Open Access
REVIEW
Recent Advances of Deep Learning in Geological Hazard Forecasting
1
Key Laboratory of in-Situ Property-Improving Mining of Ministry of Education, Taiyuan University of Technology,
Taiyuan, 030024, China
2
School of Architectural and Civil Engineering, Huanghuai University, Zhumadian, 463003, China
3
School of Energy Engineering, Xi’an University of Science and Technology, Xi’an, 710054, China
* Corresponding Author: Haojie Lian. Email:
Computer Modeling in Engineering & Sciences 2023, 137(2), 1381-1418. https://doi.org/10.32604/cmes.2023.023693
Received 09 May 2022; Accepted 22 February 2023; Issue published 26 June 2023
Abstract
Geological hazard is an adverse geological condition that can cause loss of life and property. Accurate prediction and analysis of geological hazards is an important and challenging task. In the past decade, there has been a great expansion of geohazard detection data and advancement in data-driven simulation techniques. In particular, great efforts have been made in applying deep learning to predict geohazards. To understand the recent progress in this field, this paper provides an overview of the commonly used data sources and deep neural networks in the prediction of a variety of geological hazards.Keywords
Cite This Article
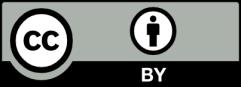