Open Access
ARTICLE
A Time-Varying Parameter Estimation Method for Physiological Models Based on Physical Information Neural Networks
1
College of Information and Electrical Engineering, China Agricultural University, Beijing, 100083, China
2
Key Laboratory of Agricultural Information Acquisition Technology (Beijing), Ministry of Agriculture, Beijing, 100083, China
3
Key Laboratory of Modern Precision Agriculture System Integration Research, Ministry of Education, Beijing, 100083, China
* Corresponding Author: Lan Huang. Email:
Computer Modeling in Engineering & Sciences 2023, 137(3), 2243-2265. https://doi.org/10.32604/cmes.2023.028101
Received 29 November 2022; Accepted 23 March 2023; Issue published 03 August 2023
Abstract
In the establishment of differential equations, the determination of time-varying parameters is a difficult problem, especially for equations related to life activities. Thus, we propose a new framework named BioE-PINN based on a physical information neural network that successfully obtains the time-varying parameters of differential equations. In the proposed framework, the learnable factors and scale parameters are used to implement adaptive activation functions, and hard constraints and loss function weights are skillfully added to the neural network output to speed up the training convergence and improve the accuracy of physical information neural networks. In this paper, taking the electrophysiological differential equation as an example, the characteristic parameters of ion channel and pump kinetics are determined using BioE-PINN. The results demonstrate that the numerical solution of the differential equation is calculated by the parameters predicted by BioE-PINN, the Root Mean Square Error (RMSE) is between 0.01 and 0.3, and the Pearson coefficient is above 0.87, which verifies the effectiveness and accuracy of BioE-PINN. Moreover, real measured membrane potential data in animals and plants are employed to determine the parameters of the electrophysiological equations, with RMSE 0.02-0.2 and Pearson coefficient above 0.85. In conclusion, this framework can be applied not only for differential equation parameter determination of physiological processes but also the prediction of time-varying parameters of equations in other fields.Keywords
Cite This Article
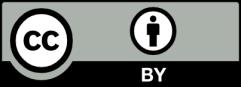
This work is licensed under a Creative Commons Attribution 4.0 International License , which permits unrestricted use, distribution, and reproduction in any medium, provided the original work is properly cited.