Open Access
ARTICLE
Deep Learning Approach for Automatic Cardiovascular Disease Prediction Employing ECG Signals
1
Department of Computer Science & Information Technology, The Islamia University of Bahawalpur, Bahawalpur, 63100, Pakistan
2
Department of Computer Engineering, College of Computer Engineering and Sciences, Prince Sattam Bin Abdulaziz University,
Al-Kharj, 11942, Saudi Arabia
3
Department of Computer Science, Khwaja Fareed University of Engineering and Information Technology, Rahim Yar Khan,
Pakistan
4
Department of Cybersecurity, College of Computer Science and Engineering, University of Jeddah, Jeddah, 21959, Saudi Arabia
5
Faculty of Computer Science and Information Technology, Universiti Tun Husein Onn Malaysia (UTHM), Bahru, 80536, Malaysia
6
Research Centre, Future University in Egypt, New Cairo, 11745, Egypt
7
Department of Information Systems, University of Maryland, Baltimore County, Baltimore, MD 21250, USA
8
Department of Information and Communication Engineering, Yeungnam University, Gyeongsan-si, 38541, Korea
* Corresponding Authors: Imran Ashraf. Email: ;
(This article belongs to the Special Issue: Bio-inspired Computer Modelling: Theories and Applications in Engineering and Sciences)
Computer Modeling in Engineering & Sciences 2023, 137(2), 1677-1694. https://doi.org/10.32604/cmes.2023.026535
Received 10 September 2022; Accepted 08 February 2023; Issue published 26 June 2023
Abstract
Cardiovascular problems have become the predominant cause of death worldwide and a rise in the number of patients has been observed lately. Currently, electrocardiogram (ECG) data is analyzed by medical experts to determine the cardiac abnormality, which is time-consuming. In addition, the diagnosis requires experienced medical experts and is error-prone. However, automated identification of cardiovascular disease using ECGs is a challenging problem and state-of-the-art performance has been attained by complex deep learning architectures. This study proposes a simple multilayer perceptron (MLP) model for heart disease prediction to reduce computational complexity. ECG dataset containing averaged signals with window size 10 is used as an input. Several competing deep learning and machine learning models are used for comparison. K-fold cross-validation is used to validate the results. Experimental outcomes reveal that the MLP-based architecture can produce better outcomes than existing approaches with a 94.40% accuracy score. The findings of this study show that the proposed system achieves high performance indicating that it has the potential for deployment in a real-world, practical medical environment.Keywords
Cite This Article
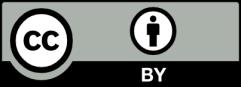
This work is licensed under a Creative Commons Attribution 4.0 International License , which permits unrestricted use, distribution, and reproduction in any medium, provided the original work is properly cited.