Open Access
ARTICLE
Multidimensional Quality Evaluation of Graduate Thesis: Based on the Probabilistic Linguistic MABAC Method
1
College of Management Science, Chengdu University of Technology, Chengdu, 610059, China
2
Post-Doctorate R&D Base of Management Science and Engineering, Chengdu University of Technology, Chengdu, 610059, China
3
Business School, Sichuan University, Chengdu, 610064, China
* Corresponding Authors: Xiaoxu Zhang. Email: ; Yong Qin. Email:
(This article belongs to the Special Issue: Linguistic Approaches for Multiple Criteria Decision Making and Applications)
Computer Modeling in Engineering & Sciences 2023, 137(2), 2049-2076. https://doi.org/10.32604/cmes.2023.025413
Received 11 July 2022; Accepted 10 February 2023; Issue published 26 June 2023
Abstract
Graduate education is the main way to train high-level innovative talents, the basic layout to cope with the global talent competition, and the important cornerstone for implementing the innovation-driven development strategy and building an innovation-driven country. Therefore, graduate education is of great remarkably to the development of national education. As an important manifestation of graduate education, the quality of a graduate thesis should receive more attention. It is conducive to promoting the quality of graduates by supervising and examining the quality of the graduate thesis. For this purpose, this work is based on text mining, expert interviews, and questionnaire surveys to obtain the factors influencing the quality of a graduate thesis first. Then, through three rounds of expert consultation, a multidimensional evaluation indicator system for the graduate thesis quality is built. Furthermore, probabilistic linguistic term sets (PLTSs) are utilized to obtain the initial evaluation information and apply the stepwise weight assessment ratio analysis method to determine the weights of attributes. In the ensuing step, the novel multi-attribute border approximation area comparison based on the PLTS method is established. Finally, the proposed method is employed in a case study concerning the quality evaluation of a graduate thesis and the effectiveness of this approach is further illustrated.Keywords
As the highest level of higher education in China, graduate education is an important way to cultivate innovative, high-quality talents. With the rapid development of China’s economy, the level of graduate education is also improving rapidly [1]. At present, to improve the quality of higher education comprehensively, the reform of domestic graduate training mechanism continues to deepen, and the writing and publication of a graduate thesis are closely related to the enhancement of graduate research ability, the cultivation of graduate quality, and the sustainable development of graduate education. Therefore, thesis quality is an important embodiment of the graduate education level. The quantitative data show that students who enter graduate education do so chiefly to improve employment prospects [2]. The lack of academic research spirit among graduates has led to a downward trend in the level of theses, a marker of the quality of graduate training. The most evident manifestation is that theses published by graduates suffer from insufficient innovation, insufficient theoretical depth, and weak writing ability. In addition, several problems are observed in topic selection, writing, and academic ethics in graduate theses, such as impractical topic selection and lack of standardization in thesis and academic misconduct [3]. These problems are highly detrimental to the development of a good environment for thesis quality. In response to a series of problems in practice, thesis quality has attracted wide attention from educational and theoretical circles, and several scholars have launched active discussions on thesis quality.
The bibliometric tool CiteSpace is utilized to select the core database of Web of Science to explore the overall research status of thesis quality with the theme “dissertation or thesis” and “quality or impact”. The time period is from 2000 to 2021, with one year as the time slice and keywords as the nodes, to generate the keyword co-occurrence map, and the results are shown in Fig. 1. The larger the node is, the more the occurrences of keywords. Fig. 1 shows that the research on the quality of the thesis is carried out in three main directions, namely, the direction of thesis quality evaluation represented by “quality,” the response to students’ education represented by “education”, and the effect of thesis quality produced by “impact”.
Figure 1: Keyword co-occurrence map of graduate thesis research
First, the data of the top 12 keywords are obtained by merging similar keywords, as shown in Table 1. The research centrality of “education,” “quality,” and “impact” is much higher than other aspects. The centrality of “simulation,” “webinar model,” and “growth” are all 0.13. Moreover, the centrality of other keywords is 0.1.
Then, the frontiers of research are analyzed. Burst term refers to the keyword with a sudden increase in frequency in a short period of time, and burst rate refers to the intensity of the sudden increase in the frequency of words in a short period of time. Burst term and burst rate are used to reflect the changing trend of the research frontier. The results of the analysis of emerging keywords in the literature generated by CiteSpace are shown in Table 2. The top 11 keywords with high intensity of appearance and strong disciplinary representation are given. From 2010 to 2021, the academic terms with high research popularity include “management,” “quality,” “education,” “impact,” “model,” “student,” and “higher education.” However, the research fields represented by “quality,” “student,” and “higher education” have been gaining attention since 2012 and remain research hot spots in this field until now.
The above researches show that thesis quality is closely integrated with students and higher education, which has certain research value and practical significance. Improving the quality of the graduate thesis is not only an important embodiment of graduate education but also an inevitable requirement of university development. Therefore, more attention should be paid to it. Besides, the quality of theses published by graduates is one of the common problems of degree-granting units in China. It is of great significance to explore the influencing factors and effective evaluation methods. The investigation and summary of factors affecting the quality of the graduate thesis can provide a reference for the analysis of the quality of the graduate thesis. Given the present situation of the thesis quality of college graduates, this paper discusses the influencing factors and the multidimensional evaluation index system of thesis quality, readers can understand what conditions a good thesis should have and what factors have a greater impact on its quality, so as to make efforts to effectively improve the quality of thesis. At the same time, in the actual evaluation, the evaluators may hesitate between several linguistic terms when they use those to evaluate each index of the target object, and their preference for the hesitant linguistic terms varies. Facing it, this paper tries to introduce the concept of probabilistic linguistic term sets to solve the problem that all possible linguistic terms are of equal importance, which can make the evaluation result more accurate. Therefore, this paper combines the SWARA method and PL-MABAC method to evaluate the quality of graduate theses. Readers can evaluate the quality of dissertations, and researchers can apply this evaluation method to other aspects, which is conducive to the improvement of research theories and the expansion of research dimensions. Moreover, this paper demonstrates the validity of the model by taking the quality of graduate theses from five universities in Sichuan Province as an example.
