Open Access
ARTICLE
Dual-Branch-UNet: A Dual-Branch Convolutional Neural Network for Medical Image Segmentation
1 School of Information Science and Technology, Linyi University, Linyi, 276000, China
2 School of Computer Science and Technology, Shandong University of Finance and Economics, Jinan, 250014, China
3 School of Science & Engineering, University of Limerick, Limerick, V94 T9PX, Ireland
* Corresponding Author: Muwei Jian. Email:
# They contributed equally to this work and shared the first authorship
(This article belongs to the Special Issue: Advanced Intelligent Decision and Intelligent Control with Applications in Smart City)
Computer Modeling in Engineering & Sciences 2023, 137(1), 705-716. https://doi.org/10.32604/cmes.2023.027425
Received 28 October 2022; Accepted 30 December 2022; Issue published 23 April 2023
Abstract
In intelligent perception and diagnosis of medical equipment, the visual and morphological changes in retinal vessels are closely related to the severity of cardiovascular diseases (e.g., diabetes and hypertension). Intelligent auxiliary diagnosis of these diseases depends on the accuracy of the retinal vascular segmentation results. To address this challenge, we design a Dual-Branch-UNet framework, which comprises a Dual-Branch encoder structure for feature extraction based on the traditional U-Net model for medical image segmentation. To be more explicit, we utilize a novel parallel encoder made up of various convolutional modules to enhance the encoder portion of the original U-Net. Then, image features are combined at each layer to produce richer semantic data and the model’s capacity is adjusted to various input images. Meanwhile, in the lower sampling section, we give up pooling and conduct the lower sampling by convolution operation to control step size for information fusion. We also employ an attention module in the decoder stage to filter the image noises so as to lessen the response of irrelevant features. Experiments are verified and compared on the DRIVE and ARIA datasets for retinal vessels segmentation. The proposed Dual-Branch-UNet has proved to be superior to other five typical state-of-the-art methods.Keywords
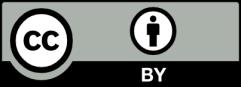