Open Access
REVIEW
A Systematic Review on the Internet of Medical Things: Techniques, Open Issues, and Future Directions
1
School of Computer Science and Engineering, Lovely Professional University, Punjab, 144411, India
2
Department of Computer Science, Babasaheb Bhimrao Ambedkar University, Satellite Centre, Tikarmafi, Amethi, 227413, India
3
Department of Computer Science, Maharaja Agrasen Institute of Technology, New Delhi, 110086, India
* Corresponding Author: Aditya Khamparia. Email:
(This article belongs to the Special Issue: Intelligent Biomedical Image Processing and Computer Vision)
Computer Modeling in Engineering & Sciences 2023, 137(2), 1525-1550. https://doi.org/10.32604/cmes.2023.028203
Received 04 December 2022; Accepted 20 February 2023; Issue published 26 June 2023
Abstract
IoT usage in healthcare is one of the fastest growing domains all over the world which applies to every age group. Internet of Medical Things (IoMT) bridges the gap between the medical and IoT field where medical devices communicate with each other through a wireless communication network. Advancement in IoMT makes human lives easy and better. This paper provides a comprehensive detailed literature survey to investigate different IoMT-driven applications, methodologies, and techniques to ensure the sustainability of IoMT-driven systems. The limitations of existing IoMT frameworks are also analyzed concerning their applicability in real-time driven systems or applications. In addition to this, various issues (gaps), challenges, and needs in the context of such systems are highlighted. The purpose of this paper is to interpret a rigorous review concept related to IoMT and present significant contributions in the field across the research fraternity. Lastly, this paper discusses the opportunities and prospects of IoMT and discusses various open research problems.Keywords
Kevin Ashton introduced the term Internet of Things (IoT) In 1999 [1]. Data regarding objects around the globe accessed through systems is restricted to data given by humans only. To overcome this, he visualized relating Radio Frequency Identification systems (RFIDs) as well as sensors with the internet. IoT is making the world better day by day. Every single thing which is linked with each other in the Internet of Things concept is counted as a smart entity and this is different from the traditional pattern. IoT can be termed as a self-configured interoperable network of virtual as well as physical devices with communication media, patterns, and protocols. These devices with the features and identity should have the capacity of linking to data networks like the internet as well as able to execute sensation, communication, information processes, and networking [2]. So, IoT is known to be a new term for Information and Communication Technology (ICT). The Internet of Things (IoT) can be known as the Internet of Everything as well as Industrial IoT, which has the huge capacity to redesign the whole business world. Various domains like social, economic, and technological including medical are offered by fast-growing IoT-based technology. ICTs impelled from the expansion and the favorable response of the internet had a large part in growing the quality, efficiency, and also the access, so success for any task regarding the medical [3–5].
In the present situation of healthcare, any human wishes should be assisted with the low-cost charge as well as considerable ease from employing technologies. Considering this, the Internet of Medical Things (IoMT) is an astonishing component for transforming traditional society [6]. Arising trends for intelligence in healthcare had restructured the target patients from the children’s age group to the elder age group. Particularly, the fast improvement in IoMT has grown the conventional healthcare system in different ways like analyzing as well as diagnosing the disease. Researchers utilize the data of patients’ health to diagnose as well as predict the disease. For the guaranteed healthcare domain, the change of traditional practices to digital format is the topmost preference to inspire the e-Healthcare domain, portable, handheld, or wearable sensors/devices that help to share or transfer the data, and manage the various entities. Therefore, holding in perspective the requirement and necessity of the rising trend of IoT are acquired along with the product lifecycle management, a determining and smart business aspect for notably running the lifecycle of IoT based system when nursing the patient in a hospital/medical centre or medical theatre [7,8].
It seems like each perspective of person lives for occurrence, factory to farm, city to village, healthcare to welfare, etc., have been involved. It includes interlinked devices like wireless body sensor networks, Wi-Fi, ZigBee, RFID, PDA, smartphone, tags, sensors, etc., which can sense, execute as well as transfer data in healthcare as well as in wearable, etc. [9]. The primary action towards the intelligent medical eco-system is to use the capability of traditional methodologies not only in serving the finest utilities to users but also creating their lives finer. For assisting healthcare professionals in nearly every region of their expertise like medical decisive action, Artificial Intelligence (AI) is one of the preeminent technologies which serve it. Techniques like Machine Learning (ML) and Deep Learning (DL) help the system to learn easy and difficult decisive actions by utilizing the data produced by the medical experts and feedback of patients [10–12]. Improvements in technologies like AI (Artificial Intelligence), sensors, and telemedicine help IoMT to make use of clinical decisions. The decision by humans endured with a virtual assistant which can study hidden attributes from the huge medical database with the help of advanced algorithms like Deep Learning is currently applied in a few hospitals, particularly in the radiology area.
Using IoMT, supplementary self-correcting capability may be rigged to enhance accuracy and ensuing feedback in the decision. By giving the latest information from various resources like journals, clinical exercises, and articles, a virtual assistant may stay doctors upgraded [13]. Internet of Medical Things can be applied to constant tracking of patient’s essentials, and can generate alert for varying health condition as well as in urgency takes essential precautions.
One more helpful technology for IoMT is Blockchain [14]. For the subject of it, the technology of blockchain with the capability of directing health records integration, ownership, control of access, and also integrity as shown in Fig. 1.
Figure 1: IoT architecture
The healthcare system or devices which produce psychological information by direct interaction with humans are applications of body-centric. This generated information is processed and then given to healthcare persons to do medical analysis. Medical non-wearables, as well as wearables, are examples of body-centric applications. These wearables or can say intelligent sensing devices can be put on a patient’s body like implantation or ornamentation. On the other side, non-wearables are intelligent sensing devices that cannot be put on a patient's body. Depending on the surroundings, applications of body-centric are classified into two types, i.e., indoor and outdoor [15].
Applications of object-centric are different from applications of body-centric type. Applications of object-centric have not straight concerned with the human body. But they regard healthcare solutions as well as services that can progress the healthcare system efficiently. The main example of this is HMS (Hospital management system). These applications are classified into two domains, one is indoor, and one is outdoor.
The IoT can be used to observe, report, and alert not only caretakers but give medical experts real data to detect the problem before they come out critically or to give permission for early prevention. IoMT is not proposed to take place of medical experts but to give them gained data from IoMT devices to make excellent diagnoses and treatments and to minimize inefficiency as well as waste in medical systems. For example, remote location patients are operating by IoMT by observing their records. Records contain the condition of the patient's medical track which can be chronic or long-term. IoMT locates them as well as directs them to the hospital with the help of wearable mHealth devices. These devices send the patient's data to their physician or healthcare person. The dashboard of analytics, as well as sensors, and equipped beds at hospitals, may analyze the vital symptoms and signs of the patient which make use of it in upcoming hospitals. By upgrading in-person care, the individual treatments which are data-driven may increase the living standard. Revised devices may be of all kinds in wearable devices as a regimen for the device in hospital and in-home in an intelligent method. With the help of mobile kits, devices at remote locations, and utility of care, medical devices expect may interact. It gives exactness, averts disease as well as maintains the status of health in urgency and remote mediation [16]. Nowadays, due to the COVID-19 pandemic, patients face problems with regular checkups or ongoing treatments during the lockdown period. In this case, it is beneficial for treatment from a remote location [17].
Integrating different technologies in medical areas to keep and better the quality of human lives, has exposed new scopes. It developed modern application domains as well as changed the method for working in the already existing areas like medical decision-making, patient data maintenance, and data gaining.
The novel contributions of this paper are summed up in two aspects.
First, a novel comprehensive study of components is required to develop the system as a system dependability analysis process which includes data acquisition, communication gateway, and cloud/server. It also reviews the drawbacks of such a comprehensive study. Second, the research gap, challenges, and research priorities. Some important contributions by showing:
1. To do a comprehensive study about real-time applications, methodologies, and techniques used in that application, advantages and disadvantages related to that system, issues, and challenges, we have reviewed the existing work of different applications (Section 2). It gives a complete idea about techniques with related advantages and future work/disadvantages. So, another researcher can analyze this literature review and they can select a suitable topic for their context.
2. A detailed study of components required to develop the system as system dependability explores 3 primary components, i.e., 1) Data Acquisition, 2) Communication Gateway, and 3) Cloud/Server. The data acquisition section includes biosensors, preprocessing, and A/D conversion. IoT gateway explores details of its architecture, its role, and wireless field connectors (Section 4).
3. Some notable threats to the system like slow internet connection/loss in internet connection, cyber-attacks, and faulty sensors/environmental effects on sensors have been covered in Section 5.