The rest of this paper is arranged as follows: Section 2 provides an in-depth analysis of related research. Section 3 briefly introduces the PLTSs and the MABAC methods. Section 4 discusses the realization of the proposed method according to the operation. Section 5 takes five universities as cases to demonstrate the use of this method and analyzes its scientific nature. Section 6 draws conclusions that clarify the contributions and limitations of this paper.
2.1 Study on Influencing Factors of Thesis Quality
Graduate students and supervisors directly affect the quality of the thesis. On the one hand, graduates are direct “surgeons.” The differences in the quality of graduates and their specialties make them spend different efforts and time in thesis writing; hence, the quality of the thesis is also different. For example, helping engineering graduates write their theses can be a difficult, time-consuming undertaking for a thesis advisor [4]. Graduates who properly manage their time, process, and energy are more likely to write research proposals, theses, and dissertations and become published [5]. On the other hand, a tutor is an important person within a student’s talent development [6], and their academic ethics, research level, and academic attitude directly affect the innovative research of a graduate thesis. What’s more, the relationship with the supervisor could influence the quality evaluation of the postgraduate theses [7], but will not on that about Ph.D. dissertations. In the field of graduate education, tutor feedback plays a critical role in students’ learning experiences and academic performance [8]. A good tutor’s feedback can play a better role in guidance. The personal ability and the quality of the tutor are also related to the level of graduates’ learning achievement [9]. However, in developing countries, the growth in the supervisory capacity of tutors has not kept pace with the growth in the number of students [10]. Several tutors have outdated personal knowledge, lax requirements for students, poor guidance, and inadequate responsibilities.
The factors that graduates are most concerned about include tutors, competency development, research climate, infrastructure, paper review, and clarity of mission [11]. Existing research has found five factors at three different levels that affect the quality of the thesis: one student factor (favorable characteristics for conducting research), three consultant factors (research experience, latest research knowledge, and ratio of consultants to students), and one institutional factor (close monitoring and management system) [12]. The content of graduate curricula and the opportunities for graduates to participate in research projects can also affect the quality of their theses. A high-quality thesis may also be caused by factors such as the language skills that students have already mastered at the time of admission or the complex interaction between tutor and student, and not just effective classroom design or curriculum settings [13]. Mastering academic writing is indispensable for successful performance in tertiary education [14]. Thesis writing is inseparable from citation, and citation is positively correlated with the quality of writing. After analyzing the citation types and trends of 157 master’s theses in 19 different disciplines from one university over 10 years, Swart [15] suggested that postgraduate students need to select citations from more reliable sources and format them correctly, which can help improve the quality of theses. By comparing the citation characteristics of master’s and doctoral dissertations of English majors in China, it can be found that doctoral students can cite more theories or models, which to some extent reflects the higher academic ability of doctoral students [16]. The choice of the topic and the connection of the content directly affect the quality of the thesis. A high-quality thesis should have an excellent literature review to elaborate on the research background and the motivation of the research. Therefore, scholars regard the quality of the literature review as the influencing factor of thesis quality [17]. The peer-review system is an important step to evaluate the quality of a graduate thesis, using a specially developed online peer-review system, the Peer Portal, which also has a certain degree of supervision for improving the quality of the graduate thesis and affecting the thesis quality [18].
The above research reveals that the problems in graduate theses are formed under the combined influence of many factors. The factors affecting the quality of graduate theses can be summarized in many aspects. At present, a single level of research on the influencing factors of thesis quality is noted. A multidimensional, all-around thesis quality evaluation system is lacking. Based on online and offline approaches, the factors affecting the quality of graduate theses are identified in this paper from multiple perspectives through text mining, interviews, and questionnaires. This paper takes graduates, tutors, university administrators, and recruitment units as research objects to determine the influencing factors of thesis quality and constructs the evaluation indicator system.
2.2 Study on Evaluation of Thesis Quality
The performance of a graduate thesis is related to the quality of graduate education and the outcomes of scientific research [16]. With the continuous reform of graduate education, the importance has gradually changed from the quantity of the thesis to quality. How to improve the quality of the thesis has become a hot topic at present. Studying the quality evaluation methods of the past articles is conducive to further analysis and exploration of the quality evaluation of the graduate thesis, which can provide extensive reference experience for graduate education management.
Thesis quality evaluation is a typical multifactor comprehensive evaluation system. Existing indicators for evaluating thesis quality mainly include peer review and citation frequency. When citation frequency becomes an indicator of the importance of scientific articles [19], it is used by many scholars in measuring the quality of articles [20–22]. In the evaluation of citations, multiple indicators such as article impact factors, position impact factors, and journal impact factors are proposed. Using multiple indicators for evaluation, especially considering different weights, should be given to citations in different positions of the article [23]. Moreover, the criteria and focus of evaluation are different according to specialty. For the master of public health (MPH), a specific description of the methods and results is valued in the dissertations [24]. For translation studies, examiners put more emphasis on the accuracy of language and the presentation of ideas in master’s dissertations [25]. How to ensure the accuracy of the evaluation effectively needs further discussion and research. The existing research is limited by the data sources, which makes the research unconvincing. The evaluation of the quality of the graduate thesis should not be confined to a single thesis but should be carried out from the perspective of the whole university. Nevertheless, the overall evaluation of the thesis situation under the graduate education system is lacking. To determine the differences between the quality of thesis in universities’ undergraduate education, universities must be set as alternatives using the constructed evaluation indicator system; thus, this constitutes multiple attribute decision-making (MADM) problems. MADM is an important research field in decision science and operations research [26]. Further theoretical studies on MADM problems with qualitative information have been ongoing all the time in recent years [27]. They increasingly find that linguistic information is better at coordinating the human mind in terms of expressing preference information as well as conveying ambiguous information than numerical values. With the application of a linguistic term set (LTS), the probabilistic linguistic term set (PLTS) is proposed [28]. PLTS is an up-to-date tool for dealing with uncertain information in decision-making [29]. Many scholars have applied PLTSs to the MADM problem. PLTSs are integrated with the ORESTE method to identify preference relations, indifference relations, and incomparability among alternatives [30]. Water security is evaluated based on the TODIM method with PLTSs [31]. PLTSs are also combined with other MADM methods to explore more scientific decision processes and outcomes.
