) and MABs (3rd column) of α
Table S2: The average estimates (1st column), RMSEs (2nd column) and MABs (3rd column) of β
Table S3: The average estimates (1st column), RMSEs (2nd column) and MABs (3rd column) of θ
Table S4: The average estimates (1st column), RMSEs (2nd column) and MABs (3rd column) of R(t)
Table S5: The average estimates (1st column), RMSEs (2nd column) and MABs (3rd column) of h(t)
Table S6: The ACLs (1st column) and CPs (2nd column) of 95% ACI/HPD credible intervals of α
Table S7: The ACLs (1st column) and CPs (2nd column) of 95% ACI/HPD credible intervals of β
Table S8: The ACLs (1st column) and CPs (2nd column) of 95% ACI/HPD credible intervals of θ
Table S9: The ACLs (1st column) and CPs (2nd column) of 95% ACI/HPD credible intervals of R(t)
Table S10: The ACLs (1st column) and CPs (2nd column) of 95% ACI/HPD credible intervals of h(t)
Figure S1: Trace plots (left) and Histogram plots (right) for simulated MCMC samples of α, β, θ, R(t) and h(t) using sample S2 from turbochargers data
Figure S2: Trace plots (left) and Histogram plots (right) for simulated MCMC samples of α, β, θ, R(t) and h(t) using sample S3 from turbochargers data
Figure S3: Trace plots (top panel) and Histogram plots (bottom panel) for simulated MCMC samples of α, β, θ, R(t) and h(t) using sample S2 from guinea-pigs data
Figure S4: Trace plots (top panel) and Histogram plots (bottom panel) for simulated MCMC samples of α, β, θ, R(t) and h(t) using sample S3 from guinea-pigs data
Cite This Article
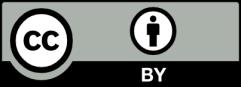