4. Different techniques can be used in the system for processing and analyzing the data like Signals Representation in Time and Frequency Domain, Data Preprocessing which mainly includes various AI/ ML techniques, and Blockchain has been discussed in Section 6.
5. The result and Discussion/Observation of the Review (Section 7) highlight a) the research gap and future scope in IoMT, b) Open issues in real-time IoMT applications, and c) Research priorities.
By summarizing the above contributions, the structure of this paper is drawn as Section 2. Discuss the existing work as Literature Review in Section 3. The methodology elaborates on the research questions. Components required to develop the system as system dependability are in Section 4. Threats to the system are discussed in Section 5. The system can use different techniques for processing and analyzing the data in Section 6. The result and Discussion/Observation of the Review are shown in Section 7. Section 8 concludes this whole research work.
Several works can be seen in the literature about various existing IoMT applications. Their main features and drawbacks are highlighted to find current problems to be solved for the next-generation applications.
Reference [18] proposed Medication Control system. In this, RFID EPC is used as an object identifier as well as an informed reader. To use e-Health services, a web application has been developed.
Reference [19] offered end-to-end security framework for portable medical IoT applications. This is 3 layer framework: device layer, fog layer, and cloud layer. The security of the proposed system was designed by utilizing a DTLS certificate between gateways and end users.
Reference [20] proposed food contamination monitoring system with the help of a small-size immunosensor and wireless internet connection. This allows us to analyze food and show the analysis.
Reference [21] proposed Medicine Reminder and Monitoring System.
Reference [22] proposed IoT-driven glucose level monitoring system with the utilization of a back-end PHP server and front-end tools. The sensor node is integrated with a power unit and energy-controlled unit for effectively utilizing the operation and working of installed sensor devices. The glucose level of patients will be monitored effectively in a real-time environment.
Reference [23] presented work discussed different human body postures and their bottlenecks with integrating IoT sensor devices. The complete dataset would be provided with help of Bayesian calculation which predicts the body movement based on joint probability distributions. This system utilizes low-level hardware management units with low complexity to facilitate the model in the real world.
CHV is a mosquito-borne virus that causes human morbidity and mortality. In the proposed work, to detect and diagnose CHV, fog-based sensor computing units installed in different distribution sites send mobile alerts to end users. This system utilizes FCM clustering for grouping the fog nodes. The obtained computational results compared with cloud technology optimize bandwidth efficiency, execution time, and delay in sending alerts or notifications [24].
In this work, medical sensors are integrated into the human body to detect and predict the diabetes type and level associated. Artificial neural networks and the fuzzy system were amalgamated for diabetes-type classification and the levels associated with them [25].
Reference [26] proposed a deep learning algorithm to recover the carcinoma and mammographic data from IoMT-driven sensor nodes. The comparative assessment of both models will be done and performed with disease data and normal healthy clinical data.
In this work, IoT based system has been utilized for the diagnosis of sleep apnea by utilizing cloud-based big data architecture. Finally, a Web UI application was developed for continuous monitoring of the elderly's sleep apnea [27].
This paper proposed an IoMT platform consisting of sensor nodes with a reward-based mechanism as a means of distributing the energy among the nodes so that the life cycle is extended [28].
In this paper, authors proposed a framework for breast cancer detection using cytology images to classify tumor types either benign or malignant. The feature selection would be performed with help of a random forest algorithm which would be later classified by neural network models. The results obtained using real data sets during experimentation validate the classification accuracy of up to 98% for NB as compared to SVM and RF [29].
The review aims to get knowledge about real-time applications, methodologies, and techniques used in that applications, advantages and disadvantages related to that system, issues, and challenges concerning those applications from existing work in it. So, we reviewed 42 papers that were published from 2011 to 2020 in Table 1.
A variety of literature was reviewed to gain insights into IoMT work, i.e., how authors proposed or analyzed different IoMT applications as well as dependability in the IoMT system. The main aim of the literature review is to explore and understand the real-time application, benefits, issues, and upcoming trends in research. To get deep knowledge, we divide work into sections (Research questions, search scheme, inclusion standard, dependability study, threats, discussion).
Deciding RQs is the basic strategical step while doing a detailed literature review. We highlight the points that are focused on the dependability analysis of it. From this consideration, we constructed RQs as below:
RQ1. Which components are required to develop the system as system dependability?
Before constructing any IoMT system, it is necessary to know what components are required to develop system dependability. Due to this, it becomes easy to detect specific dependability as per the requirement of the system at an early stage of development.
RQ2. Which are the issues or challenges that can be a threat to the system?
After the development of the system, when it will use in real-time some challenges or issues can obtain which may become a threat to the system's dependability. To prevent the system from those threats we should take that challenges and issues into consideration.
RQ3. Which are the different techniques that can be used in the system for processing and analyzing the data?
In most of the IoMT applications, a privilege has been provided to process and analyze patient data or any other device data by the system itself. For this procedure, many techniques are available, like artificial intelligence and machine learning algorithms, data mining techniques.
RQ4. Result and Discussion/What is the observation of the review?
In this section, we will discuss observations or highlights of the review. Also, we will discuss the future scope of IoMT. And a survey of research with different graphs.
For finding research and review papers related to IoMT, we just went with Google Scholar, and with a custom range for the year, we downloaded papers year-wise and specific publications. In the search string we used (“IoT” OR “IoMT” OR “IoHT”) AND (“Healthcare” OR “Health” OR “Medical” OR “Biosensors” OR “Disease”).
We included papers for a systematic review that have mentioned topics that answer constructed RQs directly or indirectly. The following are the main criteria for referring papers for this review (anyone criterion should be fulfilled):
1. Should have mentioned the architecture and components of IoMT
2. Should have mentioned or proposed a review of real-world application/s of IoMT
3. Should have mentioned challenges or issues in IoMT
4. Should have mentioned techniques or technologies for processing and analyzing collected data
4 Components Required to Develop the System as System Dependability (RQ1)
IoMT system contains 3 primary components, i.e., 1) Data Acquisition, 2) Communication Gateway, 3) Cloud/Server as shown in Fig. 2. We can say that this is the dependability of the IoMT system because the quality of service depends upon each component as shown in Eq. (1).
Its first component is data acquisition and it is particularly dependent on sensors as well as users. Gathered data of patients using various types of sensors, went through preprocessing actions and then transferred to the cloud/server. Different data processing methodologies have been developed for extracting and classifying needy information. Processed information utilized by medical experts to analyze. Interchanging the information between the user and components is done through a communication gateway.
Figure 2: IoMT components
The procedure of acquiring biological data for needy applications is called data acquisition. This biological data is acquired by using various biosensors that represent data as analog signals. Normally, these data signals have noise and also low amplitude. So, it is important to preprocess them and then digitize them well.
Fig. 3 refers to how data has been acquired and what steps are involved in preprocessing. Preprocessing involves operations like amplifying and filtering analog data signals. While acquiring the data, the important factor is to make sure that the data is well-conserved with no loss, else during diagnosis it may cause wrong decisions.
Figure 3: Basic operations in data acquisition
For acquiring the biomedical signals of the patient, we prefer biosensors which are electronic devices. They are devices that are used for the analysis of biological elements like tissues, enzymes, or other biometric components. Also, to collect patient data like temperature, to count heart rate, and ECG, to monitor fitness. Biosensors can produce huge data and transfer it to medical experts. Also, they can process produced data by themselves and make diagnose fast by permitting people to observe their health [61–67]. For IoMT, the classification of biosensors into wearables and non-wearables.
Wearable biosensors as the name suggest biosensors which can be worn on the body like accessories/implants. This technology is a kind of intelligent sensing device. This type of biosensor provides a large capacity to produce data as well as allow communication with another device. Same to wearable sensors, Non-wearable sensors can sense and produce huge data and provide communication with other devices except they cannot be worn on the human body.
This operation contains artifact (like ECG) removal, filtration of noise, and resampling of collected signals to send these preprocessed signals or data for analog to digital conversion [8]. Biosensors collect biological signals and they are weak and also have a noise that causes hard to process. So, it is necessary to amplify them with the correct amplifier. Generally, an amplifier contains an electronic circuit that results in signals with high amplitude. This amplifying of signals does not change other attributes of collected signals like morphology and frequency. An amplifier’s circuit contains components like conductors, transistors, and resistors. If an amplifier has a single transistor then it is a single-stage amplifier otherwise a multi-stage amplifier. For real-time use, the multi-stage amplifier is popular. Gain is a measurement for counting amplification amounts. And this measurement is calculated as ratio values of output to the input of amplifier as in the following Eq. (2).
To separate unwanted portions of signals, filtering is applied to signals after the process of amplification. There are various filters available for the filtering of signals. They are classified into 4 types as shown in Fig. 4; low pass filter, high pass filter, bandpass, and bandstop filter.