The existing research on graduate thesis quality evaluation inspires us to establish an evaluation index system from different stakeholder perspectives to better evaluate the quality of the graduate thesis. And that is where we lay the originality of this paper. On the one hand, traditional research from a certain single point of view on the quality of graduate thesis factors to explore, few at the same time combine multiple perspectives to study, and few people pay attention to the enterprise recruitment unit on the quality of the graduate thesis. On the other hand, most of the existing evaluation methods ignore the influence of the ambiguity and hesitancy of experts’ scores on the evaluation results. Related studies combined with PLTS are very scarce, which is worthy of further study. Therefore, it is very important to study the influencing factors of thesis quality from the overall perspective, combine multi-source data, and build the thesis quality evaluation model which is helpful to eliminate the influence of experts’ hesitation, to improve the correctness of the thesis quality evaluation results of graduate students, and to provide a feasible evaluation method for universities.
Given the complexity of decision-making problems in the real life, decision-makers (DMs) often hesitate among several possible linguistic terms when expressing their preferences in using linguistic information for decision-making [29]. Therefore, the PLTSs, which consist of several possible linguistic terms and their relative probabilities, are proposed [32]. As a flexible, efficient tool, PLTSs can handle the probabilistic distribution of linguistic assessments well and help experts express complex cognitive information, which is difficult to define or express in precise language. As an extended tool, it can simultaneously consider several linguistic terms with different weights (represented by probabilities), which can help DMs describe objects in terms of linguistic expression and probability [33]. Several concepts and operations related to PLTSs are as follows:
Definition 1 [28] Let
where
Definition 2 [28] Given a PLTS
where
Definition 3 [28] Let
Normalization can be conducted in two steps:
(1) If
(2) If
After standardizing the PLTSs, they need to be compared with one another to conduct subsequent rankings. Then, the score of each PLTS can be obtained.
Definition 4 [28] Let
where
For two PLTSs,
Definition 5 [28] Let
For two PLTSs,
The MABAC method was introduced by Pamucar and Cirovic in 2015 [34]. Initially, this method was utilized to research the problem of selecting transport and distributing resources in logistic centers [35]. The basic assumption in this method is to divide the alternatives into the border, upper, and lower approximation regions, and define the distance of the alternatives from the border approximation area. Each alternative is evaluated and ranked by specifying the difference between the distances. The MABAC method has the advantages that the computation is simple, the results are stable, and a comprehensive result can be obtained considering the potential amount of gain or loss [36], which is a helpful, reliable tool for MADM [37]. Currently, this method is utilized in MADM problems.
First, the MABAC method utilizes a compromise normalization equation to compute the positive and the negative attributes to form a matrix
To solve the problem of graduate thesis quality evaluation, a multidimensional evaluation indicator system for graduate theses is constructed, and then the attribute weights are calculated using the SWARA method in this section. Finally, the PL-MABAC method is introduced to rank the quality of the graduate theses. The proposed framework is shown in Fig. 2.
Figure 2: Framework of graduate thesis quality evaluation
4.1 Evaluation Indicator Analysis
In this section, the information data generated by text mining, expert interviews, and questionnaire surveys are utilized to study the relative influencing factors of the research object. By collating and analyzing influencing factors, the underlying information can be obtained to establish the indicator system, and the decision-making method is applied to make a rigorous process and gain accurate results.
4.1.1 Acquisition and Pretreatment of Influencing Factors
Evaluating the quality of graduate theses is conducive to optimizing the thesis quality management system and management process, discovering the deficiencies in university education in time, promoting the benign development of academic research, creating an effective research environment, and strengthening the cultivation of high-quality talents. Most of the existing factors affecting the quality of graduate thesis focus on three aspects, namely, students, tutors, and universities, but the factors affecting thesis quality are multifaceted, including structural and nonstructural factors. A comprehensive study of these factors is of great relevance to improving the comprehensive quality of students and the influence of scientific research in universities.
Data on factors affecting thesis quality are obtained through online and offline channels. Online text mining methods include the use of small wood insects to obtain education review texts and literature searches based on thesis quality. Offline methods mainly utilize semi-structured interviews and questionnaires to collect opinions and information from relevant experts to help construct a reasonable evaluation indicator system. We sent questionnaires to four main types of experts: the graduate students who have graduated who are very familiar with the writing work of the dissertation, the supervisors who direct the work of the graduate thesis who understand the problems often encountered in the writing process of the graduate thesis and what should be paid attention to, the university management personnel responsible for the evaluation of the graduate thesis who comprehend the review process and basic requirements of the graduate thesis, and the personnel responsible for the recruitment of the company who are clear about the abilities and conditions they need to recruit graduate students.
Through the previous research and analysis, this work obtains 68 influencing factors, which can be divided into four dimensions according to their correlation: graduates, tutors, university administrators, and recruitment units.
