Open Access
ARTICLE
Outage Behaviors of Active Intelligent Reflecting Surface Enabled NOMA Communications
1 School of Information and Communication Engineering, Beijing University of Posts and Telecommunications,
State Key Laboratory of Wireless Mobile Communications, China Academy of Telecommunications Technology (CATT), Beijing, China
2 School of Information and Communication Engineering, Beijing Information Science and Technology University, Beijing, 100101, China
* Corresponding Author: Xinwei Yue. Email:
(This article belongs to the Special Issue: Recent Advances in Backscatter and Intelligent Reflecting Surface Communications for 6G-enabled Internet of Things Networks)
Computer Modeling in Engineering & Sciences 2023, 137(1), 789-812. https://doi.org/10.32604/cmes.2023.027663
Received 08 November 2022; Accepted 16 December 2022; Issue published 23 April 2023
Abstract
Active intelligent reflecting surface (IRS) is a novel and promising technology that is able to overcome the multiplicative fading introduced by passive IRS. In this paper, we consider the application of active IRS to non-orthogonal multiple access (NOMA) networks, where the incident signals are amplified actively through integrating amplifier to reflecting elements. More specifically, the performance of active/passive IRS-NOMA networks is investigated over large and small-scale fading channels. Aiming to characterize the performance of active IRS-NOMA networks, the exact and asymptotic expressions of outage probability for a couple of users, i.e., near-end user and far-end user are derived by exploiting a 1-bit coding scheme. Based on approximated analyses, the diversity orders of user and user are obtained for active IRS-NOMA. In addition, the system throughput of active IRS-NOMA is discussed in the delay-sensitive transmission. The simulation results are carried out to verify that: i) The outage behaviors of active IRS-NOMA networks are superior to that of passive IRS-NOMA networks; ii) As the reflection amplitude factors increase, the active IRS-NOMA networks are capable of furnishing the enhanced outage performance; and iii) The active IRS-NOMA has a larger system throughput than passive IRS-NOMA and conventional communications.Keywords
With the commercialization of the fifth-generation networks, both academia and industry are focusing on the design of the sixth-generation (6G) networks. It will create tremendous research possibilities and enable many new technologies. Compared to the previous wireless communication systems, 6G networks need to take into account the higher performance metrics and are expected to support huge connectivity, high data rates and various heterogeneous communication scenarios [1,2]. Non-orthogonal multiple access (NOMA) has been regarded as one of the promising technologies, which is able to improve spectral efficiency, connectivity and user fairness by encouraging sharing the same time/frequence/code/space resource among plenty of users [3–5]. It has been shown that when the non-orthogonal users have disparate channels to the base station (BS), NOMA is capable of offering better performance gain relative to orthogonal multiple access (OMA) [6–8].
In parallel with the development of NOMA, intelligent reflecting surface (IRS) assisted wireless communications have received a lot of attention due to their superior performance in creating favourable radio propagation environments [9–11]. The basic components of IRS include plenty of low-cost passive reflecting elements, which can independently change the amplitude and phase of incident signals to provide a new degree of freedom. Hence this creates the possibility of realizing a programmable and smart radio communication environments. The continuous deepening researches of passive IRS find that the received signals may suffer from multiplicative fading or double path-loss attenuation [12,13], i.e., the product of path losses from the BS to IRS, and then from IRS to the desired users. To solve the effect of multiplicative fading on system performance, the IRS can be equipped with reflecting amplification elements, such as current-inverting converters and tunnel diode to amplify the reflected signals. The basic approach of designing a reflecting power-amplifying surface was highlighted in [14], where the stability of power amplification was safeguarded by minimizing the nonreciprocal response of the reflecting surface. Moreover, the authors of [15] proposed the concept of active IRS to overcome the multiplicative fading influence, and confirmed that active IRSs are able to achieve the enhanced sum rate gain relative to passive IRS. For the purpose of saving power consumption, the authors in [16] further outlined the sub-connected architecture of active IRS, in which numerous reflecting elements share the integrated power amplifier. The research progresses of NOMA, IRS and IRS assisted NOMA communications are surveyed comprehensively in the following paragraphs.