Figure 4: Types of filters
A/D converters are used to convert analog signals to digital signals. Fig. 5 compares the analog and digital versions of a recorded signal. A/D comprises sampling and quantization techniques. Sampling converts continuous signals to discrete-time and measures signal values at certain time duration/intervals. The quantization converts a range of numeric values into finite discrete values. Every sample is quantized and stored in binary bits. 16 bit A/D converter can represent 65,536 amplitude levels while 8 bit A/D converter can represent only 256 amplitude levels.
Figure 5: General architecture of IoMT gateway
Internet of Things (IoT) gateways are software programs or physical devices which are connected to smart systems, sensors, servers as well as data systems to each other [68–70]. The data sent to the servers and vice versa passes via this gateway. So, it is known as a communication bridge for various IoMT components.
Data Normalization—Various sensors are used to collect data. This collected data is present in various data patterns. The role of the gateway here is to receive different datasets and transform these datasets into a single format.
Data Preprocessing–It is important to preprocess data by the gateway before sending it to the server. This operation does the minimization as well as filters data that must be sent forward. And also minimizes the conveyance, storage needs, and processing.
Network Connectivity–Sensors are lacking with ability to connect network. They are very poor at connecting directly with huge networks such as WAN. So, the gateway is used to provide a connection with external networks with the help of the internet, Wi-Fi, or many more options.
4.2.2 Architecture of IoMT Gateway
Fig. 5 refers to the general architecture of gateway systems. In general, it contains the CPU and WFC. Normally, sensors are not inbuilt into the gateway. But the gateway obtains the data by wireless sensors with the help of field connectors like ZigBee, NFC, or many more available options. Acquiring of data is in the form of small chunks and transferred to the central processing unit to preprocess, which is a key component of the system.
The gateway consists of an embedded system. This system is considered the core of the architecture. The key responsibilities of the gateway system are collecting data and then preprocessing and managing that collected data. During preprocessing of that collected data, the gateway must decide on data whether it should be kept or removed. To keep operations stable, it is important to handle external issues. This type of obstacle may lead to disturbance in gateway processing. A power outage is the best example of an external issue. In order to ensure continuity, the system must automatically restart and resume operations from where it stopped once the issue is resolved. After preprocessing and filtration, the system transfers the data to the server/cloud. Then there will be further processing as well as analysis.
4.2.3 Wireless Field Connectors
Generally, IoMT utilizes protocols like WFCs to communicate. This protocol is also known as a communication bridge between intelligent devices. From the scope of applications in various areas, the classification of WFCs is in 2 classes, i.e., CAN (Close Area Network) and BAN (Broad Area Network).
CAN refers to the network where devices are connected for a close distance. PAN, LAN, and HAN are examples of CAN.
Personal Area Networks (PAN)–It is good for a single person where the distance for the network within up to 15 meters. PC, mobile phones, input-output devices (like printers, and scanners), and many more individual-use devices are the devices for interconnection in this network.
Home Area Networks (HAN)–As the name suggests, this network is used to communicate between intelligent devices in the area covered by the home of the user. This network uses intelligent security, smart television, telephones, and many more intelligent domestic devices for interconnection.
Local Area Networks (LAN)–This network is used for one or a group of buildings. The area covered by this network must be a few square km. LAN can be used for a residential society, a school/college campus area as well as hospitals.
Short-range WFCs are used for this network as devices for interconnection. These devices consume less power and exchanging of data between devices is done in small chunks. Some technologies to communicate and various WFCs are as follows.
Bluetooth technology is older technology but is now recognized worldwide as a popular wireless transmission methodology [71,72]. It is inbuilt technology in various smart devices like mobile phones, laptops, and many input-output devices. IoMT is one of the domains of wide Bluetooth adoption. Many biosensors can easily communicate with smartphones with the help of Bluetooth. ZigBee is known as a trustable standard protocol utilized to communicate wirelessly [73]. It requires large power than Bluetooth which provides devices to communicate and send commands backward and forward. RFID is an automation technology that helps systems to detect an object as well as produce metadata using radio waves [74,75]. It contains tags and readers. Tags are used to detect an object and the readers are used to transmit data. NFC is the same as RFID where it uses radio waves for communication purposes. It is used to interconnect devices within a short distance.
A broad area network is used for applications requiring a device connection for a significant distance. CAN, WAN and MAN are specific examples.
Controller Area Networks (CAN)–Speedy communication network typically proposed for the industry of automation. Microcontrollers, as well as smart devices, can connect and also exchange data with each other and there is no need for the host computer.
Metropolitan Area Networks (MAN)–It is the same as LAN except this is used for the area as large as the metropolitan area. It is larger than LAN and smaller as compared to WAN. A city or a state is an example of MAN.
Wide Area Networks (WAN)–This network is used for an area as large as a wide geographical area. This network can cover many cities, states, or countries.
Long-range WFCs are used for this network as devices for interconnection. In general, these devices consume high power than short-range WFCs.
Wi-Fi offers wireless communication. It processes in a limited area and has large power consumption. Radio waves are used to transmit data. Wi-Fi offers high-speed internet accessibility. Mobile Data is a very common technology in smartphones to connect users worldwide. It can be used in the real-time medical domain. A satellite is a very efficient connector to connect from any geographical area and is mainly useful in that remote areas where they have less, or no telecommunication is possible.
The server is known as a key part of the IoMT system that finds unusual activities as well as executes analysis. Servers receive digital data from the gateway which is preprocessed for data mining. For IoMT, the classification of critical data of biosignals is data mining. Some techniques for signal processing as well as data mining are in the RQ3 section.
Case study (COVID-19)
Due to the COVID-19 pandemic, patients face problems with regular checkups or ongoing treatments during the lockdown period. In this case, IoMT is beneficial for treatment from a remote location. Also, IoMT can help to fight COVID-19. Table 2 shows the review of research on how IoT/IoMT can help with COVID-19.
5 Limitations or Threats to the System (RQ2)
5.1 Slow Internet Connection or Loss in Internet Connection
The internet plays an indispensable role in IoMT. We can say that the system has no use without the internet because things are connected via the internet only. Sometimes (most of the time in a rural area) the internet goes down. In remote monitoring, if there is an emergency and at the same time lack of internet, then the system will fail in that case. For many applications, not only patients record but also processing and analyzing mechanisms stored and deployed at the cloud. Suppose a healthcare person or medical experts wants to see a patient's record or some analyzing process should be taken and there is no internet or slow internet. In that case, there will be a delay in the processor may be some serious loss can take place. Without the internet, sensors cannot send captured data to the cloud. It may result in a wrong diagnosis which may be hazardous in the future.
The main objective of the IoMT is to make life easier, and thus usability is the ultimate yet essential design factor for improving patient safety and quality of care. Usability is usually tested using input from customers, and product defects are found. It is necessary to adapt the standards for app development and user experience to make elements work in harmony within the network. The world is entering the era of mobile apps that can anticipate and socialize and there is a growing need for well-defined levels of user experience.
5.3 Faulty Sensors/Environmental Effect on Sensors
Sensors and their credibility play a vital role in IoMT. They generate huge data. Different methods are applied for different applications as to at what intervals data should be taken. Once the data is gathered it is then further transferred for analysis or processing. A drawback in this process is data corruption or invalid or missing data may have occurred. Even with the proper maintenance, faulty sensors can capture incorrect data while the application is executing for the real-time healthcare system and it may threaten somebody’s health seriously and cause the failure to the system. It has a drastic effect on the overall operation. Some sensors have an environmental impact on them. So, they are unable capture to capture data, or if captured, then it may be incorrect.
Medicine depends more on innovations in the 21st century. One of the biggest healthcare infrastructure problems is its spiraling cost. Hospitals spend billions of dollars each year on advanced technological devices. However, these new improvements lead to costly treatment which affects a lot of people. This situation needs to be taken into consideration as a matter of urgency, and new deployment strategies for minimum equipment and operating costs need to be developed. IoMT plays a crucial role in strengthening the overall situation in that respect. There have already been various IoMT-based monitoring devices available in markets. Such tools will immediately transfer data directly to caregivers through online applications. Thus, smart IoMT devices can also save time and money [76–79].
Imprecise data can be inaccurate and can be detrimental to the patients. It is important to ensure the reliability and performance of the system stay the same despite several uses. Otherwise, to request a repair or substitution, the program will produce alarms or notifications. IoMT plays a very effective role in delivering more accurate healthcare, such as integrating and analyzing various types of medical records and using them in clinical decision-support systems. In this way, caregivers could get a complete picture of the health of each patient, resulting in faster treatment and being able to perform more precise treatments [80].