4.1.2 Establishing the Indicator System
Based on the collected data and information, combined with the expert evaluation method, experts’ professional knowledge and experience are reviewed and utilized in this paper to determine the relationship between the influencing factors and score them according to the degree of influence. The higher the score is, the higher the influence. According to the above influencing factors, three expert interviews are conducted, and we keep deleting and merging the influencing factors. Finally, a thesis quality evaluation indicator system including 13 attributes is obtained, as summarized in Table 3. Graduates, tutors, university administrators, and recruitment units are taken as the survey objects, and questionnaires and interviews are conducted to collect relevant data. Moreover, the evaluation indicator related to the quality of theses is evaluated by reading graduate theses of relevant universities.
The evaluation indicator system constructed in this paper includes multiple perspectives of graduates, tutors, university administrators, and recruitment units, which is conducive to a multi-dimensional analysis of the objective and subjective factors affecting the graduate dissertation. At the same time, the paper is based on the actual situation, pays attention to the key factors affecting the quality of graduate papers, focus on breaking through the bottleneck, and solves the existing problems, which is conducive to improving the level of graduate papers on the whole.
4.2 PL-MABAC Method Based on the SWARA Weight Method
In this section, the concrete process of implementing the PL-MABAC method based on the SWARA weight method to solve the problem of MADM is put forward. The method consists of two parts: (1) calculating the weight of attribute based on the SWARA method and (2) determining the final ranking based on the PL-MABAC method. The detailed flowchart is shown in Fig. 3.
Figure 3: Flowchart of the proposed PL-MABAC method
4.2.1 Calculating the Weight of Attribute Based on the SWARA Method
Determining attribute weights is a basic step in MADM. Different attribute weight values will lead to different decision results. The process of attribute prioritization and weighting is an important part of MADM, and it affects the final decision result. Therefore, it is very important to obtain attribute weights correctly. The SWARA method is utilized to calculate attribute weights. The SWARA method was introduced by Kersuliene et al. in 2010 [38]. In this method, done by the weighting method, the relative importance and the initial priority of each attribute are determined by the opinion of the DMs, and then the relative weight of each attribute is determined by calculation. This method enables DMs to express their opinions freely because they are not defined by fixed measures or scales [39]. The strength of the SWARA method is simpler and more straightforward to operate compared with other weighting methods [40,41].
The attributes are prioritized in terms of relative importance, determined by DMs. The modified SWARA method [42] is used to integrate the results from DMs by introducing the geometric average method. First, each DM prioritizes attributes based on their importance. The most important attribute is assigned a value of 1.00, and other attributes are given in descending order according to their relative importance as a multiple of 0.05. In the attribute decision method, the mean value of the attribute
Integrating the evaluation information of all DMs, the combined relative importance score of each attribute is determined as follows:
Then, starting from the second attribute, the relative importance of the attribute
The coefficient (
At this stage,
Hence, the final attribute weights are calculated as
The above are all the steps in this paper to calculate the weight
4.2.2 Determining the Final Ranking Based on the PL-MABAC Method
Similar to the classical MABAC method, the initial decision matrix must be obtained and normalized. First, PLTS is utilized to build the initial decision matrix. PLTS has been proposed to deal with the probabilistic distribution in providing linguistic assessments [43]. The PL-MABAC method is proposed in this work to explore the ranking of alternatives. The LTS
Considering the MADM problem with probabilistic linguistic information, n alternatives
where
To rank the alternatives, the initial matrix, which should have specific operator values to facilitate the computation and solution, needs to be obtained. In previous studies, people always utilized the score of PLTS to construct the decision matrix, but in PLTS comparison, when the scores are the same, further deviation degree needs to be compared. Therefore, the deviation degree is directly utilized to construct the decision matrix to make the results more accurate. Eqs. (3) and (4) are applied to calculate the deviation degree of
The initial decision matrix generated by PLTS can be directly applied to the rank calculation. Therefore, the generation of a matrix
Above all, the positive and negative attributes of the decision matrix are normalized by Eqs. (13) and (14), respectively.
The new matrix
The border approximation area for each attribute is obtained according to Eq. (16).
By determining the values of the border approximation area matrix, the border approximation area matrix
With respect to the elements in the weighted normalized values and the border approximation area matrix of each attribute, the distance of the alternatives from the border approximation area is determined by Eq. (17).
A calculation of the values of the attribute functions for the alternatives is determined as the sum of the distance of the alternatives from the border approximation areas. The total distances of each alternative from the border approximate area are determined by Eq. (18).
The total distance between the alternatives and the border approximate area in descending order is determined, and the final ranking of all alternatives is obtained based on this.
In summary, an algorithm for the whole decision making is presented as follows:
Input: Probabilistic linguistic decision matrix
Output: Ranking of alternatives
Step 1: Collect relevant information and construct the probabilistic linguistic decision matrix.
Step 2: Normalize the probabilistic linguistic decision matrix by Definitions 3 and 4.
Step 3: Construct the initial decision matrix
Step 4: Calculate the ranking order of attributes according to DMs.
Step 5: Determine alternative weights
Step 6: Normalize the initial decision matrix and form the decision matrix
Step 7: Obtain the border approximation area matrix
Step 8: Determine the total distances from the border approximate area
Step 9: Rank the overall alternatives
Step 10: End.
A case study on the ranking of graduate thesis quality of five universities in Sichuan Province, China through the proposed method, which can illustrate the use of the PL-MABAC method, is presented in this section. Then, its validity and feasibility in actual cases and comparative analysis can be proven.
5.1 Application of the Proposed Methodology
In recent years, university construction has become an important part of national education development. In China, the reform of the graduate cultivation mechanism is being promoted deeply, and many universities are strengthening quality education. To reflect the quality of current graduate education in universities, we can start from the most intuitive aspect and judge the graduate education of universities through the evaluation of the quality of the graduate thesis. In this paper, five universities in Sichuan Province are selected to illustrate the proposed approach.