1.1 Studies on Cooperative NOMA Communications
Many applications of NOMA have been developed to meet the requirements of different communications scenarios. The cooperative NOMA can be categorized into two major types. The first type is that the near-end user with better channel gains is selected as decode-and-forward (DF) or amplify-and-forward (AF) relaying to forward the information. For example, the authors of [17] put forward a cooperative NOMA scheme to achieve the maximum diversity order of users. In [18], the outage probability of energy harvesting based cooperative NOMA was investigated by invoking stochastic geometry. Furthermore, the authors of [19] regarded the near-end user with better channels as decode-and-forward (DF) relaying, which can switch between half-duplex (HD) and full-duplex (FD) modes. Inspired by user collaboration, the integration of NOMA to cognitive radio networks was surveyed in [20], where the secondary users served as HD DF relays to improve the multicast performance. To further enhance the spectrum efficiency, the authors of [21] researched the outage probability of FD device-to-device cooperative NOMA systems. The second type is that the dedicated DF or AF relaying is introduced into NOMA networks to help the BS. In [22], the authors exploited an FD DF relaying based two-way cooperative NOMA system to exchange the information between a pair of users. The ergodic sum rate of AF based cooperative NOMA networks was studied over Nakagami-
1.2 Studies on IRS Assisted Wireless Communications
In contrast with conventional wireless communications, the channel coefficients between the BS and desired users can be adjusted constructively by deploying the IRS for wireless communications [26–28]. From the viewpoint of energy consumption, the authors of [29] revealed the design of energy efficiency for passive IRS aided wireless systems. In [30], the outage probability of passive IRS for wireless communications was studied by employing coherent phase shifting scheme. By emphasizing the performance limits, the authors of [31] researched the achievable rate of passive IRS with sensitive phase shifting. Afterward, the coverage probability of passive IRS aided wireless systems was evaluated in [32] over the real fading channels. To meet diverse requirements, the authors of [33] studied the symbol error probability of single passive IRS in detail. The system performance of passive IRS is usually constrained by the double fading influence, i.e., since the incident signals undergo the cascaded channels of BS-IRS, and then IRS-users. Hence it is necessary to solve this influence introduced by passive IRS. Apart from the aforementioned passive IRS, the growing research interests have been devoted to examine active IRS for overcoming the multiplicative fading [34–36]. The application of active IRS into wireless networks was outlined [34] to balance conflict between the amplification noise and the received signal power at the receiver. The superior of active IRS is able to amplify the incident signals and overcome the double-fading attenuation. The authors of [35] discussed the deployment issues of active IRS and showed that with the IRS’s decreasing amplification power, the active IRS should be deployed closer to the desired users. Conditioned on the small power budget [36], the active IRS assisted wireless communication was capable of supplying the better achievable rate through rational power allocation. It was found in [37] that active IRS not only improves energy efficiency greatly, but also extends transmission coverage with respect to passive IRS.
1.3 Studies on IRS Assisted NOMA Communications
In light of the above discussions, both NOMA and IRS are essential enabling techniques of 6G networks, which are complementary to each other [38,39]. In [40], the closed-form expressions for the outage probability of passive IRS assisted NOMA were derived by designing the passive beamforming weights. To shed light on phase shifting, the authors of [41] analyzed the effect of coherent phase shifting and random phase shifting on active IRS-NOMA networks. Sparked by this work, the outage probability and ergodic rate of passive IRS-NOMA were evaluated by using 1-bit coding scheme [42]. The authors in [43] paid attention to wireless power transfer based NOMA with the aid of the passive IRS. Exploiting the direct links between the BS and users [44], the authors further studied the sum throughput of passive IRS-NOMA networks. The outage probability of NOMA with passive IRS partitioning was evaluated in [45], which denotes the superior of the proposed system over IRS-OMA systems in terms of outage behaviors. Moving forward a single step, the authors of [46] researched the outage performance of multiple passive IRS aided NOMA networks with the use of discrete phase shifts. In [47], the authors outlined the network details of passive IRS aided two-way NOMA, where the outage probability of a pair of users was derived exhaustively. With the emphasis on covert communications, the authors in [48] studied the average error probability of IRS-NOMA networks. In addition, the performance of passive IRS-NOMA was discussed by taking into consideration imperfect successive interference cancellation (SIC) [49].
