Open Access
ARTICLE
An Improved High Precision 3D Semantic Mapping of Indoor Scenes from RGB-D Images
1
Shaanxi Key Laboratory of Complex System Control and Intelligent Information Processing, Xi’an University of Technology,
Xi’an, 710048, China
2
Australian Centre for Field Robotics, The University of Sydney, Sydney, 2006, Australia
* Corresponding Author: Jing Xin. Email:
(This article belongs to the Special Issue: Advanced Intelligent Decision and Intelligent Control with Applications in Smart City)
Computer Modeling in Engineering & Sciences 2023, 137(3), 2621-2640. https://doi.org/10.32604/cmes.2023.027467
Received 31 October 2022; Accepted 21 March 2023; Issue published 03 August 2023
Abstract
This paper proposes an improved high-precision 3D semantic mapping method for indoor scenes using RGB-D images. The current semantic mapping algorithms suffer from low semantic annotation accuracy and insufficient real-time performance. To address these issues, we first adopt the Elastic Fusion algorithm to select key frames from indoor environment image sequences captured by the Kinect sensor and construct the indoor environment space model. Then, an indoor RGB-D image semantic segmentation network is proposed, which uses multi-scale feature fusion to quickly and accurately obtain object labeling information at the pixel level of the spatial point cloud model. Finally, Bayesian updating is used to conduct incremental semantic label fusion on the established spatial point cloud model. We also employ dense conditional random fields (CRF) to optimize the 3D semantic map model, resulting in a high-precision spatial semantic map of indoor scenes. Experimental results show that the proposed semantic mapping system can process image sequences collected by RGB-D sensors in real-time and output accurate semantic segmentation results of indoor scene images and the current local spatial semantic map. Finally, it constructs a globally consistent high-precision indoor scenes 3D semantic map.Keywords
Cite This Article
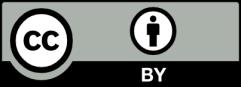
This work is licensed under a Creative Commons Attribution 4.0 International License , which permits unrestricted use, distribution, and reproduction in any medium, provided the original work is properly cited.