They are the University of Electronic Science and Technology of China (
Through questionnaire surveys and interviews, the opinions of graduates, tutors, university administrators, and recruitment units are sought to provide them with relevant reference materials and a linguistic evaluation scale. The following LTS is utilized:
By collecting relevant questionnaires, all the judgments can be obtained. For example, when evaluating the degree of recognition (O1) of alternative A1, 10.6% of the interviewees consider that the attribute O1 of A1 is “a little good (s1),”, 42.4% of the interviewees consider that the attribute O1 of A1 is “good (s2),” and 47.0% of the interviewees think that the attribute O1 of A1 is “very good (s3).” Therefore, the evaluation value of O1 in A1 is obtained, denoted as
Utilizing Eq. (3), the scores of the PLTSs are calculated. The deviation degree of the PLTSs can be obtained by Eq. (4).
Then,
The SWARA method is used to obtain the weights of attributes. Thus, the individual evaluations of five DMs are utilized to obtain the ranking order of attributes, and Eq. (5) is utilized to merge relative importance scores calculated for each criterion. The results are given in Table 7.
Attributes are ranked in descending order according to merged relative importance scores. Table 8 provides the initial prioritization, coefficient, initial weight, and final weight
Then, the attribute weights are obtained as
After determining the weight of each attribute, the universities are ranked according to the quality of their graduate thesis. Eqs. (13) and (14) are utilized to normalize the initial decision matrix
Furthermore, the weights of the attributes that are obtained by the SWARA method are utilized to obtain the weighted normalized values in Table 9 by Eq. (15). Utilizing the values in Table 9, the border approximation area matrix
Based on Tables 9 and 10, the distance of the alternatives from the border approximation area
In terms of the calculation results of
5.2.1 Comparison with Actual Situations
To validate the reliability of the PL-MABAC method, the actual development of universities is compared with the results obtained from our method. Whether the overall level of graduate thesis quality obtained from our method is consistent with the actual development of the universities can be checked. The actual situation of graduate thesis quality is a relatively complex problem, and no accurate evaluation method for determining the level of graduate thesis exists to date. Although the quality of the graduate thesis and the slight differences among graduate theses cannot be accurately determined, the overall quality in different universities can be ranked based on the related indicators of the quality of the graduate thesis. For the case study, five universities are selected, such that comparing one another directly is relatively easy, and through consulting experts, the preference order of 13 attributes is obtained, which helps complete the calculation of the decision method. The final ranking results agree with China’s university grades and rankings. The better the university is, the higher the quality of the thesis, reflecting the importance of strengthening the overall education of the university. Clearly, the ranking results obtained based on our method are relatively consistent with the actual development of colleges and universities; hence, our method is reasonable.
5.2.2 Comparison with Other Ranking Methods
To verify the effectiveness of the PL-MABAC method, a comparative analysis is carried out. The two other methods are utilized to obtain the ranking of thesis quality. They are the Technique for Order Preference by Similarity to an Ideal Solution based on the PLTS (PL-TOPSIS) method and the Additive Ratio Assessment based on the PLTS (PL-ARAS) method. The TOPSIS method [45] is one of the most well-known methods in MADM problems. The classical TOPSIS method employs a similarity index to rank alternatives [46]. It was first proposed by Hwang and Yoon in 1981, and it is suitable for the ranking of limited alternatives with multiple indicators or attributes. Its central idea is to hope that the object to be evaluated is as close to the positive ideal solution as possible and as far as the possible negative ideal solution. As a kind of commonly utilized effective MADM method, it can fully reflect the differences among alternatives and has no special requirement for sample data. Therefore, it has been utilized widely in practical applications. The ARAS method [47], which aims to select the best alternative based on several attributes, was introduced by Zavadskas and Turskis in 2010, and the final ranking of alternatives is made by determining the utility degree of each alternative.
First, considering the results of Table 6 and the attribute weighting vector
Then, considering the results of the initial decision matrix
Table 15 shows that the ranking results obtained by the three methods are roughly the same. The university with the highest score is the University of Electronic Science and Technology of China (
(1) Considering the expert psychological factors that affect the result of the decision, this paper uses the subjective weighting method which gives full consideration to the decision makers of experience, professional knowledge, and mastery of specific problems. At the same time, the paper applies MABAC to an uncertain probabilistic linguistic environment and quantifies the qualitative index to make policymakers’ evaluation more accurate results and decision-making information more comprehensive. Therefore, the method used in this paper can not only consider the completion of unknown probabilistic information but also integrate the psychological factors of experts, while the TOPSIS method is based on the strict assumption of complete rationality of experts, ignoring the subjective influence of experts.
(2) Although PL-MABAC and PL-ARAS have the same ranking order, alternatives in PL-ARAS are evaluated based on the utility of each attribute, which is different from PL-MABAC. PL-MABAC ranks alternatives according to the distance from the boundary approximation region, which can be easy to understand and operate.
The above analysis shows that the model established in this paper has good applicability both in theory and in experimental data, and verifies the effectiveness and practicability of the model.
In this paper, a new multidimensional ranking method for evaluating the quality of graduate theses is explored, and an evaluation ranking based on the SWARA weighting method and the PL-MABAC decision method is proposed. In the operation, text mining, expert interviews, and questionnaire surveys are utilized to acquire information and data. Thus, the four perspectives of graduates, tutors, university administrators, and recruitment units are used to build a more comprehensive, reasonable multi-attribute evaluation indicator system for graduate thesis quality. Based on the obtained data, the SWARA method is introduced to obtain the attribute weights. The MABAC method is combined with PLTSs to construct the PL-MABAC method. The overall situation of the quality of graduate thesis in five universities is explored, and the results show that: the quality of master dissertations in
Furthermore, the PL-MABAC method with the PL-TOPSIS method and the PL-ARAS method are compared and analyzed to show that the results obtained by utilizing the method proposed are in line with the actual situation and more scientific and reliable. Based on the above analysis, the contributions of this paper are as follows:
(1) This paper refers to the perspective of multiple stakeholders. Our study perspectives include graduates, tutors, university administrators, and recruitment units. The perspectives can better reflect the overall results of the quality of the graduate thesis, and also help these stakeholders to take measures to improve the quality of the thesis effectively.