1.4 Motivation and Contributions
While the before-mentioned enjoyable contributions have laid a substantial foundation for finding out the diverse aspects of passive IRS assisted NOMA networks, the active IRS enabled NOMA communications for 6G networks are far from being well comprehended. It is worth noting that the performance of passive IRS assisted wireless networks may be restricted by its high multiplicative fading. This phenomenon can be alleviated by active IRS equipped with multiple reflecting amplification elements [15], which is able to amplify the incident signals with low cost negative impedance converter or asymmetric current mirrors. At this moment, the fewer reflecting element is required to attain the enhanced performance for users. In contrast to the gain introduced by passive IRS with a large number of elements, the advantages of active IRS with power amplification are more efficient and conspicuous. In [50], two typical active aided multiple access schemes, i.e., time division multiple access and NOMA were surveyed, where the achievable sum throughput was only compared each other from the perspective of system optimization. To the best of our knowledge, the performance analysis of active IRS enabled NOMA networks is not researched yet. Motivated by these, we simultaneously characterize the performance of active/passive IRS-NOMA networks in terms of outage probability and system throughput. The staple issue for active IRS-NOMA are further handled that how amplification noise affects the system performance and how many performance gains can be brought over conventional OMA and cooperative communication schemes. Based on the these explanations, the principal contributions of this manuscript are summarized as follows:
1. We derive the exact expressions of outage probability for user
2. We simultaneously derive the exact and asymptotic expressions of outage probability for user
3. We confirm that the outage probability of user
1.5 Organization and Notations
The rest of manuscript is organized as below. Section 1 establishes the system model of active RIS enabled NOMA networks; In Section 2, the outage probabilities of a couple of users are analysed and the commensurable high SNR approximated results are provided at length; The computer simulation and analytical discussions are presented in Section 3 to illustrate remarks; Immediately after, the Section 4 gives a summary of this manuscript.
The principal notations in this manuscript are shown as follows. The superscript
Consider an active IRS enabled NOMA communication scenarios, as illustrated in Fig. 1, where the BS sends the superposed signals to M users with the help of active IRS. The BS and non-orthogonal users are equipped single antenna, while the IRS consists of K active reflecting amplification elements. The IRS is capable of dynamically adjusting the amplitude and phase shifting of each active element through a smart controller. Compared to the passive IRS that just reflects the signals without amplification, active IRS is able to amplify the reflected signals with the additionally active reflection-type amplifier, which can be accomplished by some integrated circuits1. The direct links between the BS and non-orthogonal users are supposed strongly attenuated due to surrounding physical obstacles [41,42]. The assumption can reflect the role of IRS’s deployment between the BS and users. All wireless communication links in the networks are supposed to experience identically and independent Rayleigh fading. Define the phase shift matrix of active IRS as
Figure 1: System model of active IRS enabled NOMA networks
In conformity with the superposition coding principle, the BS broadcasts the superposed signals to non-orthogonal users with the assistance of active IRS. At this moment, the received signals of user
where
For the user
where
For the user
2.2 Active IRS-NOMA with 1-bit Coding
Different to the coherent phase shifting, 1-bit coding scheme is a special case of random phase shifting scheme, which can be easily applicable in practice and avoid the excessive system overhead [41,42]. More specifically, the elements of diagonal matrix
and
From the perspective of fair comparison, the active IRS enabled OMA networks are seemed as a benchmark, where the active IRS is deployed between the BS and orthogonal user, i.e., user
where
In this section, the performance of active IRS-NOMA networks is characterized in terms of outage probability and system throughput. As a further development, the asymptotic outage probabilities for a pair of users are derived in the following part.
3.1 The Outage Probability of User
The SIC scheme is firstly carried out at user
where
Proof. See Appendix A.
As a further advance, the following corollary provides the outage probability of user
3.2 The Outage Probability of User m
Different from the outage events of user
Proof. See Appendix B.
