Open Access
ARTICLE
Enhanced Harris Hawks Optimization Integrated with Coot Bird Optimization for Solving Continuous Numerical Optimization Problems
College of Mechanical and Electrical Engineering, Northeast Forestry University, Harbin, 150040, China
* Corresponding Authors: Yanling Guo. Email: ; Yangwei Wang. Email:
(This article belongs to the Special Issue: Bio-inspired Computer Modelling: Theories and Applications in Engineering and Sciences)
Computer Modeling in Engineering & Sciences 2023, 137(2), 1635-1675. https://doi.org/10.32604/cmes.2023.026019
Received 10 August 2022; Accepted 30 January 2023; Issue published 26 June 2023
Abstract
Harris Hawks Optimization (HHO) is a novel meta-heuristic algorithm that imitates the predation characteristics of Harris Hawk and combines Lévy flight to solve complex multidimensional problems. Nevertheless, the basic HHO algorithm still has certain limitations, including the tendency to fall into the local optima and poor convergence accuracy. Coot Bird Optimization (CBO) is another new swarm-based optimization algorithm. CBO originates from the regular and irregular motion of a bird called Coot on the water’s surface. Although the framework of CBO is slightly complicated, it has outstanding exploration potential and excellent capability to avoid falling into local optimal solutions. This paper proposes a novel enhanced hybrid algorithm based on the basic HHO and CBO named Enhanced Harris Hawks Optimization Integrated with Coot Bird Optimization (EHHOCBO). EHHOCBO can provide higher-quality solutions for numerical optimization problems. It first embeds the leadership mechanism of CBO into the population initialization process of HHO. This way can take full advantage of the valuable solution information to provide a good foundation for the global search of the hybrid algorithm. Secondly, the Ensemble Mutation Strategy (EMS) is introduced to generate the mutant candidate positions for consideration, further improving the hybrid algorithm’s exploration trend and population diversity. To further reduce the likelihood of falling into the local optima and speed up the convergence, Refracted Opposition-Based Learning (ROBL) is adopted to update the current optimal solution in the swarm. Using 23 classical benchmark functions and the IEEE CEC2017 test suite, the performance of the proposed EHHOCBO is comprehensively evaluated and compared with eight other basic meta-heuristic algorithms and six improved variants. Experimental results show that EHHOCBO can achieve better solution accuracy, faster convergence speed, and a more robust ability to jump out of local optima than other advanced optimizers in most test cases. Finally, EHHOCBO is applied to address four engineering design problems. Our findings indicate that the proposed method also provides satisfactory performance regarding the convergence accuracy of the optimal global solution.Graphic Abstract
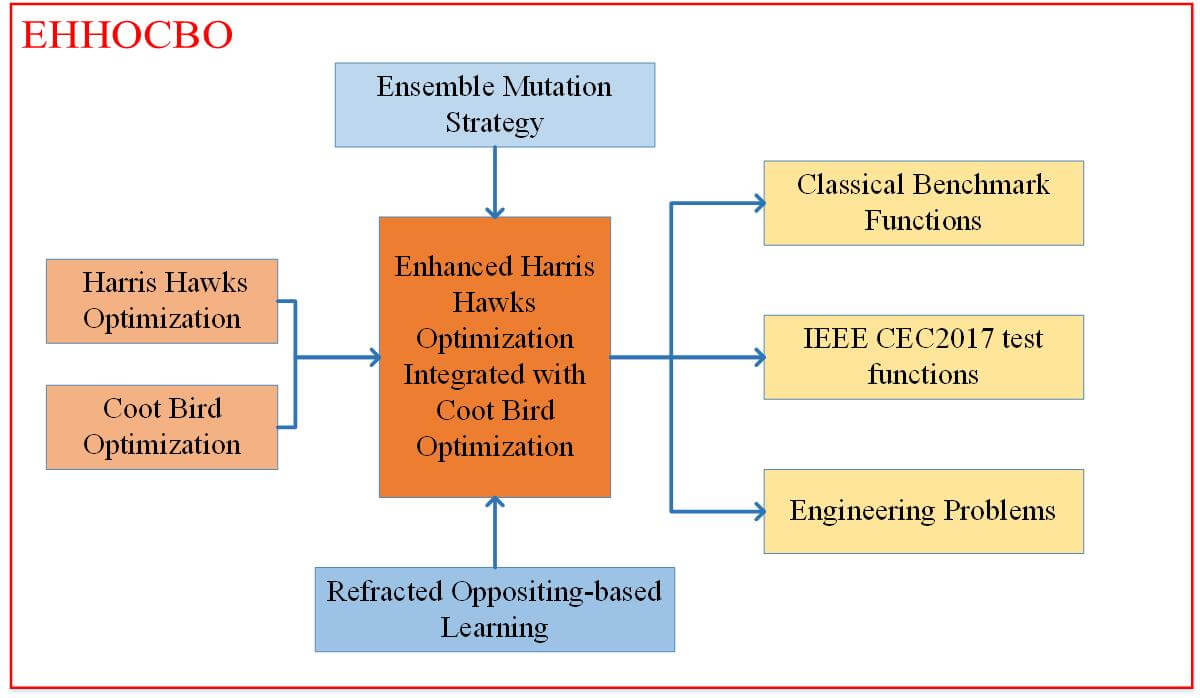
Keywords
Cite This Article
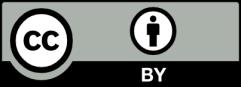
This work is licensed under a Creative Commons Attribution 4.0 International License , which permits unrestricted use, distribution, and reproduction in any medium, provided the original work is properly cited.