(2) Combining the methods of text mining, expert interview, and questionnaire survey, multidimensional data are used to obtain the influencing factors of graduate thesis quality. Therefore, a more complete indicator system for evaluating the quality of the graduate thesis can be built, laying the foundation for ensuring the scientific nature of the evaluation method.
(3) This paper evaluates the quality of theses based on PLTS and optimizes the construction method of the PLTS evaluation matrix based on linguistic information, which can effectively overcome ambiguity and uncertainty in the decision-making process and enhance the reliability and validity of decision results.
(4) This paper provides decision-making methods and directions for the quality evaluation of graduate theses and improves the shortcomings of traditional evaluation methods. By selecting graduate theses from five universities in Sichuan Province and conducting quality evaluation case analysis, the decision-making process of PL-MABAC is introduced in detail. Through comparison and analysis with other methods, it is found that the method proposed in this paper can not only use fuzzy information to express the opinions of graduate thesis quality evaluation, but also take into account the influence of subjective factors in expert scoring. This case illustrates the validity and reliability of the method in this paper. What’s more, PL-MABAC has clear logic and easy operation, which can be extended to other industries for evaluation and optimization.
The limitations of this paper are as follows:
(1) The final evaluation indicator system constructed using text mining, expert interviews, and questionnaires is affected by the accuracy of the data and is prone to bias in its construction.
(2) When using the modified SWARA method to calculate the weights, the scoring of experts is incorporated, which will make the weighting results affected by the subjective ideas of experts.
(3) The PL-MABAC method proposed in this paper is effective. Nonetheless, given that the method is a newly proposed combination method, it still has room for optimization in many aspects. It can be applied in more areas and extended in more aspects, which are not shown much in this paper.
In future research, we will continue to optimize the PL-MABAC method to make it easier to operate and more efficient and reliable. Although this paper adopts thesis quality as a case study, the method can be tried in more areas.
Funding Statement: This work was supported by the Humanities and Social Sciences Program of the Ministry of Education (Grant No. 20YJC630095), the National Natural Science Foundation of China (Grant Nos. 71501019, 71971151), Postdoctoral Research Foundation of China (Grant No. 2018M631069), Soft Science Research Program of Chengdu (Grant No. 2017-RK00-00425-ZF), General Project of Regional Public Management Informatization Research Center of Key Social Science Research Base in Sichuan (Grant No. QGXH20-03), the Funding Program for Middle Aged Core Teachers at Chengdu University of Technology (Grant No. 2019KY3704203), Philosophy and Social Science Research Foundation of Chengdu University of Technology (Grant No. YJ2019-NS004) and the Special Funding for Post-Doctoral Research Projects of Sichuan in 2017 Named “Dynamic Evolution of Multi-System Coupling in Resource-Oriented Cities of Western China from a Technology Innovation-Driven Perspective” and the Program of Graduate Education Reform Program at Chengdu University of Technology (Grant No. 10800-00009824), Key Project of National Park Research Center of Sichuan Social Science Research Base (Grant No. GJGY2020-ZD001) “Research on the Path to Improve Tourism Ecological Value of Giant Panda National Park from the Perspective of Host-Guest Governance”.
Conflicts of Interest: The authors declare that they have no conflicts of interest to report regarding the present study.
References
1. Zhao, Q. D., Huang, Y. H., Liu, Y. S. (2020). Spatiotemporal evolution pattern and driving factors of higher-education population in China. Complexity, 2020, 1–11. https://doi.org/10.1155/2020/1927364 [Google Scholar] [CrossRef]
2. Liu, D., Morgan, W. J. (2020). Why do students enrol for postgraduate education in China? The influence of gender and of family habitus. Gender and Education, 32(2), 177–193. https://doi.org/10.1080/09540253.2018.1447092 [Google Scholar] [CrossRef]
3. Ali, I., Aboelmaged, M. (2021). A bibliometric analysis of academic misconduct research in higher education: Current status and future research opportunities. Accountability in Research, 28(6), 372–393. https://doi.org/10.1080/08989621.2020.1836620 [Google Scholar] [PubMed] [CrossRef]
4. Tanaka, M. L. (2020). A thesis proposal development course for engineering graduate students. Journal of Biomechanical Engineering, 142(11), 114704. https://doi.org/10.1115/1.4047925 [Google Scholar] [PubMed] [CrossRef]
5. Gump, S. E. (2020). The productive graduate student writer: How to manage your time, process, and energy to write your research proposal, thesis, and dissertation and get published. Journal of Scholarly Publishing, 51(3), 216–223. https://doi.org/10.3138/jsp.51.3.05 [Google Scholar] [CrossRef]
6. Margot, K. C., Kettler, T. (2019). Teachers’ perception of STEM integration and education: A systematic literature review. International Journal of Stem Education, 6(1), 2. https://doi.org/10.1186/s40594-018-0151-2 [Google Scholar] [CrossRef]