Based on the above analytical result, the following corollary provides the outage probability of user
3.3 The Outage Probability of User
In active IRS-OMA networks, if the detecting SINR of user
The closed-form and asymptotic expressions for outage probability of orthogonal user in passivea IRS-OMA networks can be found in [42, Eq. (12), Eq. (19), Eq. (20)], which are regarded as a baseline from the comparison point of view.
To gain the tractable insights, we provide the diversity orders of user
where
To calculate the asymptotic outage probability of user
For the special case with
and
on the condition of
Similar to the calculation processes of user
and
with the cases
In this subsection, the system throughput of active IRS-NOMA networks is taken into account in delay-sensitive transmission. At this mode, the delay-sensitive throughput system mainly depend on the influence of outage probability. According to the derived outage probability of user
where
In this section, computer simulations are carried out to verify the analytical results of active IRS-NOMA networks. The impact of reflection amplitude factor on active IRS enabled NOMA is taken into account in detail. For clarity of exposition, the key simulation parameters used are summarized in Table 1, where the BPCU is abbreviation of bit per channel use. The complexity-accuracy tradeoff parameter W is set to be
Fig. 2 plots the outage probability of active IRS-NOMA networks vs. SNR for a simulation setting with
Figure 2: Outage probability vs. the transmit SNR, with
Fig. 3 plots the outage probability of active IRS-NOMA networks vs. SNR for a simulation setting with
Figure 3: Outage probability vs. the transmit SNR, with
Fig. 4 plots the outage probability of active IRS-NOMA networks vs. SNR for the different reflection amplitude factors with
Figure 4: Outage probability vs. the transmit SNR, with
Fig. 5 plots the outage probability of active IRS-NOMA networks vs. SNR for the different reflection elements with
Figure 5: Outage probability vs. the transmit SNR, with
Figure 6: Outage probability vs. the transmit SNR, with
Fig. 7 plots the outage probability of active IRS-NOMA networks vs. SNR for the different target rates with
Figure 7: Outage probability vs. the transmit SNR, with
Figure 8: Outage probability vs. the transmit SNR, with
Fig. 9 plots the delay-sensitive system throughput of active IRS-NOMA networks vs. SNR for a simulation setting with
Figure 9: The delay-sensitive system throughput vs. the transmit SNR, with
In this paper, the active IRS has been introduced into NOMA networks to help BS forward the incident signals. More specifically, we have investigated the performance of active IRS-NOMA networks by invoking a 1-bit coding scheme. The approximated expressions of outage probability for active/passive IRS-NOMA networks have been derived in detail. Based on these, the diversity orders of user
Funding Statement: This work was supported by the National Natural Science Foundation of China Grant 61901043.
Conflicts of Interest: The authors declare that they have no conflicts of interest to report regarding the present study.
1Note that the amplification function of active IRS’ reflecting elements can be implemented by the current-inverting converters or tunnel diode and so on [51,52].
References
1. You, X., Wang, C., Huang, J. (2021). Towards 6G wireless communication networks: Vision, enabling technologies, and new paradigm shifts. Science China-Information Sciences, 64(1), 110301:1–110301:74. https://doi.org/10.1007/s11432-020-2955-6 [Google Scholar] [CrossRef]
