Open Access
EDITORIAL
Bio-Inspired Optimization in Engineering and Sciences
1
School of Computing and Mathematical Sciences, University of Leicester, Leicester, LE1 7RH, UK
2
College of Computer Science and Artificial Intelligence, Wenzhou University, Wenzhou, 325035, China
* Corresponding Author: Yudong Zhang. Email:
(This article belongs to the Special Issue: Bio-inspired Optimization in Engineering and Sciences)
Computer Modeling in Engineering & Sciences 2023, 137(2), 1065-1067. https://doi.org/10.32604/cmes.2023.029710
Received 04 March 2023; Accepted 06 March 2023; Issue published 26 June 2023
Abstract
This article has no abstract.Bio-inspired optimization algorithms [1,2] are a set of optimization algorithms inspired by natural phenomena, such as evolutionary processes, social behaviours, and swarm intelligence [3]. These algorithms attempt to simulate these processes to solve optimization problems [4,5].
Classical bio-inspired algorithms include genetic algorithm, ant colony optimization, artificial bee colony, particle swarm optimization, firefly algorithm, Japanese tree frog algorithm, Harris hawks optimization [6], slime mould algorithm [7], grey wolf optimization, sparrow search algorithm, whale optimization algorithm, etc. Fig. 1 shows the taxonomy of common bio-inspired optimization algorithms. There are some recent newly proposed bio-inspired algorithms, such as Siberian tiger optimization [8], jellyfish search algorithm [9], etc.
Figure 1: Taxonomy of common bio-inspired optimization algorithms
Bio-inspired optimization algorithms can be applied to engineering and sciences in several ways, such as data mining classification [10], biomarker extraction, food processing [11], image segmentation [12], renewable-powered smart grids [13], concurrent software [14], disease classification [15], lesion localization, treatment recommendation, power dispatch [16,17], mammogram diagnosis [18], rectangle layout problem [19], etc.
This special issue, bio-inspired optimization in engineering and sciences, is now calling for papers to the journal ‘Computer Modeling in Engineering & Sciences’. The aim is to report the recent advances in bio-inspired optimization in Engineering and Sciences. The ultimate goal of this special issue is to promote research and development of bio-inspired optimization theories and their applications in engineering and sciences by publishing high-quality research articles and surveys in this rapidly growing interdisciplinary field.
Funding Statement: The authors received no specific funding for this study.
Conflicts of Interest: The authors declare that they have no conflicts of interest to report regarding the present editorial.
References
1. Le, T. H., Thakur, D., Nguyen, P. K. T. (2022). Modeling and optimization of direct urea-hydrogen peroxide fuel cell using the integration of artificial neural network and bio-inspired algorithms. Journal of Electroanalytical Chemistry, 922, 116783. https://doi.org/10.1016/j.jelechem.2022.116783 [Google Scholar] [CrossRef]
2. Deepak, M., Rustum, R. (2023). Review of latest advances in nature-inspired algorithms for optimization of activated sludge processes. Processes, 11(1), 77. https://doi.org/10.3390/pr11010077 [Google Scholar] [CrossRef]
3. Zhang, Y. (2015). A comprehensive survey on particle swarm optimization algorithm and its applications. Mathematical Problems in Engineering, 2015, 931256. https://doi.org/10.1155/2015/931256 [Google Scholar] [CrossRef]
4. Hepzibah, R. I., Emimal, S. S. I. (2022). On comparison of crisp, fuzzy, intuitionistic fuzzy unconstrained optimization problems using Newton’s method. Communications in Mathematics and Applications, 13(4), 1295–1305. https://doi.org/10.26713/cma.v13i4.2187 [Google Scholar] [CrossRef]
5. Schultz, E. S., Olofsson, S., Mhamdi, A., Mitsos, A. (2022). Satisfaction of path chance constraints in dynamic optimization problems. Computers & Chemical Engineering, 164(4), 107899. https://doi.org/10.1016/j.compchemeng.2022.107899 [Google Scholar] [CrossRef]