A secure IoMT system is very crucial in healthcare. As more and more devices interact with each other, maintaining the protection and privacy of patient data is crucial. Security holes cause hackers to steal patient personal information which contributes to crimes like identity theft, exposure to illegal drugs which false insurance claims. Safe preservation of diseases, vitalities, and data collected through sensors has also become a major concern of IoMT technology. Furthermore, security vulnerabilities in the hospital system may result in substantial infrastructure disruption, which in some cases can also be fatal. Device authentication is one of the essential components to secure an IoMT infrastructure. Some systems do not accept advanced authentication protocols because of inadequate computing capacity and energy. Therefore, the development of faster and lower power processors with new authentication schemes is essential to overcome security issues [80–82].
In an IoMT environment, nearly all depend on electricity. If electrical appliances are improperly used or operated, they may actually be dangerous and may inflict extreme discomfort, burn burns, and even fatalities. Organizations and hospitals have to take appropriate steps to ensure settings or appliances that are safe from electrical hazards. Also, general safety check requirements such as IEC60601-1 must be adopted to ensure maximum protection when building these devices [83].
Most wireless devices operate 7/24 times and consume considerable amounts of energy. A new challenge is the manufacturing of renewable energy-friendly products. IoMT allowed researchers to apply new techniques to reduce the energy consumption of various wireless devices. Also, however, the volume of data generated needs to be streamlined and the resources needed for storing and transmission that. There is also a need for energy harvesting modules due to the miniaturization of electronic components which can turn various energy sources into electrical energy such as wind, sunlight, etc. These methods will significantly reduce power consumption and will result in lower operation costs [84].
6 Different Techniques Can Be Used in the System for Processing and Analyzing the Data (RQ3)
6.1 Signals Representation in Time and Frequency Domain
Time and frequency domains are used to represent the bio-signals. Over time, signals obtained from sensors are registered, known as the time-domain signals. A time-domain signal at a certain point in time provides amplitude information of a signal. Other features such as the signal-to-noise ratio (SNR) and performance event detection can be extracted using the time and amplitude information.
Frequency-domain is another way of expressing the time-domain signals. At increasing frequency, the frequency-domain includes the power information. Usually, a Fourier transform is performed to measure the amplitude of each frequency. Several features of the frequency-domain signal can be extracted, including centroid frequency, sub-band energy, and spectral bandwidth [85].
In IoMT, data mining plays a major role in a patient’s early detection and continuing surveillance. This process includes huge biodata to extract patterns and separating useful information.
Usually, data mining is divided into two different types, supervised and unsupervised. A classification algorithm is trained using labeled data in the supervised model and is used to classify unlabeled data. Labeling data means that each input variable is labeled with a suitable output. Where as there are no labeled data in the unsupervised model. An unsupervised classification algorithm, therefore, attempts to cluster the data into various classes and discovers relationships within themselves. In this section, the discussion includes various data mining methodologies like SI (Swarm Intelligence), LDA (Linear Discriminant Analysis), K-C (K-means Clustering), DT (Decision Tree), NB (Naive Bayes), VQ (Vector Quantization), ANN (Artificial Neural Networks), and FL (Fuzzy Logic).
SI is a method of classification used to design diagnostic models [86]. SI used a method to diagnose cordis disease with arrhythmias. Optimal solutions can be sought easily by particle swarm optimization in large search spaces. The problem of optimization solved in the proposed analysis includes features found mainly in the classification. Less number of features lead to a speedier cycle of classification. The study showed that, since suitable parameters are selected, the overall classification results can be improved by using particle swarm optimization. LDA is a methodology for classification which produces separates samples effectively [87]. Every part holds many attributes. Some attributes occur in large dimension rea while some in smaller ones. The main functionality of LDA is projecting high dimensional attributes to smaller dimensional space. So this technique is called a dimensionality reduction technique. A decision tree is decision making and also supervised methodology to classify problems and in regression also. It has tree representation and roots as sample data and child nodes as decision nodes. One more ML algorithm applied for classification is Naïve Bayes (NB) [87–89]. This algorithm holds probabilistic functionality. To find a countable set of classes, an unsupervised algorithm VQ is used. This includes K-means, X-means methodologies. An artificial neural network is a network of a large number of neurons where they are clustered in many levels [90]. But general ANN contains 3 levels as input, hidden (processing), output. FL algorithm is dependent on the “degrees of truth” concept to detect an approximate but not a completely correct or precise solution [91]. When we combine the term Big Data with healthcare, some other components also intersect, like Mathematics, computer science, statistics, and healthcare. When we talk in the healthcare sphere then, we have seen that there are over 10.7 billion objects and devices that are connected to the internet and this number is expected to grow exponentially. So, for this much data to handle, we need Big Data so we can filter out the important data and somehow make it autonomous by using various devices, like wearable technologies, biosensors and mHealth have increased the data which is captured as biological data. Big data is mainly focused on three varieties like Transactional data, Machine or clinical data, and social data [92–94].
As currently, there are 10.7 billion devices connected to the internet and IoT has estimated 50 billion devices to be connected to the internet from different domains. As this number increases and so the privacy and security of these so many users in IoMT. Then at this specific part, Blockchain comes into effect so that we can secure the Internet of Medical Things. As there will be so many users, data privacy is very important especially if it is medically related. In the proper functioning of Blockchain, various factors like sensor networks, Big data analysis, and wearable devices are necessary to gather data, and then there will be much life-critical medical information and analyzing the data to make a critical life decision. Then by using Blockchain we can prevent the vulnerabilities which are targeted by cybercriminals in these IoMT devices and they also want to access the hospital's network and take information that is sensitive of the patients and then harm them or threaten them [95].
7 Result and Discussion/Observation of the Review (RQ4)
In this paper, we reviewed papers on the Internet of Medical Things based on various topics, i.e., What is IoMT, Architecture of IoMT, Impact of IoMT, Applications, Biosensors used in IoMT, IoMT gateway, server/cloud, Data and processing techniques, and challenges. Observation of the review is divided into parts as a) research gap and future scope in IoMT, b) Open issues in real-time IoMT applications, c) Research priorities. We have reviewed 93 papers overall. In Fig. 6a, we can observe increasing research in IoMT. In Fig. 6b, we can see 63% of IoMT applications are in personal healthcare device (PHD) and 37% for public healthcare or applications in public places like hospitals, railway stations, etc. In Fig. 6c, techniques used for the IoMT system have been reviewed. As per the observation of the review, there are common issues in real-time applications, i.e., cost and security [96–100].
Figure 6: (a) Year wise research work (b) Percentage of applications (c) Techniques used in IoMT
7.1 What Is the Gap in Research and Future Scope?
IoMT is a combination of IoT and the medical domain. So, the research gap for IoMT is somehow the same as the research gap for IoT and the medical domain. Technical issues like hacking of patient’s data, error in managing patient’s data, a fewer amount of expertise, high power consuming biosensors, Hardware implementation, and design optimization are presented. Therefore, it is necessary to implement or apply different security protocols and algorithms while transferring and managing data. To use less power consumption by biosensors, we can work on automatic power scheduling for using biosensors. The upcoming market of IoMT needs to include more experts to get a more precise analysis of the disease and to provide correct treatment to the patient. New technologies like artificial intelligence, machine learning, data mining, image processing, NLP can use to analyze many diseases. Nowadays, due to the COVID-19 pandemic, patients face problems for regular checkups or ongoing treatments during the lockdown period. In this case, IoMT is beneficial for treatment from remote locations. Also, we can see an increasing number of psychological diseases day by day. Normally, many people feel shy or not comfortable sharing this type of disease. IoMT can help these patients with human-computer interaction (HCI) system [101,102], NLP, monitoring [103,104] and diagnosing patient’s behavior.
Following are some open issues in review:
• To deal with automation, it is important to take cost and security into consideration.
• There are many personal healthcare IoMT applications are available for elderly people. Due to lack of knowledge or unknown to digitalization, they cannot utilize applications.
• The lack of an automatic data recovery system can be the reason for the failure of the system.
• Due to expensive sensors or the IoMT system, common people refuse to use it.
• Some applications or sensors should reduce in size.
• Delay in decision-making by the system in emergencies may result in loss.
• Some sensors have an environmental impact on them. So, they are unable to capture accurate data and hence decision-making will be wrong.
• Lack of internet connection or slow internet connection unable to transfer data to the cloud and also unable to process as well as analyze data. This may cause hazardous results in emergencies.
• Personal healthcare IoMT applications for smartphone slows down the system for a while when analyzing of data takes place.
While developing any IoMT system following parameters should be prioritized:
• Before constructing any IoMT system, it is necessary to know what components are required for development as system dependability [105]. Due to this, it becomes easy to detect specific dependability as per the requirement of the system at an early stage of development.