2. Saad, W., Bennis, M., Chen, M. (2020). A vision of 6G wireless systems: Applications, trends, technologies, and open research problems. IEEE Network, 34(3), 134–142. https://doi.org/10.1109/MNET.001.1900287 [Google Scholar] [CrossRef]
3. Ding, Z., Liu, Y., Choi, J., Sun, Q., Elkashlan, M. et al. (2017). Application of non-orthogonal multiple access in LTE and 5G networks. IEEE Communications Magazine, 55(2), 185–191. https://doi.org/10.1109/MCOM.2017.1500657CM [Google Scholar] [CrossRef]
4. Liu, Y., Yi, W., Ding, Z., Liu, X., Dobre. et al. (2021). Application of NOMA in 6G networks: Future vision and research opportunities for next generation multiple access. https://arxiv.org/abs/2103.02334v1 [Google Scholar]
5. Bouzinis, P. S., Diamantoulakis, P. D., Karagiannidis, G. K. (2022). Wireless federated learning WFL for 6G networks-part II: The compute-then-transmit NOMA paradigm. IEEE Communications Letters, 26(1), 8–12. [Google Scholar]
6. Tse, D., Viswanath, P. (2005). Fundamentals of wireless communication. Cambridge, UK: Cambridge University Press. [Google Scholar]
7. Ding, Z., Yang, Z., Fan, P., Poor, H. V. (2014). On the performance of non-orthogonal multiple access in 5G systems with randomly deployed users. IEEE Signal Processing Letters, 21(12), 1501–1505. https://doi.org/10.1109/LSP.2014.2343971 [Google Scholar] [CrossRef]
8. Yue, X., Qin, Z., Liu, Y., Kang, S., Chen, Y. (2018). A unified framework for non-orthogonal multiple access. IEEE Transactions on Communications, 66(11), 5346–5359. https://doi.org/10.1109/TCOMM.2018.2842217 [Google Scholar] [CrossRef]
9. Wu, Q., Zhang, R. (2020). Towards smart and reconfigurable environment: Intelligent reflecting surface aided wireless network. IEEE Transactions on Communications, 58(1), 106–112. [Google Scholar]
10. ElMossallamy, M. A., Zhang, H., Song, L., Seddik, K. G., Han, Z. et al. (2020). Reconfigurable intelligent surfaces for wireless communications: Principles, challenges, and opportunities. IEEE Transactions on Cognitive Communications and Networking, 6(3), 990–1002. https://doi.org/10.1109/TCCN.2020.2992604 [Google Scholar] [CrossRef]
11. Liang, Y. C., Chen, J., Long, R., He, Z., Renzo, M. D. (2021). Reconfigurable intelligent surfaces for smart wireless environments: Channel estimation, system design and applications in 6G networks. Science China-Information Sciences, 64(10), 200301:1–200301:21. https://doi.org/10.1007/s11432-020-3261-5 [Google Scholar] [CrossRef]
12. Najafi, M., Jamali, V., Schober, R., Poor, H. V. (2021). Physics-based modeling and scalable optimization of large intelligent reflecting surfaces. IEEE Transactions on Communications, 69(4), 2673–2691. https://doi.org/10.1109/TCOMM.2020.3047098 [Google Scholar] [CrossRef]
13. Basar, E., Poor, H. V. (2021). Present and future of reconfigurable intelligent surface-empowered communications. IEEE Signal Processing Magazine, 38(6), 146–152. [Google Scholar]
14. Lonǎar, J., Šipuš, Z. (2020). Challenges in design of power-amplifying active metasurfaces. 2020 International Symposium ELMAR. [Google Scholar]
15. Zhang, Z., Dai, L., Chen, X., Liu, C., Yang, F. et al. (2022). Active RIS vs. passive RIS: Which will prevail in 6G? https://arxiv.org/abs/2103.15154v4 [Google Scholar]
16. Liu, K., Zhang, Z., Dai, L., Xu, S., Yang, F. (2022). Active reconfigurable intelligent surface: Fully-connected or sub-connected? IEEE Communications Letters, 26(1), 167–171. https://doi.org/10.1109/LCOMM.2021.3119696 [Google Scholar] [CrossRef]
17. Ding, Z., Peng, M., Poor, H. V. (2015). Cooperative non-orthogonal multiple access in 5G systems. IEEE Communications Letters, 19(8), 1462–1465. https://doi.org/10.1109/LCOMM.2015.2441064 [Google Scholar] [CrossRef]