6. Heidari, A. A., Mirjalili, S., Faris, H., Aljarah, I., Mafarja, M. et al. (2019). Harris hawks optimization: Algorithm and applications. Future Generation Computer Systems, 97, 849–872. https://doi.org/10.1016/j.future.2019.02.028 [Google Scholar] [CrossRef]
7. Li, S. M., Chen, H. L., Wang, M. J., Heidari, A. A., Mirjalili, S. (2020). Slime mould algorithm: A new method for stochastic optimization. Future Generation Computer Systems, 111, 300–323. https://doi.org/10.1016/j.future.2020.03.055 [Google Scholar] [CrossRef]
8. Trojovsky, P., Dehghani, M., Hanus, P. (2022). Siberian tiger optimization: A new bio-inspired metaheuristic algorithm for solving engineering optimization problems. IEEE Access, 10, 132396–132431. https://doi.org/10.1109/ACCESS.2022.3229964 [Google Scholar] [CrossRef]
9. Chou, J. S., Molla, A. (2022). Recent advances in use of bio-inspired jellyfish search algorithm for solving optimization problems. Scientific Reports, 12(1), 19157. https://doi.org/10.1038/s41598-022-23121-z [Google Scholar] [PubMed] [CrossRef]
10. Murugan, T. M., Baburaj, E. (2022). Comparative analysis of bio-inspired optimization algorithms in neural network-based data mining classification. International Journal of Swarm Intelligence Research, 13(1), 1–25. https://doi.org/10.4018/ijsir.2022010103 [Google Scholar] [CrossRef]
11. Sarkar, T., Salauddin, M., Mukherjee, A., Shariati, M. A., Rebezov, M. et al. (2022). Application of bio-inspired optimization algorithms in food processing. Current Research in Food Science, 5(4), 432–450. https://doi.org/10.1016/j.crfs.2022.02.006 [Google Scholar] [PubMed] [CrossRef]
12. Dhal, K. G., Glvez, J., Das, S. (2020). Toward the modification of flower pollination algorithm in clustering-based image segmentation. Neural Computing & Applications, 32(8), 3059–3077. https://doi.org/10.1007/s00521-019-04585-z [Google Scholar] [CrossRef]
13. Pop, C. B., Cioara, T., Anghel, I., Antal, M., Chifu, V. R. et al. (2022). Review of bio-inspired optimization applications in renewable-powered smart grids: Emerging population-based metaheuristics. Energy Reports, 8(1), 11769–11798. https://doi.org/10.1016/j.egyr.2022.09.025 [Google Scholar] [CrossRef]
14. Vilela, R. F., Neto, J. C., Pinto, V., de Souza, P. S. L., de Souza, S. D. S. (2023). Bio-inspired optimization to support the test data generation of concurrent software. Concurrency and Computation-Practice & Experience, 35(2), e7489. https://doi.org/10.1002/cpe.7489 [Google Scholar] [CrossRef]
15. Dhivya, P., Bazilabanu, A. (2023). Deep hyper optimization approach for disease classification using artificial intelligence. Data & Knowledge Engineering, 145(1), 102147. https://doi.org/10.1016/j.datak.2023.102147 [Google Scholar] [CrossRef]
16. Zhao, P., Zhang, Y. X., Hua, Q. Z., Li, H. P., Wen, Z. (2023). Bio-inspired optimal dispatching of wind power consumption considering multi-time scale demand response and high-energy load participation. Computer Modeling in Engineering & Sciences, 134(2), 957–979. https://doi.org/10.32604/cmes.2022.021783 [Google Scholar] [CrossRef]
17. Pawani, K., Singh, M. (2023). Combined heat and power dispatch problem using comprehensive learning wavelet-mutated slime mould algorithm. Electric Power Components and Systems, 51(1), 12–28. https://doi.org/10.1080/15325008.2022.2151666 [Google Scholar] [CrossRef]
18. Zhang, Y. (2016). Smart detection on abnormal breasts in digital mammography based on contrast-limited adaptive histogram equalization and chaotic adaptive real-coded biogeography-based optimization. Simulation, 92(9), 873–885. https://doi.org/10.1177/0037549716667834 [Google Scholar] [CrossRef]
19. Zheng, W. M., Si, M. C., Sui, X., Chu, S. C., Pan, J. Y. (2022). Application of a parallel adaptive cuckoo search algorithm in the rectangle layout problem. Computer Modeling in Engineering & Sciences, 135(3), 2173–2196. https://doi.org/10.32604/cmes.2023.019890 [Google Scholar] [CrossRef]
Cite This Article
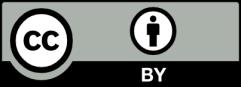