Open Access
ARTICLE
Transductive Transfer Dictionary Learning Algorithm for Remote Sensing Image Classification
1
Aliyun School of Big Data, Changzhou University, Changzhou, 213164, China
2
Hua Lookeng Honors College, Changzhou University, Changzhou, 213164, China
* Corresponding Author: Tongguang Ni. Email:
(This article belongs to the Special Issue: Computer Modeling for Smart Cities Applications)
Computer Modeling in Engineering & Sciences 2023, 137(3), 2267-2283. https://doi.org/10.32604/cmes.2023.027709
Received 10 November 2022; Accepted 24 March 2023; Issue published 03 August 2023
Abstract
To create a green and healthy living environment, people have put forward higher requirements for the refined management of ecological resources. A variety of technologies, including satellite remote sensing, Internet of Things, artificial intelligence, and big data, can build a smart environmental monitoring system. Remote sensing image classification is an important research content in ecological environmental monitoring. Remote sensing images contain rich spatial information and multi-temporal information, but also bring challenges such as difficulty in obtaining classification labels and low classification accuracy. To solve this problem, this study develops a transductive transfer dictionary learning (TTDL) algorithm. In the TTDL, the source and target domains are transformed from the original sample space to a common subspace. TTDL trains a shared discriminative dictionary in this subspace, establishes associations between domains, and also obtains sparse representations of source and target domain data. To obtain an effective shared discriminative dictionary, triple-induced ordinal locality preserving term, Fisher discriminant term, and graph Laplacian regularization term are introduced into the TTDL. The triplet-induced ordinal locality preserving term on sub-space projection preserves the local structure of data in low-dimensional subspaces. The Fisher discriminant term on dictionary improves differences among different sub-dictionaries through intra-class and inter-class scatters. The graph Laplacian regularization term on sparse representation maintains the manifold structure using a semi-supervised weight graph matrix, which can indirectly improve the discriminative performance of the dictionary. The TTDL is tested on several remote sensing image datasets and has strong discrimination classification performance.Keywords
Cite This Article
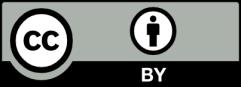
This work is licensed under a Creative Commons Attribution 4.0 International License , which permits unrestricted use, distribution, and reproduction in any medium, provided the original work is properly cited.