18. Liu, Y., Ding, Z., Elkashlan, M., Poor, H. V. (2016). Cooperative non-orthogonal multiple access with simultaneous wireless information and power transfer. IEEE Journal on Selected Areas in Communications, 34(4), 938–953. https://doi.org/10.1109/JSAC.2016.2549378 [Google Scholar] [CrossRef]
19. Yue, X., Liu, Y., Kang, S., Nallanathan, A., Ding, Z. (2018). Exploiting full/half-duplex user relaying in NOMA systems. IEEE Transactions on Communications, 66(2), 560–575. https://doi.org/10.1109/TCOMM.2017.2749400 [Google Scholar] [CrossRef]
20. Lv, L., Chen, J., Ni, Q., Ding, Z. (2017). Design of cooperative non-orthogonal multicast cognitive multiple access for 5G systems: User scheduling and performance analysis. IEEE Transactions on Communications, 65(6), 2641–2656. https://doi.org/10.1109/TCOMM.2017.2677942 [Google Scholar] [CrossRef]
21. Zhang, Z., Ma, Z., Xiao, M., Ding, Z., Fan, P. (2017). Full-duplex device-to-device-aided cooperative nonorthogonal multiple access. IEEE Transactions on Vehicular Technology, 66(5), 4467–4471. [Google Scholar]
22. Wang, X., Jia, M., Ho, I. W. H., Guo, Q., Lau, F. C. M. (2019). Exploiting full-duplex two-way relay cooperative non-orthogonal multiple access. IEEE Transactions on Communications, 67(4), 2716–2729. [Google Scholar]
23. Men, J., Ge, J., Zhang, C. (2017). Performance analysis for downlink relaying aided non-orthogonal multiple access networks with imperfect CSI over Nakagami-m fading. IEEE Access, 5, 998–1004. [Google Scholar]
24. Li, X., Li, J., Liu, Y., Ding, Z., Nallanathan, A. (2020). Residual transceiver hardware impairments on cooperative NOMA networks. IEEE Transactions on Wireless Communications, 19(1), 680–695. [Google Scholar]
25. Abbasi, O., Ebrahimi, A., Mokari, N. (2019). Noma inspired cooperative relaying system using an AF relay. IEEE Wireless Communications Letters, 8(1), 261–264. [Google Scholar]
26. ElMossallamy, M. A., Zhang, H., Song, L., Seddik, K. G., Han, Z. et al. (2020). Reconfigurable intelligent surfaces for wireless communications: Principles, challenges, and opportunities. IEEE Transactions on Cognitive Communications and Networking, 6(3), 990–1002. [Google Scholar]
27. Liu, Y., Liu, X., Mu, X., Hou, T., Xu, J. et al. (2021). Reconfigurable intelligent surfaces: Principles and opportunities. IEEE Communications Surveys Tutorials, 23(3), 1546–1577. https://doi.org/10.1109/COMST.2021.3077737 [Google Scholar] [CrossRef]
28. Yang, H., Xiong, Z., Zhao, J., Niyato, D., Wu, Q. et al. (2021). Intelligent reflecting surface assisted anti-jamming communications: A fast reinforcement learning approach. IEEE Transactions on Wireless Communications, 20(3), 1963–1974. https://doi.org/10.1109/TWC.2020.3037767 [Google Scholar] [CrossRef]
29. Huang, C., Zappone, A., Alexandropoulos, G. C., Debbah, M., Yuen, C. (2019). Reconfigurable intelligent surfaces for energy efficiency in wireless communication. IEEE Transactions on Wireless Communications, 18(8), 4157–4170. https://doi.org/10.1109/TWC.2019.2922609 [Google Scholar] [CrossRef]
30. Kudathanthirige, D., Gunasinghe, D., Amarasuriya, G. (2020). Performance analysis of intelligent reflective surfaces for wireless communication. IEEE International Communication Conference, pp. 1–6. Dublin, IRL. [Google Scholar]
31. Zhang, H., Di, B., Song, L., Han, Z. (2020). Reconfigurable intelligent surfaces assisted communications with limited phase shifts: How many phase shifts are enough? IEEE Transactions on Vehicular Technology, 69(4), 4498–4502. https://doi.org/10.1109/TVT.2020.2973073 [Google Scholar] [CrossRef]