7. Wagener, B. (2018). The importance of affects, self-regulation and relationships in the writing of a master’s thesis. Teaching in Higher Education, 23(2), 227–242. https://doi.org/10.1080/13562517.2017.1379480 [Google Scholar] [CrossRef]
8. Li, F. F., Curdt-Christiansen, X. L. (2020). Teacher feedback in UK higher education: Affective and cognitive perceptions of Chinese postgraduate students. International Journal of Educational Research, 104(6), 101674. https://doi.org/10.1016/j.ijer.2020.101674 [Google Scholar] [CrossRef]
9. Darling-Hammond, L. (2000). Teacher quality and student achievement: A review of state policy evidence. Education Policy Analysis Archives, 8(1). https://doi.org/10.14507/epaa.v8n1.2000 [Google Scholar] [CrossRef]
10. Renaud, K., Van Biljon, J. (2017). The role of knowledge visualization in supporting postgraduate dissertation assessment. British Journal of Educational Technology, 48(6), 1475–1490. https://doi.org/10.1111/bjet.12494 [Google Scholar] [CrossRef]
11. Marsh, H. W., Martin, R. A. (2002). PhD students’ evaluations of research supervision: Issues, complexities, and challenges in a nationwide Australian experiment in benchmarking universities. The Journal of Higher Education, 73(3), 313–348. https://doi.org/10.1080/00221546.2002.11777151 [Google Scholar] [CrossRef]
12. Laosum, T., Kanjanawasee, S. (2016). Development of a dissertation quality value-added model for humanities and social sciences programs for private higher education institutions in Thailand. Kasetsart Journal of Social Sciences, 37(3), 138–143. https://doi.org/10.1016/j.kjss.2016.08.010 [Google Scholar] [CrossRef]
13. Hamilton, P., Johnson, R., Poudrier, C. (2010). Measuring educational quality by appraising theses and dissertations: Pitfalls and remedies. Teaching in Higher Education, 15(5), 567–577. https://doi.org/10.1080/13562517.2010.491905 [Google Scholar] [CrossRef]
14. Dontcheva-Navratilova, O., Jancarikova, R., Hulkova, I., Schmied, J. (2020). Theme choices in Czech University students’ English-medium master’s theses. Lingua, 243(2), 102892. https://doi.org/10.1016/j.lingua.2020.102892 [Google Scholar] [CrossRef]
15. Swart, A. J. (2019). Citation analysis of master dissertations at the central university of technology, South Africa. African Journal of Library Archives and Information Science, 29(2), 115–129. [Google Scholar]
16. Li, Q. C., Zhang, X. (2021). An analysis of citations in Chinese English-major master’s theses and doctoral dissertations. Journal of English for Academic Purposes, 51(1), 100982. https://doi.org/10.1016/j.jeap.2021.100982 [Google Scholar] [CrossRef]
17. Xie, Z., Li, Y. W., Li, Z. M. (2020). Assessing and predicting the quality of research master’s theses: An application of scientometrics. Scientometrics, 124(2), 953–972. https://doi.org/10.1007/s11192-020-03489-3 [Google Scholar] [CrossRef]
18. Aghaee, N., Hansson, H. (2013). Peer portal: Quality enhancement in thesis writing using self-managed peer review on a mass scale. International Review of Research in Open and Distributed Learning, 14(1), 186–203. https://doi.org/10.19173/irrodl.v14i1.1394 [Google Scholar] [CrossRef]
19. Virgo, J. A. (1977). A statistical procedure for evaluating the importance of scientific papers. Library Quarterly Information Community Policy, 47(4), 415–430. https://doi.org/10.1086/620723 [Google Scholar] [CrossRef]
20. Eshraghi, A., Osman, N., Gholizadeh, H., Ali, S., Shadgan, B. (2013). 100 top-cited scientific papers in limb prosthetics. Biomedical Engineering OnLine, 12(1), 119. https://doi.org/10.1186/1475-925X-12-119 [Google Scholar] [PubMed] [CrossRef]
21. Faruk, T. (2014). An analysis of the most-cited research papers on oncology: Which journals have they been published in? Tumor Biology, 35(5), 4645–4649. https://doi.org/10.1007/s13277-014-1608-7 [Google Scholar] [PubMed] [CrossRef]
22. Yamashita, Y., Yoshinaga, D. (2014). Influence of researchers’ international mobilities on publication: A comparison of highly cited and uncited papers. Scientometrics, 101(2), 1475–1489. https://doi.org/10.1007/s11192-014-1384-6 [Google Scholar] [CrossRef]
23. Sombatsompop, N., Kositchaiyong, A., Markpin, T., Inrit, S. (2006). Scientific evaluations of citation quality of international research articles in the SCI database: Thailand case study. Scientometrics, 66(3), 521–535. https://doi.org/10.1007/s11192-006-0038-8 [Google Scholar] [CrossRef]
24. Dai, S. Y., Zhou, X. B., Xu, H., Li, B. B., Zhang, J. G. (2020). Evaluation of the reporting quality of observational studies in master of public health dissertations in China. BMC Medical Research Methodology, 20(1), 230. https://doi.org/10.1186/s12874-020-01116-6 [Google Scholar] [PubMed] [CrossRef]
25. Man, D. L., Xu, Y. Q., Chau, M. H., O’Toole, J. M., Shunmugam, K. (2020). Assessment feedback in examiner reports on master’s dissertations in translation studies. Studies in Educational Evaluation, 64(6), 100823. https://doi.org/10.1016/j.stueduc.2019.100823 [Google Scholar] [CrossRef]
26. Lewis, M., Kochenberger, G. (2013). Graph bisection modeled as cardinality constrained binary quadratic task allocation. International Journal of Information Technology & Decision Making, 12(2), 261–276. https://doi.org/10.1142/S0219622013500119 [Google Scholar] [CrossRef]
27. Feng, X. Q., Zhang, Q., Jin, L. S. (2020). Aggregation of pragmatic operators to support probabilistic linguistic multi-criteria group decision-making problems. Soft Computing, 24(10), 7735–7755. https://doi.org/10.1007/s00500-019-04393-6 [Google Scholar] [CrossRef]
