Open Access
ARTICLE
Stochastic Programming for Hub Energy Management Considering Uncertainty Using Two-Point Estimate Method and Optimization Algorithm
1
Department of Electrical Engineering, College of Engineering, Majmaah University, Al-Majmaah, 11952, Saudi Arabia
2
Department of Electrical Engineering, College of Engineering, Jouf University, Sakaka, 72341, Saudi Arabia
3
Department of Electrical Engineering, College of Engineering, Northern Border University, Ar’Ar, 73222, Saudi Arabia
4
Automation and Control Department, College of Engineering Technology, University of Doha for Science and Technology, Doha,
24449, Qatar
5
Department of Electrical and Computer Engineering, College of Engineering and Information Technology, Ajman University,
Ajman, 20550, United Arab Emirates
6
Department of Physics, College of Science, Imam Abdulrahman Bin Faisal University, Dammam, 31441, Saudi Arabia
* Corresponding Author: Yazeed Qasaymeh. Email:
Computer Modeling in Engineering & Sciences 2023, 137(3), 2163-2192. https://doi.org/10.32604/cmes.2023.029453
Received 20 February 2023; Accepted 31 March 2023; Issue published 03 August 2023
Abstract
To maximize energy profit with the participation of electricity, natural gas, and district heating networks in the day-ahead market, stochastic scheduling of energy hubs taking into account the uncertainty of photovoltaic and wind resources, has been carried out. This has been done using a new meta-heuristic algorithm, improved artificial rabbits optimization (IARO). In this study, the uncertainty of solar and wind energy sources is modeled using Hang’s two-point estimating method (TPEM). The IARO algorithm is applied to calculate the best capacity of hub energy equipment, such as solar and wind renewable energy sources, combined heat and power (CHP) systems, steam boilers, energy storage, and electric cars in the day-ahead market. The standard ARO algorithm is developed to mimic the foraging behavior of rabbits, and in this work, the algorithm’s effectiveness in avoiding premature convergence is improved by using the dystudynamic inertia weight technique. The proposed IARO-based scheduling framework’s performance is evaluated against that of traditional ARO, particle swarm optimization (PSO), and salp swarm algorithm (SSA). The findings show that, in comparison to previous approaches, the suggested meta-heuristic scheduling framework based on the IARO has increased energy profit in day-ahead electricity, gas, and heating markets by satisfying the operational and energy hub limitations. Additionally, the results show that TPEM approach dependability consideration decreased hub energy’s profit by 8.995% as compared to deterministic planning.Keywords
Cite This Article
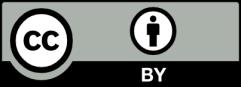
This work is licensed under a Creative Commons Attribution 4.0 International License , which permits unrestricted use, distribution, and reproduction in any medium, provided the original work is properly cited.