32. Cui, Z., Guan, K., Zhang, J., Zhong, Z. (2021). SNR coverage probability analysis of RIS-aided communication systems. IEEE Transactions on Vehicular Technology, 70(4), 3914–3919. https://doi.org/10.1109/TVT.2021.3063408 [Google Scholar] [CrossRef]
33. Yildirim, I., Uyrus, A., Basar, E. (2021). Modeling and analysis of reconfigurable intelligent surfaces for indoor and outdoor applications in future wireless networks. IEEE Transactions on Communications, 69(2), 1290–1301. https://doi.org/10.1109/TCOMM.2020.3035391 [Google Scholar] [CrossRef]
34. Long, R., Liang, Y. C., Pei, Y., Larsson, E. G. (2021). Active reconfigurable intelligent surface-aided wireless communications. IEEE Transactions on Communications, 20(8), 4962–4975. [Google Scholar]
35. You, C., Zhang, R. (2021). Wireless communication aided by intelligent reflecting surface: Active or passive? IEEE Wireless Communications Letters, 10(12), 2659–2663. https://doi.org/10.1109/LWC.2021.3111044 [Google Scholar] [CrossRef]
36. Zhi, K., Pan, C., Ren, H., Chai, K. K., Elkashlan, M. (2022). Active RIS vs. passive RIS: Which is superior with the same power budget? IEEE Communications Letters, 26(5), 1150–1154. https://doi.org/10.1109/LCOMM.2022.3159525 [Google Scholar] [CrossRef]
37. Zeng, P., Qiao, D., Wu, Q., Wu, Y. (2021). Active IRS aided WPCNs: A new paradigm towards higher efficiency and wider coverage. https://arxiv.org/abs/2111.11600v1 [Google Scholar]
38. Liu, Y., Mu, X., Liu, X., di Renzo, M., Ding, Z. et al. (2022). Reconfigurable intelligent surface-aided multi-user networks: Interplay between NOMA and RIS. IEEE Wireless Communications, 29(2), 169–176. https://doi.org/10.1109/MWC.102.2100363 [Google Scholar] [CrossRef]
39. Ding, Z., Lv, L., Fang, F., Dobre, O. A., Karagiannidis, G. K. et al. (2022). A state-of-the-art survey on reconfigurable intelligent surface-assisted non-orthogonal multiple access networks. Proceedings of the IEEE, 110(9), 1358–1379. https://doi.org/10.1109/JPROC.2022.3174140 [Google Scholar] [CrossRef]
40. Hou, T., Liu, Y., Song, Z., Sun, X., Chen, Y. et al. (2020). Reconfigurable intelligent surface aided NOMA networks. IEEE Journal on Selected Areas in Communications, 38(11), 2575–2588. https://doi.org/10.1109/JSAC.2020.3007039 [Google Scholar] [CrossRef]
41. Ding, Z., Schober, R., Poor, H. V. (2020). On the impact of phase shifting designs on IRS-NOMA. IEEE Wireless Communications Letters, 9(10), 1596–1600. https://doi.org/10.1109/LWC.2020.2991116 [Google Scholar] [CrossRef]
42. Yue, X., Liu, Y. (2022). Performance analysis of intelligent reflecting surface assisted NOMA networks. IEEE Transactions on Wireless Communications, 21(4), 2623–2636. https://doi.org/10.1109/TWC.2021.3114221 [Google Scholar] [CrossRef]
43. Li, X., Xie, Z., Chu, Z., Menon, V. G., Mumtaz, S. et al. (2022). Exploiting benefits of IRS in wireless powered NOMA networks. IEEE Transactions on Green Communications and Networking, 6(1), 175–186. https://doi.org/10.1109/TGCN.2022.3144744 [Google Scholar] [CrossRef]
44. Li, X., Xie, Z., Huang, G., Zhang, J., Zeng, M. et al. (2022). Sum rate maximization for RIS-aided NOMA with direct links. IEEE Networking Letters, 4(2), 55–58. https://doi.org/10.1109/LNET.2022.3161981 [Google Scholar] [CrossRef]
45. Khaleel, A., Basar, E. (2022). A novel NOMA solution with RIS partitioning. IEEE Journal of Selected Topics in Signal Processing, 16(1), 70–81. https://doi.org/10.1109/JSTSP.2021.3127725 [Google Scholar] [CrossRef]
46. Cheng, Y., Li, K. H., Liu, Y., Teh, K. C., Karagiannidis, G. K. (2021). Non-orthogonal multiple access (NOMA) with multiple intelligent reflecting surfaces. IEEE Transactions on Wireless Communications, 20(11), 7184–7195. https://doi.org/10.1109/TWC.2021.3081423 [Google Scholar] [CrossRef]