28. Pang, Q., Wang, H., Xu, Z. (2016). Probabilistic linguistic term sets in multi-attribute group decision making. Information Sciences, 369, 128–143. https://doi.org/10.1016/j.ins.2016.06.021 [Google Scholar] [CrossRef]
29. Xie, W., Xu, Z., Ren, Z., Wang, H. (2018). Probabilistic linguistic analytic hierarchy process and its application on the performance assessment of Xiongan New Area. International Journal of Information Technology & Decision Making, 17(6), 1693–1724. https://doi.org/10.1142/S0219622017500420 [Google Scholar] [CrossRef]
30. Wu, X. L., Liao, H. C. (2018). An approach to quality function deployment based on probabilistic linguistic term sets and ORESTE method for multi-expert multi-criteria decision making. Information Fusion, 43(1), 13–26. https://doi.org/10.1016/j.inffus.2017.11.008 [Google Scholar] [CrossRef]
31. Zhang, Y. X., Xu, Z. S., Liao, H. C. (2019). Water security evaluation based on the TODIM method with probabilistic linguistic term sets. Soft Computing, 23(15), 6215–6230. https://doi.org/10.1007/s00500-018-3276-9 [Google Scholar] [CrossRef]
32. Liu, P., Li, Y., Teng, F. (2019). Bidirectional projection method for probabilistic linguistic multi-criteria group decision-making based on power average operator. International Journal of Fuzzy Systems, 21(8), 2340–2353. https://doi.org/10.1007/s40815-019-00705-y [Google Scholar] [CrossRef]
33. Luo, Y., Zhang, X., Qin, Y., Yang, Z., Liang, Y. (2021). Tourism attraction selection with sentiment analysis of online reviews based on probabilistic linguistic term sets and the IDOCRIW-COCOSO model. International Journal of Fuzzy Systems, 23(1), 295–308. https://doi.org/10.1007/s40815-020-00969-9 [Google Scholar] [CrossRef]
34. Pamucar, D., Cirovic, G. (2015). The selection of transport and handling resources in logistics centers using multi-attributive border approximation area comparison (MABAC). Expert Systems with Applications, 42(6), 3016–3028. https://doi.org/10.1016/j.eswa.2014.11.057 [Google Scholar] [CrossRef]
35. Zhang, S., Wei, G., Alsaadi, F. E., Hayat, T., Wei, C. et al. (2020). MABAC method for multiple attribute group decision making under picture 2-tuple linguistic environment. Soft Computing, 24(8), 5819–5829. https://doi.org/10.1007/s00500-019-04364-x [Google Scholar] [CrossRef]
36. Liu, R., Hou, L. X., Liu, H. C., Lin, W. (2020). Occupational health and safety risk assessment using an integrated SWARA-MABAC model under bipolar fuzzy environment. Computational & Applied Mathematics, 39(4), 276. https://doi.org/10.1007/s40314-020-01311-7 [Google Scholar] [CrossRef]
37. Liu, P. D., Wang, D. Y. (2022). A 2-dimensional uncertain linguistic MABAC method for multiattribute group decision-making problems. Complex & Intelligent Systems, 8(1), 349–360. https://doi.org/10.1007/s40747-021-00372-3 [Google Scholar] [CrossRef]
38. Kersuliene, V., Zavadskas, E. K. (2010). Selection of rational dispute resolution method by applying new step-wise weight assessment ratio analysis (SWARA). Journal of Business Economics and Management, 11(2), 243–258. https://doi.org/10.3846/jbem.2010.12 [Google Scholar] [CrossRef]
39. Zolfani, S. H., Yazdani, M., Zavadskas, E. K. (2018). An extended stepwise weight assessment ratio analysis (SWARA) method for improving criteria prioritization process. Soft Computing, 22(22), 7399–7405. https://doi.org/10.1007/s00500-018-3092-2 [Google Scholar] [CrossRef]
40. Duan, C. Y., Liu, H. C., Zhang, L. J., Shi, H. (2019). An extended alternative queuing method with linguistic Z-numbers and its application for green supplier selection and order allocation. International Journal of Fuzzy Systems, 21(8), 2510–2523. https://doi.org/10.1007/s40815-019-00717-8 [Google Scholar] [CrossRef]
41. Ghenai, C., Albawab, M., Bettayeb, M. (2020). Sustainability indicators for renewable energy systems using multi-criteria decision-making model and extended SWARA/ARAS hybrid method. Renewable Energy, 146(2), 580–597. https://doi.org/10.1016/j.renene.2019.06.157 [Google Scholar] [CrossRef]
42. Bac, U. (2020). An integrated SWARA-WASPAS group decision making framework to evaluate smart card systems for public transportation. Mathematics, 8(10), 1723. https://doi.org/10.3390/math8101723 [Google Scholar] [CrossRef]
43. Jin, C., Wang, H., Xu, Z. (2019). Uncertain probabilistic linguistic term sets in group decision making. International Journal of Fuzzy Systems, 21(4), 1241–1258. https://doi.org/10.1007/s40815-019-00619-9 [Google Scholar] [CrossRef]
44. Xu, Z. S. (2005). Deviation measures of linguistic preference relations in group decision making. Omega-International Journal of Management Science, 33(3), 249–254. https://doi.org/10.1016/j.omega.2004.04.008 [Google Scholar] [CrossRef]
45. Hwang, C. L., Yoon, K. (1981). Multiple attribute decision making: Methods and applications. Berlin: Springer. [Google Scholar]
46. Sadabadi, S. A., Hadi-Vencheh, A., Jamshidi, A., Jalali, M. (2020). A new index for TOPSIS based on relative distance to best and worst points. International Journal of Information Technology & Decision Making, 19(3), 695–719. https://doi.org/10.1142/S0219622020500145 [Google Scholar] [CrossRef]
47. Zavadskas, E. K., Turskis, Z. (2010). A new additive ratio assessment (ARAS) method in multicriteria decision-making. Ukio Technologinis ir Ekonominis Vystymas, 16(2), 159–172. [Google Scholar]
Cite This Article
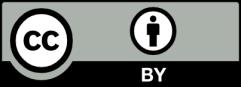
This work is licensed under a Creative Commons Attribution 4.0 International License , which permits unrestricted use, distribution, and reproduction in any medium, provided the original work is properly cited.