• After the development of the system, when it will use in real-time some challenges or issues can obtain, which may become a threat to system dependability. To prevent the system from those threatening we should take that challenges and issues into consideration (security, reliability, privacy, cost, accuracy, precision, electrical safety, low power consumption, type of network, etc.).
• In most of the IoMT applications, a privilege has been provided to process and analyze patient’s data or any other device data by the system itself. For this procedure, many techniques are available like artificial intelligence and machine learning algorithms, data mining techniques, etc.
• When automation is provided, then it is necessary to ensure the system does not face extra overhead.
IoMT has transformed the conventional healthcare system in different ways like analyzing as well as diagnosing the disease. Researchers utilize the data of patients’ health to diagnose as well as predict the disease. For the guaranteed healthcare domain, the change of traditional practices to digital format is the topmost preference to inspire the e-Healthcare domain. Portable, handheld, or wearable sensors/devices help to share or transfer the data, and manage the various entities.
In this paper, we tried to elaborate on system dependability components for building IoMT system. The review encompasses various components, including the dependability of the system, different techniques and technologies used for the development of the system, as well as underlying notable challenges/limitations and threats to the system applications. Based on a review of existing work, we highlighted and discussed some crucial and common challenges which are suggested to be overcome in future work. Also, we proposed some new technologies and techniques that can be used to develop systems for achieving precise results in the future. The discussion of research priorities suggests priority parameters that must be taken into consideration while developing any application.
In this paper, we have presented a review of IoMT. For the systematic review, we constructed four research questions. We reviewed real-time applications, methodologies, and techniques used for processing and analyzing data, advantages, and disadvantages, and issues and challenges concerning that application from existing work in IoMT. Most of the applications have some benefits such as being useful in emergencies, remote monitoring, etc., and some drawbacks like security, privacy, and high power consumption.
Funding Statement: The authors received no specific funding for this study.
Conflicts of Interest: The authors declare that they have no conflicts of interest to report regarding the present study.
References
1. Elazhary, H. (2019). Internet of Things (IoTmobile cloud, cloudlet, mobile IoT, IoT cloud, fog, mobile edge, and edge emerging computing paradigms: Disambiguation and research directions. Journal of Network and Computer Applications, 128(2), 105–140. https://doi.org/10.1016/j.jnca.2018.10.021 [Google Scholar] [CrossRef]
2. Čolaković, A., Hadžialić, M. (2018). Internet of Things (IoTA review of enabling technologies, challenges, and open research issues. Computer Networks, 144, 17–39. https://doi.org/10.1016/j.comnet.2018.07.017 [Google Scholar] [CrossRef]
3. Ray, P. (2018). A survey on Internet of Things architectures. Journal of King Saud University-Computer and Information Sciences, 30(3), 291–319. https://doi.org/10.1016/j.jksuci.2016.10.003 [Google Scholar] [CrossRef]
4. Campos, J. (2009). Development in the application of ICT in condition monitoring and maintenance. Computers in Industry, 60(1), 1–20. https://doi.org/10.1016/j.compind.2008.09.007 [Google Scholar] [CrossRef]
5. Papanastasiou, G., Drigas, A., Skianis, C., Lytras, M., Papanastasiou, E. (2018). Patient-centric ICTs based healthcare for students with learning, physical and/or sensory disabilities. Telematics and Informatics, 35(4), 654–664. https://doi.org/10.1016/j.tele.2017.09.002 [Google Scholar] [CrossRef]
6. Al-Turjman, F., Nawaz, M. H., Ulusar, U. D. (2020). Intelligence in the Internet of Medical Things era: A systematic review of current and future trends. Computer Communications, 150(1), 644–660. https://doi.org/10.1016/j.comcom.2019.12.030 [Google Scholar] [CrossRef]
7. Savaridass, M. P., Ikram, N., Deepika, R., Aarnika, R. (2021). Development of smart health monitoring system using Internet of Things. Materials Today: Proceedings, 45(2), 986–989. https://doi.org/10.1016/j.matpr.2020.03.046 [Google Scholar] [CrossRef]
8. da Costa, C. A., Pasluosta, C. F., Eskofier, B., da Silva, D. B., da Rosa Righi, R. (2018). Internet of Health Things: Toward intelligent vital signs monitoring in hospital wards. Artificial Intelligence in Medicine, 89(2), 61–69. https://doi.org/10.1016/j.artmed.2018.05.005 [Google Scholar] [PubMed] [CrossRef]
9. Yin, Y., Zeng, Y., Chen, X., Fan, Y. (2016). The Internet of Things: An overview. Journal of Industrial Information Integration, 1(4), 3–13. https://doi.org/10.1016/j.jii.2016.03.004 [Google Scholar] [CrossRef]
10. Salehi, H., Burgueño, R. (2018). Emerging artificial intelligence methods in structural engineering. Engineering Structures, 171(1), 170–189. https://doi.org/10.1016/j.engstruct.2018.05.084 [Google Scholar] [CrossRef]
11. Mahdavinejad, M. S., Rezvan, M., Barekatain, M., Adibi, P., Barnaghi, P. et al. (2018). Machine learning for internet of things data analysis: A survey. Digital Communications and Networks, 4(3), 161–175. https://doi.org/10.1016/j.dcan.2017.10.002 [Google Scholar] [CrossRef]
12. Gill, S. S., Tuli, S., Xu, M., Singh, I., Singh, K. V. et al. (2019). Transformative effects of IoT, blockchain and artificial intelligence on cloud computing: Evolution, vision, trends and open challenges. Internet of Things, 8(1), 100118. https://doi.org/10.1016/j.iot.2019.100118 [Google Scholar] [CrossRef]
13. IBM Watson–How to replicate Watson hardware and systems design for your use in your basement (Inside System Storage) (2004). https://www.ibm.com/support/pages/ibm-watson-how-replicate-watson-hardware-and-systems-design-your-own-use-your-basement [Google Scholar]
14. Farouk, A., Alahmadi, A., Ghose, S., Mashatan, A. (2020). Blockchain platform for industrial healthcare: Vision and future opportunities. Computer Communications, 154(10), 223–235. https://doi.org/10.1016/j.comcom.2020.02.058 [Google Scholar] [CrossRef]
15. Al-Turjman, F., Nawaz, M. H., Ulusar, U. D. (2020). Intelligence in the internet of medical things era: A systematic review of current and future trends. Computer Communications, 150(1), 644–660. https://doi.org/10.1016/j.comcom.2019.12.030 [Google Scholar] [CrossRef]
16. Marr, B. (2018). Why the Internet of Medical Things (IoMT) will start to transform healthcare in 2018. https://www.forbes.com/sites/bernardmarr/2018/01/25/why-the-internet-of-medical-things-iomt-will-start-to-transform-healthcare-in-2018/?sh=52d266774a3c [Google Scholar]
17. Singh, R. P., Javaid, M., Haleem, A., Vaishya, R., Ali, S. (2020). Internet of Medical Things (IoMT) for orthopaedic in COVID-19 pandemic: Roles, challenges, and applications. Journal of Clinical Orthopaedics and Trauma, 11(4), 713–717. https://doi.org/10.1016/j.jcot.2020.05.011 [Google Scholar] [PubMed] [CrossRef]
18. Laranjo, I., Macedo, J., Santos, A. (2012). Internet of Things for medication control: Service implementation and testing. Procedia Technology, 5, 777–786. https://doi.org/10.1016/j.protcy.2012.09.086 [Google Scholar] [CrossRef]
19. Moosavi, S. R., Gia, T. N., Nigussie, E., Rahmani, A. M., Virtanen, S. et al. (2016). End-to-end security scheme for mobility enabled healthcare Internet of Things. Future Generation Computer Systems, 64(4), 108–124. https://doi.org/10.1016/j.future.2016.02.020 [Google Scholar] [CrossRef]
