Open Access
ARTICLE
Brain Functional Network Generation Using Distribution-Regularized Adversarial Graph Autoencoder with Transformer for Dementia Diagnosis
1
School of Information Engineering, Hubei University of Economics, Wuhan, 430205, China
2
State Key Laboratory of Simulation and Regulation of Water Cycle in River Basin, China Institute of Water Resources
and Hydropower Research, Beijing, 100038, China
3
School of Computing and Mathematic Sciences, University of Leicester, Leicester, LE1 7RH, UK
4
Shenzhen Institutes of Advanced Technology, Chinese Academy of Sciences, Shenzhen, 518055, China
5
Faculty of Science and Technology, University of Macau, Macau, 999078, China
6
School of Geophysics, Chengdu University of Technology, Chengdu, 610059, China
7
Laboratory of Artificial Intelligence and 3D Technologies for Cardiovascular Diseases, Guangdong Provincial Key Laboratory
of South China Structural Heart Disease, Guangdong Provincial People’s Hospital (Guangdong Academy of Medical Sciences),
Southern Medical University, Guangzhou, 519041, China
8
Medical Research Institute, Guangdong Provincial People’s Hospital (Guangdong Academy of Medical Sciences),
Southern Medical University, Guangzhou, 519041, China
* Corresponding Authors: Yudong Zhang. Email: ; Jin Hong. Email:
Computer Modeling in Engineering & Sciences 2023, 137(3), 2129-2147. https://doi.org/10.32604/cmes.2023.028732
Received 04 January 2023; Accepted 20 March 2023; Issue published 03 August 2023
Abstract
The topological connectivity information derived from the brain functional network can bring new insights for diagnosing and analyzing dementia disorders. The brain functional network is suitable to bridge the correlation between abnormal connectivities and dementia disorders. However, it is challenging to access considerable amounts of brain functional network data, which hinders the widespread application of data-driven models in dementia diagnosis. In this study, a novel distribution-regularized adversarial graph auto-Encoder (DAGAE) with transformer is proposed to generate new fake brain functional networks to augment the brain functional network dataset, improving the dementia diagnosis accuracy of data-driven models. Specifically, the label distribution is estimated to regularize the latent space learned by the graph encoder, which can make the learning process stable and the learned representation robust. Also, the transformer generator is devised to map the node representations into node-to-node connections by exploring the long-term dependence of highly-correlated distant brain regions. The typical topological properties and discriminative features can be preserved entirely. Furthermore, the generated brain functional networks improve the prediction performance using different classifiers, which can be applied to analyze other cognitive diseases. Attempts on the Alzheimer’s Disease Neuroimaging Initiative (ADNI) dataset demonstrate that the proposed model can generate good brain functional networks. The classification results show adding generated data can achieve the best accuracy value of 85.33%, sensitivity value of 84.00%, specificity value of 86.67%. The proposed model also achieves superior performance compared with other related augmented models. Overall, the proposed model effectively improves cognitive disease diagnosis by generating diverse brain functional networks.Graphic Abstract
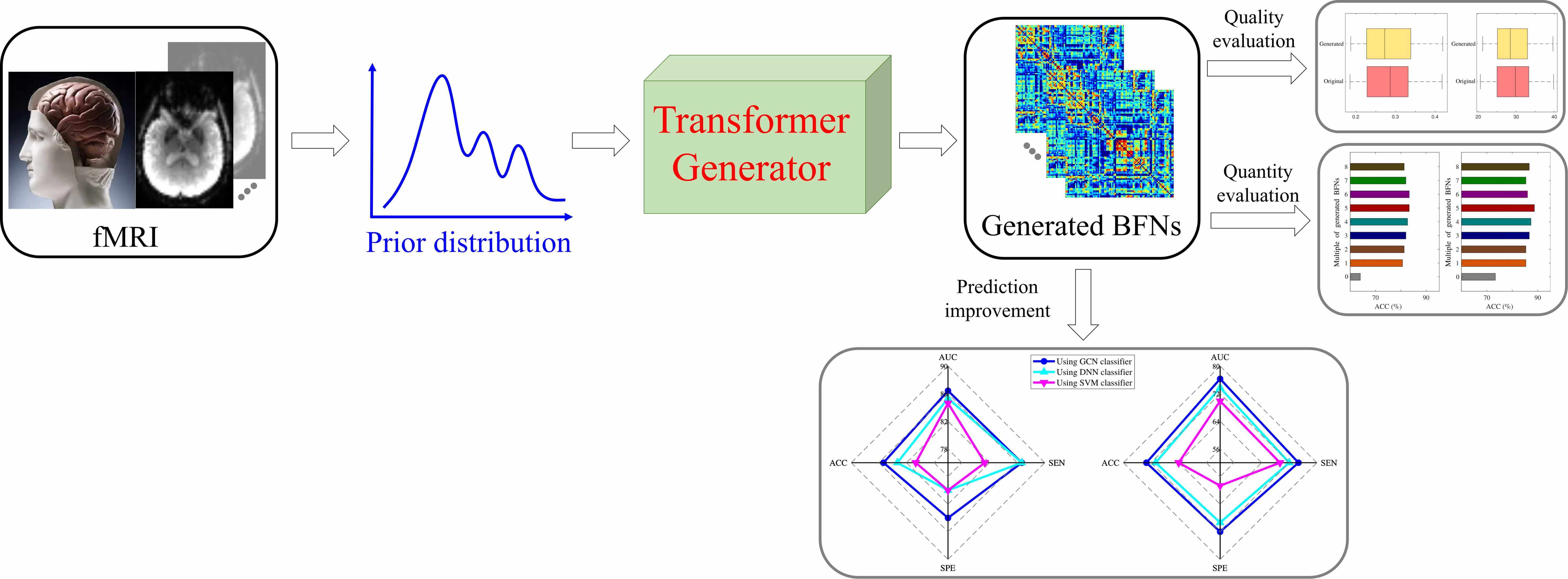
Keywords
Cite This Article
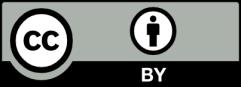
This work is licensed under a Creative Commons Attribution 4.0 International License , which permits unrestricted use, distribution, and reproduction in any medium, provided the original work is properly cited.