47. Liu, Z., Yue, X., Zhang, C., Liu, Y., Wang, Y. et al. (2022). Performance analysis of reconfigurable intelligent surface assisted two-way NOMA networks. IEEE Transactions on Vehicular Technology, 71(12), 13091–13104. [Google Scholar]
48. Lv, L., Wu, Q., Li, Z., Ding, Z., Al-Dhahir, N. et al. (2022). Covert communication in intelligent reflecting surface-assisted NOMA systems: Design, analysis, and optimization. IEEE Transactions on Wireless Communications, 21(3), 1735–1750. https://doi.org/10.1109/TWC.2021.3106346 [Google Scholar] [CrossRef]
49. Wang, H., Shi, Z., Fu, Y., Fu, S. (2022). On intelligent reflecting surface-assisted NOMA uplinks with imperfect SIC. IEEE Wireless Communication Letters, 11(7), 1518–1522. https://doi.org/10.1109/LWC.2022.3177733 [Google Scholar] [CrossRef]
50. Chen, G., Wu, Q., He, C., Chen, W., Tang, J. et al. (2022). Active IRS aided multiple access for energy-constrained IoT systems. https://arxiv.org/abs/2201.12565v1 [Google Scholar]
51. Kishor, K. K., Hum, S. V. (2012). An amplifying reconfigurable reflectarray antenna. IEEE Transactions on Antennas and Propagation, 60(1), 197–205. https://doi.org/10.1109/TAP.2011.2167939 [Google Scholar] [CrossRef]
52. Amato, F., Peterson, C. W., Degnan, B. P., Durgin, G. D. (2018). Tunneling RFID tags for long-range and low-power microwave applications. IEEE Journal of Radio Frequency Identification, 2(2), 93–103. https://doi.org/10.1109/JRFID.2018.2852498 [Google Scholar] [CrossRef]
53. Gradshteyn, I. S., Ryzhik, I. M. (2000). Table of integrals, series and products. sixth edition. New York, NY, USA: Academic Press. [Google Scholar]
54. Laneman, J. N., Tse, D. N. C., Wornell, G. W. (2004). Cooperative diversity in wireless networks: Efficient protocols and outage behavior. IEEE Transactions on Information Theory, 50(12), 3062–3080. [Google Scholar]
55. Kwon, T., Lim, S., Choi, S., Hong, D. (2010). Optimal duplex mode for DF relay in terms of the outage probability. IEEE Transactions on Vehicular Technology, 59(7), 3628–3634. [Google Scholar]
56. Liu, H., Ding, H., Xiang, L., Yuana, J., Zhenga, L. (2014). Outage and ber performance analysis of cascade channel in relay networks. Proceedings the 9th International Conference on Future Networks and Communications. [Google Scholar]
57. David, H. A., Nagaraja, H. N. (2003). Order statistics. Third edition. New York: John Wiley. [Google Scholar]
58. Abramowitz, M., Stegun, I. (1972). Handbook of mathematical functions with formulas, graphs, and mathematical tables. New York, NY, USA: Dover. [Google Scholar]
Appendix A: Proof of Theorem 1
The proof process starts by assuming
where
where
where
Upon substituting (A.3) and (A.4) into (A.1), the outage probability of user
where
As a further advance, the Gauss-Laguerre integration [58] can be selected to approximate the above equation. More specifically, this integration is expressed as
With the help of 1-bit coding scheme,
The proof is completed.
Appendix B: Proof of Theorem 2
When
With the assistance of (A.3), the CDF of random variable
It is worth noting that the PDF of random variable
where
Then further applying Gauss-Laguerre integration in the above equation, the outage probability of user
It is known from the 1-bit coding scheme that
The proof is completed.
Cite This Article
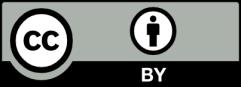
This work is licensed under a Creative Commons Attribution 4.0 International License , which permits unrestricted use, distribution, and reproduction in any medium, provided the original work is properly cited.