20. Seo, S. M., Kim, S., Jeon, J., Kim, J., Kim, H. et al. (2016). Food contamination monitoring via Internet of Things, exemplified by using pocket-sized immunosensor as terminal unit. Sensors and Actuators B: Chemical, 233(2), 148–156. https://doi.org/10.1016/j.snb.2016.04.061 [Google Scholar] [CrossRef]
21. Zanjal, S. V., Talmale, G. R. (2016). Medicine reminder and monitoring system for secure health using IOT. Procedia Computer Science, 78(2), 471–476. https://doi.org/10.1016/j.procs.2016.02.090 [Google Scholar] [CrossRef]
22. Gia, T. N., Ali, M., Dhaou, I. B., Rahmani, A. M., Westerlund, T. et al. (2017). IoT-based continuous glucose monitoring system: A feasibility study. Procedia Computer Science, 109(18), 327–334. https://doi.org/10.1016/j.procs.2017.05.359 [Google Scholar] [CrossRef]
23. Zhang, C., Lai, C. F., Lai, Y. H., Wu, Z. W., Chao, H. C. (2017). An inferential real-time falling posture reconstruction for Internet of Healthcare Things. Journal of Network and Computer Applications, 89(3), 86–95. https://doi.org/10.1016/j.jnca.2017.02.006 [Google Scholar] [CrossRef]
24. Sood, S. K., Mahajan, I. (2017). Wearable IoT sensor based healthcare system for identifying and controlling Chikungunya virus. Computers in Industry, 91(1), 33–44. https://doi.org/10.1016/j.compind.2017.05.006 [Google Scholar] [PubMed] [CrossRef]
25. Kumar, P. M., Lokesh, S., Varatharajan, R., Chandra Babu, G. C., Parthasarathy, P. (2018). Cloud and IoT based disease prediction and diagnosis system for healthcare using Fuzzy neural classifier. Future Generation Computer Systems, 86(3), 527–534. https://doi.org/10.1016/j.future.2018.04.036 [Google Scholar] [CrossRef]
26. Turabieh, H., Abu Salem, A., Abu-El-Rub, N. (2018). Dynamic L-RNN recovery of missing data in IoMT applications. Future Generation Computer Systems, 89(2), 575–583. https://doi.org/10.1016/j.future.2018.07.006 [Google Scholar] [CrossRef]
27. Yacchirema, D., Sarabia-Jácome, D., Palau, C. E., Esteve, M. (2018). System for monitoring and supporting the treatment of sleep apnea using IoT and big data. Pervasive and MobileComputing, 50(3), 25–40. https://doi.org/10.1016/j.pmcj.2018.07.007 [Google Scholar] [CrossRef]
28. Rajasekaran, M., Yassine, A., Hossain, M. S., Alhamid, M. F., Guizani, M. (2019). Autonomous monitoring in healthcare environment: Reward-based energy charging mechanism for IoMT wireless sensing nodes. Future Generation Computer Systems, 98(6), 565–576. https://doi.org/10.1016/j.future.2019.01.021 [Google Scholar] [CrossRef]
29. Khan, S. U., Islam, N., Jan, Z., Din, I. U., Khan, A. et al. (2019). An e-Health care services framework for the detection and classification of breast cancer in breast cytology images as an IoMT application. Future Generation Computer Systems, 98(7), 286–296. https://doi.org/10.1016/j.future.2019.01.033 [Google Scholar] [CrossRef]
30. Mitton, N., Simplot-Ryl, D. (2011). From the Internet of Things to the internet of the physical world. Comptes Rendus Physique, 12(7), 669–674. https://doi.org/10.1016/j.crhy.2011.06.006 [Google Scholar] [CrossRef]
31. Li, B., Yu, J. (2011). Research and application on the smart home based on component technologies and Internet of Things. Procedia Engineering, 15(7), 2087–2092. https://doi.org/10.1016/j.proeng.2011.08.390 [Google Scholar] [CrossRef]
32. Miorandi, D., Sicari, S., de Pellegrini, F., Chlamtac, I. (2012). Internet of Things: Vision, applications and research challenges. Ad Hoc Networks, 10(7), 1497–1516. https://doi.org/10.1016/j.adhoc.2012.02.016 [Google Scholar] [CrossRef]
33. Domingo, M. (2012). An overview of the Internet of Things for people with disabilities. Journal of Network and Computer Applications, 35(2), 584–596. https://doi.org/10.1016/j.jnca.2011.10.015 [Google Scholar] [CrossRef]
34. Domingo, M. (2012). An overview of the internet of underwater things. Journal of Network and Computer Applications, 35(6), 1879–1890. https://doi.org/10.1016/j.jnca.2012.07.012 [Google Scholar] [CrossRef]
35. Corredor, I., Martínez, J. F., Familiar, M. S., López, L. (2012). Knowledge-aware and service-oriented middleware for deploying pervasive services. Journal of Network and Computer Applications, 35(2), 562–576. https://doi.org/10.1016/j.jnca.2011.05.009 [Google Scholar] [CrossRef]
36. Sung, W. T., Tsai, M. H. (2012). Data fusion of multi-sensor for IOT precise measurement based on improved PSO algorithms. Computers and Mathematics with Applications, 64(5), 1450–1461. https://doi.org/10.1016/j.camwa.2012.03.092 [Google Scholar] [CrossRef]
37. Grieco, L. A., Rizzo, A., Colucci, S., Sicari, S., Piro, G. et al. (2014). IoT-aided robotics applications: Technological implications, target domains and open issues. Computer Communications, 54(4), 32–47. https://doi.org/10.1016/j.comcom.2014.07.013 [Google Scholar] [CrossRef]
38. Borgia, E. (2014). The Internet of Things vision: Key features, applications and open issues. Computer Communications, 54(1), 1–31. https://doi.org/10.1016/j.comcom.2014.09.008 [Google Scholar] [CrossRef]
39. Almadani, B., Bin-Yahya, M., Shakshuki, E. M. (2015). E-AMBULANCE: Real-time integration platform for heterogeneous medical telemetry system. Procedia Computer Science, 63(1), 400–407. https://doi.org/10.1016/j.procs.2015.08.359 [Google Scholar] [CrossRef]
40. Wcislik, M., Pozoga, M., Smerdzynski, P. (2015). Wireless health monitoring system. IFAC-PapersOnLine, 48(4), 312–317. https://doi.org/10.1016/j.ifacol.2015.07.053 [Google Scholar] [CrossRef]
41. Hudson, F. D., Nichols, E. W. (2016). The Internet of Things and cognitive computing. In: Handbook of statistics, 1st edition, vol. 35, pp. 341–373. USA: Elsevier. https://doi.org/10.1016/bs.host.2016.07.009 [Google Scholar] [CrossRef]
42. Hossain, M. S., Muhammad, G. (2016). Cloud-assisted Industrial Internet of Things (IIoT)–Enabled framework for health monitoring. Computer Networks, 101(6), 192–202. https://doi.org/10.1016/j.comnet.2016.01.009 [Google Scholar] [CrossRef]
43. Sahney, R. (2016). Smart OPD framework–new era in the digital healthcare initiative of Sir Ganga Ram Hospital. Current Medicine Research and Practice, 6(5), 204–207. https://doi.org/10.1016/j.cmrp.2016.09.011 [Google Scholar] [CrossRef]
44. Qi, J., Yang, P., Min, G., Amft, O., Dong, F. et al. (2017). Advanced Internet of Things for personalised healthcare systems: A survey. Pervasive and Mobile Computing, 41(12), 132–149. https://doi.org/10.1016/j.pmcj.2017.06.018 [Google Scholar] [CrossRef]
45. Srivastava, J., Routray, S., Ahmad, S., Waris, M. M. (2022). Internet of Medical Things (IoMT)-based smart healthcare system: Trends and progress. Computational Intelligence and Neuroscience, 2022(4), 7218113–7218117. https://doi.org/10.1155/2022/7218113 [Google Scholar] [PubMed] [CrossRef]
46. Bhatia, M., Sood, S. K. (2017). A comprehensive health assessment framework to facilitate IoT-assisted smart workouts: A predictive healthcare perspective. Computers in Industry, 92–93(7), 50–66. https://doi.org/10.1016/j.compind.2017.06.009 [Google Scholar] [CrossRef]
47. Gkouskos, D., Burgos, J. (2017). I’m in! Towards participatory healthcare of elderly through IOT. Procedia Computer Science, 113, 647–652. https://doi.org/10.1016/j.procs.2017.08.325 [Google Scholar] [CrossRef]
48. Qi, J., Yang, P., Waraich, A., Deng, Z., Zhao, Y. et al. (2018). Examining sensor-based physical activity recognition and monitoring for healthcare using Internet of Things: A systematic review. Journal of Biomedical Informatics, 87(2), 138–153. https://doi.org/10.1016/j.jbi.2018.09.002 [Google Scholar] [PubMed] [CrossRef]
