Open Access
ARTICLE
Improved Supervised and Unsupervised Metaheuristic-Based Approaches to Detect Intrusion in Various Datasets
1 Faculty of Sciences and Technology, Department of Computer Sciences, Cadi Ayyad University, Marrakech, 40000, Morocco
2 Mathematics and Informatics Department, Royal Air School, Marrakech, 40000, Morocco
* Corresponding Author: Ouail Mjahed. Email:
Computer Modeling in Engineering & Sciences 2023, 137(1), 265-298. https://doi.org/10.32604/cmes.2023.027581
Received 04 November 2022; Accepted 06 January 2023; Issue published 23 April 2023
Abstract
Due to the increasing number of cyber-attacks, the necessity to develop efficient intrusion detection systems (IDS) is more imperative than ever. In IDS research, the most effectively used methodology is based on supervised Neural Networks (NN) and unsupervised clustering, but there are few works dedicated to their hybridization with metaheuristic algorithms. As intrusion detection data usually contains several features, it is essential to select the best ones appropriately. Linear Discriminant Analysis (LDA) and t-statistic are considered as efficient conventional techniques to select the best features, but they have been little exploited in IDS design. Thus, the research proposed in this paper can be summarized as follows. a) The proposed approach aims to use hybridized unsupervised and hybridized supervised detection processes of all the attack categories in the CICIDS2017 Dataset. Nevertheless, owing to the large size of the CICIDS2017 Dataset, only 25% of the data was used. b) As a feature selection method, the LDA performance measure is chosen and combined with the t-statistic. c) For intrusion detection, unsupervised Fuzzy C-means (FCM) clustering and supervised Back-propagation NN are adopted. d) In addition and in order to enhance the suggested classifiers, FCM and NN are hybridized with the seven most known metaheuristic algorithms, including Genetic Algorithm (GA), Particle Swarm Optimization (PSO), Differential Evolution (DE), Cultural Algorithm (CA), Harmony Search (HS), Ant-Lion Optimizer (ALO) and Black Hole (BH) Algorithm. Performance metrics extracted from confusion matrices, such as accuracy, precision, sensitivity and F1-score are exploited. The experimental result for the proposed intrusion detection, based on training and test CICIDS2017 datasets, indicated that PSO, GA and ALO-based NNs can achieve promising results. PSO-NN produces a tested accuracy, global sensitivity and F1-score of 99.97%, 99.95% and 99.96%, respectively, outperforming performance concluded in several related works. Furthermore, the best-proposed approaches are valued in the most recent intrusion detection datasets: CSE-CICIDS2018 and LUFlow2020. The evaluation fallouts consolidate the previous results and confirm their correctness.Keywords
Cite This Article
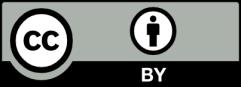
This work is licensed under a Creative Commons Attribution 4.0 International License , which permits unrestricted use, distribution, and reproduction in any medium, provided the original work is properly cited.