Open Access
ARTICLE
Sparsity-Enhanced Model-Based Method for Intelligent Fault Detection of Mechanical Transmission Chain in Electrical Vehicle
1
School of Aerospace Science and Technology, Xidian University, Xi’an, 710071, China
2
Guangzhou Institute of Technology, Xidian University, Guangzhou, 510555, China
3
Research Center for Applied Mechanics, Key Laboratory of Ministry of Education for Electronic Equipment Structure Design,
Xidian University, Xi’an, 710071, China
* Corresponding Author: Wangpeng He. Email:
(This article belongs to the Special Issue: AI and Machine Learning Modeling in Civil and Building Engineering)
Computer Modeling in Engineering & Sciences 2023, 137(3), 2495-2511. https://doi.org/10.32604/cmes.2023.027896
Received 20 November 2022; Accepted 23 March 2023; Issue published 03 August 2023
Abstract
In today’s world, smart electric vehicles are deeply integrated with smart energy, smart transportation and smart cities. In electric vehicles (EVs), owing to the harsh working conditions, mechanical parts are prone to fatigue damages, which endanger the driving safety of EVs. The practice has proved that the identification of periodic impact characteristics (PICs) can effectively indicate mechanical faults. This paper proposes a novel model-based approach for intelligent fault diagnosis of mechanical transmission train in EVs. The essential idea of this approach lies in the fusion of statistical information and model information from a dynamic process. In the algorithm, a novel fractal wavelet decomposition (FWD) is used to investigate the time-frequency representation of the input signal. Based on the sparsity of the PIC model in the Hilbert envelope spectrum, a method for evaluating PIC energy ratio (PICER) is defined based on an over-complete Fourier dictionary. A compound indicator considering kurtosis and PICER of dynamic signal is designed. Using this index, evaluations of the impulsiveness of the cycle-stationary process can be enabled, thus avoiding serious interference from the sporadic impact during measurements. The robustness of the proposed approach to noise is demonstrated via numerical simulations, and an engineering application is employed to validate its effectiveness.Keywords
Cite This Article
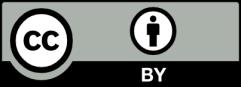
This work is licensed under a Creative Commons Attribution 4.0 International License , which permits unrestricted use, distribution, and reproduction in any medium, provided the original work is properly cited.