49. Verma, P., Sood, S. K. (2018). Cloud-centric IoT based disease diagnosis healthcare framework. Journal of Parallel and Distributed Computing, 116(15), 27–38. https://doi.org/10.1016/j.jpdc.2017.11.018 [Google Scholar] [CrossRef]
50. Woo, M. W., Lee, J. W., Park, K. H. (2018). A reliable IoT system for personal healthcare devices. Future Generation Computer Systems, 78(3), 626–640. https://doi.org/10.1016/j.future.2017.04.004 [Google Scholar] [CrossRef]
51. Badr, S., Gomaa, I., Abd-Elrahman, E. (2018). Multi-tier blockchain framework for IoT-EHRs systems. Procedia Computer Science, 141, 159–166. https://doi.org/10.1016/j.procs.2018.10.162 [Google Scholar] [CrossRef]
52. Azimi, I., Pahikkala, T., Rahmani, A. M., Niela-Vilén, H., Axelin, A. et al. (2019). Missing data resilient decision-making for healthcare IoT through personalization: A case study on maternal health. Future Generation Computer Systems, 96(15), 297–308. https://doi.org/10.1016/j.future.2019.02.015 [Google Scholar] [CrossRef]
53. Debauche, O., Mahmoudi, S., Manneback, P., Assila, A. (2019). Fog IoT for health: A new architecture for patients and elderly monitoring. Procedia Computer Science, 160(2), 289–297. https://doi.org/10.1016/j.procs.2019.11.087 [Google Scholar] [CrossRef]
54. Aladwani, T. (2019). Scheduling IoT healthcare tasks in fog computing based on their importance. Procedia Computer Science, 163(3), 560–569. https://doi.org/10.1016/j.procs.2019.12.138 [Google Scholar] [CrossRef]
55. Al-Makhadmeh, Z., Tolba, A. (2019). Utilizing IoT wearable medical device for heart disease prediction using higher order Boltzmann model: A classification approach. Measurement, 147(2), 106815. https://doi.org/10.1016/j.measurement.2019.07.043 [Google Scholar] [CrossRef]
56. Tuli, S., Basumatary, N., Gill, S. S., Kahani, M., Arya, R. C. et al. (2020). HealthFog: An ensemble deep learning based smart healthcare system for automatic diagnosis of heart diseases in integrated IoT and fog computing environments. Future Generation Computer Systems, 104(11), 187–200. https://doi.org/10.1016/j.future.2019.10.043 [Google Scholar] [CrossRef]
57. Khan, S. R., Sikandar, M., Almogren, A., Din, I. U., Guerrieri, A. et al. (2020). IoMT-based computational approach for detecting brain tumor. Future Generation Computer Systems, 109(12), 360–367. https://doi.org/10.1016/j.future.2020.03.054 [Google Scholar] [CrossRef]
58. Manikandan, R., Patan, R., Gandomi, A. H., Sivanesan, P., Kalyanaraman, H. (2020). Hash polynomial two factor decision tree using IoT for smart health care scheduling. Expert Systems with Applications, 141(4), 112924. https://doi.org/10.1016/j.eswa.2019.112924 [Google Scholar] [CrossRef]
59. Alsiddiky, A., Awwad, W., Bakarman, K., Fouad, H., Mahmoud, N. M. (2020). Magnetic resonance imaging evaluation of vertebral tumor prediction using hierarchical hidden Markov random field model on Internet of Medical Things (IOMT) platform. Measurement, 159(4), 107772. https://doi.org/10.1016/j.measurement.2020.107772 [Google Scholar] [CrossRef]
60. Xuan, W., You, G. (2020). Detection and diagnosis of pancreatic tumor using deep learning-based hierarchical convolutional neural network on the Internet of Medical Things platform. Future Generation Computer Systems, 111(22), 132–142. https://doi.org/10.1016/j.future.2020.04.037 [Google Scholar] [CrossRef]
61. Zhu, H., Podesva, P., Liu, X., Zhang, H., Teply, T. et al. (2020). IoT PCR for pandemic disease detection and its spread monitoring. Sensors and Actuators Part B: Chemical, 303, 127098. https://doi.org/10.1016/j.snb.2019.127098 [Google Scholar] [PubMed] [CrossRef]
62. Jamil, M. S., Jamil, M. A., Mazhar, A., Ikram, A., Ahmed, A. et al. (2015). Smart environment monitoring system by employing wireless sensor networks on vehicles for pollution free smart cities. Procedia Engineering, 107(2), 480–484. https://doi.org/10.1016/j.proeng.2015.06.106 [Google Scholar] [CrossRef]
63. Lamprinakos, G. C., Asanin, S., Broden, T., Prestileo, A., Fursse, J. et al. (2015). An integrated remote monitoring platform towards telehealth and telecare services interoperability. Information Sciences, 308(1), 23–37. https://doi.org/10.1016/j.ins.2015.02.032 [Google Scholar] [CrossRef]
64. Syed, L., Jabeen, S., Manimala, S., Alsaeedi, A. (2019). Smart healthcare framework for ambient assisted living using IoMT and big data analytics techniques. Future Generation Computer Systems, 101(3), 136–151. https://doi.org/10.1016/j.future.2019.06.004 [Google Scholar] [CrossRef]
65. Mujawar, M. A., Gohel, H., Bhardwaj, S. K., Srinivasan, S., Hickman, N. et al. (2020). Nano-enabled biosensing systems for intelligent healthcare. Materials Today Chemistry, 17, 100306. https://doi.org/10.1016/j.mtchem.2020.100306 [Google Scholar] [PubMed] [CrossRef]
66. Reid, R. C., Mahbub, I. (2020). Wearable self-powered biosensors. Current Opinion in Electrochemistry, 19(8), 55–62. https://doi.org/10.1016/j.coelec.2019.10.002 [Google Scholar] [CrossRef]
67. Pateraki, M., Fysarakis, K., Sakkalis, V., Spanoudakis, G., Varlamis, I. et al. (2020). Biosensors and internet of things in smart healthcare applications: Challenges and opportunities. Wearable and Implantable Medical Devices, 7, 25–53. https://doi.org/10.1016/b978-0-12-815369-7.00002-1 [Google Scholar] [CrossRef]
68. Mutlag, A. A., Abd Ghani, M. K., Arunkumar, N., Mohammed, M. A., Mohd, O. (2019). Enabling technologies for fog computing in healthcare IoT systems. Future Generation Computer Systems, 90(3), 62–78. https://doi.org/10.1016/j.future.2018.07.049 [Google Scholar] [CrossRef]
69. Dressler, F., Fischer, S. (2015). Connecting in-body nano communication with body area networks: Challenges and opportunities of the Internet of Nano Things. Nano Communication Networks, 6(2), 29–38. https://doi.org/10.1016/j.nancom.2015.01.006 [Google Scholar] [CrossRef]
70. Santos, J., Rodrigues, J. J. P. C., Silva, B. M. C., Casal, J., Saleem, K. et al. (2016). An IoT-based mobile gateway for intelligent personal assistants on mobile health environments. Journal of Network and Computer Applications, 71(2), 194–204. https://doi.org/10.1016/j.jnca.2016.03.014 [Google Scholar] [CrossRef]
71. Lou, D., Chen, X., Zhao, Z., Xuan, Y., Xu, Z. et al. (2013). A wireless health monitoring system based on android operating system. IERI Procedia, 4, 208–215. https://doi.org/10.1016/j.ieri.2013.11.030 [Google Scholar] [CrossRef]
72. Mills, G. (2012). Wireless digital stethoscope using bluetooth technology. International Journal of Engineering, Science and Technology, 4(8), 3961–3969. [Google Scholar]
73. Patil, K. (2012). Design of wireless electronic stethoscope based on Zigbee. International Journal of Distributed and Parallel Systems, 3(1), 351–359. https://doi.org/10.5121/ijdps.2012.3130 [Google Scholar] [CrossRef]
74. Huida, L., Bingchen, F., Liheng, Y., Yanan, L. (2012). RFID-based hazardous waste management platform establishment. Procedia Engineering, 29(17), 4–8. https://doi.org/10.1016/j.proeng.2011.12.658 [Google Scholar] [CrossRef]
75. Rhayem, A., Ahmed Mhiri, M. B., Salah, M. B., Gargouri, F. (2017). Ontology-based system for patient monitoring with connected objects. Procedia Computer Science, 112(5), 683–692. https://doi.org/10.1016/j.procs.2017.08.127 [Google Scholar] [CrossRef]
76. Singh, R. P., Javaid, M., Haleem, A., Suman, R. (2020). Internet of Things (IoT) applications to fight against COVID-19 pandemic. Diabetes and Metabolic Syndrome, 14(4), 521–524. https://doi.org/10.1016/j.dsx.2020.04.041 [Google Scholar] [PubMed] [CrossRef]
77. Swayamsiddha, S., Mohanty, C. (2020). Application of cognitive Internet of Medical Things for COVID-19 pandemic. Diabetes and Metabolic Syndrome, 14(5), 911–915. https://doi.org/10.1016/j.dsx.2020.06.014 [Google Scholar] [PubMed] [CrossRef]
78. Ashraf, Q. M., Habaebi, M. H. (2015). Autonomic schemes for threat mitigation in Internet of Things. Journal of Network and Computer Applications, 49(2), 112–127. https://doi.org/10.1016/j.jnca.2014.11.011 [Google Scholar] [CrossRef]
79. Tian, S., Yang, W., Le Grange, J. M. L., Wang, P., Huang, W. et al. (2019). Smart healthcare: Making medical care more intelligent. Global Health Journal, 3(3), 62–65. https://doi.org/10.1016/j.glohj.2019.07.001 [Google Scholar] [CrossRef]
80. Yaacoub, J. A., Noura, M., Noura, H. N., Salman, O., Yaacoub, E. et al. (2020). Securing Internet of Medical Things systems: Limitations, issues and recommendations. Future Generation Computer Systems, 105(10), 581–606. https://doi.org/10.1016/j.future.2019.12.028 [Google Scholar] [CrossRef]
81. Mansfield-Devine, S. (2016). Securing the Internet of Things. Computer Fraud and Security, 2016(4), 15–20. https://doi.org/10.1016/S1361-3723(16)30038-0 [Google Scholar] [CrossRef]
82. Patan, R., Pradeep Ghantasala, G. S. P., Sekaran, R., Gupta, D., Ramachandran, M. (2020). Smart healthcare and quality of service in IoT using grey filter convolutional based cyber physical system. Sustainable Cities and Society, 59, 102141. https://doi.org/10.1016/j.scs.2020.102141 [Google Scholar] [CrossRef]
83. Lee, J. G., Kim, S. W., Kim, D., Cho, Y., Rieh, J. et al. (2015). D2ART: Direct Data Accessing from Passive RFID Tag for infra-less, contact-less, and battery-less pervasive computing. Microprocessors and Microsystems, 39(8), 767–781. https://doi.org/10.1016/j.micpro.2015.09.007 [Google Scholar] [CrossRef]
84. Magno, M., Marinkovic, S., Srbinovski, B., Popovici, E. M. (2014). Wake-up radio receiver based power minimization techniques for wireless sensor networks: A review. Microelectronics Journal, 45(12), 1627–1633. https://doi.org/10.1016/j.mejo.2014.08.010 [Google Scholar] [CrossRef]
85. Talaminos-Barroso, A., Estudillo-Valderrama, M. A., Roa, L. M., Reina-Tosina, J., Ortega-Ruiz, F. (2016). A machine-to-machine protocol benchmark for eHealth applications–Use case: Respiratory rehabilitation. Computer Methods and Programs in Biomedicine, 129, 1–11. https://doi.org/10.1016/j.cmpb.2016.03.004 [Google Scholar] [PubMed] [CrossRef]
86. Harish, N., Mandal, S., Rao, S., Patil, S. G. (2015). Particle Swarm Optimization based support vector machine for damage level prediction of non-reshaped berm breakwater. Applied Soft Computing, 27(10), 313–321. https://doi.org/10.1016/j.asoc.2014.10.041 [Google Scholar] [CrossRef]
87. Rasitha, G. (2016). Predicting thyroid disease using linear discriminant analysis (LDA) data mining technique. Communications on Applied Electronics, 4(1), 4–6. https://doi.org/10.5120/cae2016651990 [Google Scholar] [CrossRef]
88. Vijayarani, S., Dhayanand, S. (2015). Liver disease rediction using SVM and Naïve Bayes algorithms. International Journal of Science, Engineering and Technology Research (IJSETR), 4(4), 816–820. [Google Scholar]
89. Masethe, H. D., Masethe, M. A. (2014). Prediction of heart disease using classification algorithms. In: Lecture notes in engineering and computer science, vol. 2, pp. 809–812. [Google Scholar]
90. Vellappally, S., Al Kheraif, A. A. Al., Anil, A., Wahba, A. A. (2019). IoT medical tooth mounted sensor for monitoring teeth and food level using bacterial optimization along with adaptive deep learning neural network. Measurement, 135(1), 672–677. https://doi.org/10.1016/j.measurement.2018.11.078 [Google Scholar] [CrossRef]
91. Collotta, M., Pau, G. (2015). Bluetooth for Internet of Things: A fuzzy approach to improve power management in smart homes. Computers and Electrical Engineering, 44(15), 137–152. https://doi.org/10.1016/j.compeleceng.2015.01.005 [Google Scholar] [CrossRef]
92. Mehmood, R., Graham, G. (2015). Big data logistics: A healthcare transport capacity sharing model. Procedia Computer Science, 64, 1107–1114. https://doi.org/10.1016/j.procs.2015.08.566 [Google Scholar] [CrossRef]
93. Saheb, T., Izadi, L. (2019). Paradigm of IoT big data analytics in the healthcare industry: A review of scientific literature and mapping of research trends. Telematics and Informatics, 41, 70–85. https://doi.org/10.1016/j.tele.2019.03.005 [Google Scholar] [CrossRef]
94. Aceto, G., Persico, V., Pescapé, A. (2020). Industry 4.0 and health: Internet of Things, bigdata, and cloud omputing for Healthcare 4.0. Journal of Industrial Information Integration, 18(1), 100129. https://doi.org/10.1016/j.jii.2020.100129 [Google Scholar] [CrossRef]
95. Hathaliya, J. J., Tanwar, S. (2020). An exhaustive survey on security and privacy issues in Healthcare 4.0. Computer Communications, 153, 311–335. https://doi.org/10.1016/j.comcom.2020.02.018 [Google Scholar] [CrossRef]
96. Alatoun, K., Matrouk, K., Mohammed, M. A., Nedoma, J., Martinek, R. et al. (2022). A novel low-latency and energy-efficient task scheduling framework for Internet of Medical Things in an edge fog cloud system. Sensors, 22(14), 5327. https://doi.org/10.3390/s22145327 [Google Scholar] [PubMed] [CrossRef]
97. Hameed Abdulkareem, K., Awad Mutlag, A., Musa Dinar, A., Frnda, J., Abed Mohammed, M. et al. (2022). Smart healthcare system for severity prediction and critical tasks management of COVID-19 patients in IoT-fog computing environments. Computational Intelligence and Neuroscience, 2022(2), 5012962. https://doi.org/10.1155/2022/5012962 [Google Scholar] [PubMed] [CrossRef]
98. Razdan, S., Sharma, S. (2022). Internet of Medical Things (IoMTOverview, emerging technologies, and case studies. IETE Technical Review, 39(4), 775–788. https://doi.org/10.1080/02564602.2021.1927863 [Google Scholar] [CrossRef]
99. Ghazal, T. M., Hasan, M. K., Abdullah, S. N. H., Abubakkar, K. A., Afifi, M. A. (2022). IoMT-enabled fusion-based model to predict posture for smart healthcare systems. Computers, Materials & Continua, 71(2), 2579–2597. https://doi.org/10.32604/cmc.2022.019706 [Google Scholar] [CrossRef]
100. Koutras, D., Stergiopoulos, G., Dasaklis, T., Kotzanikolaou, P., Glynos, D. et al. (2020). Security in IoMT communications: A survey. Sensors, 20(17), 4828. https://doi.org/10.3390/s20174828 [Google Scholar] [PubMed] [CrossRef]
101. Islam, M. K., Kaushal, C., Amin, M. A., Algarni, A. D., Alturki, N. et al. (2022). A secure framework toward IoMT-assisted data collection, modeling, and classification for intelligent dermatology healthcare services. Contrast Media and Molecular Imaging, 2022(2), 6805460. https://doi.org/10.1155/2022/6805460 [Google Scholar] [PubMed] [CrossRef]
102. Mansour, R. F., Parah, S. A. (2021). Reversible data hiding for electronic patient information security for telemedicine applications. Arabian Journal for Science and Engineering, 46(9), 9129–9144. https://doi.org/10.1007/s13369-021-05716-2 [Google Scholar] [CrossRef]
103. Chen, C., Liu, S., Ashraf Chaudhry S., Chen Y., Asghar khan, M. (2022). A lightweight and robust user authentication protocol with user anonymity for IoT-based healthcare. Computer Modeling in Engineering & Sciences, 131(1), 307–329. https://doi.org/10.32604/cmes.2022.018749 [Google Scholar] [CrossRef]
104. Seh, A. H., Al-Amri, J. F., Subahi, A. F., Agrawal, A., Pathak, N. et al. (2022). An analysis of integrating machine learning in healthcare for ensuring confidentiality of the electronic records. Computer Modeling in Engineering & Sciences, 130(3), 1387–1422. https://doi.org/10.32604/cmes.2022.018163 [Google Scholar] [CrossRef]
105. Gu, X., Chen, S., Zhu, H., Brown, M. (2022). COVID-19 imaging detection in the context of artificial intelligence and the Internet of Things. Computer Modeling in Engineering & Sciences, 132(2), 507–530. https://doi.org/10.32604/cmes.2022.018948 [Google Scholar] [CrossRef]
Cite This Article
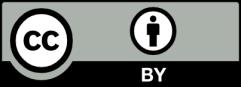