Open Access
ARTICLE
Rectal Cancer Stages T2 and T3 Identification Based on Asymptotic Hybrid Feature Maps
1 R&D Center of Artificial Intelligence Systems and Applications, School of Artificial Intelligence and Computer Science, Jiangnan University, Wuxi, 214122, China
2 Shanghai Enterprise Information Operation Center, China Telecom Group Co., Ltd., Shanghai, 201315, China
3 Department of Radiology, Dushu Lake Hospital Affiliated to Soochow University, Suzhou, 215123, China
4 Suzhou Yunmai Software Technology Co., Ltd., Suzhou, 215100, China
* Corresponding Author: Pengjiang Qian. Email:
(This article belongs to the Special Issue: Intelligent Biomedical Image Processing and Computer Vision)
Computer Modeling in Engineering & Sciences 2023, 137(1), 923-938. https://doi.org/10.32604/cmes.2023.027356
Received 26 October 2022; Accepted 19 December 2022; Issue published 23 April 2023
Abstract
Many existing intelligent recognition technologies require huge datasets for model learning. However, it is not easy to collect rectal cancer images, so the performance is usually low with limited training samples. In addition, traditional rectal cancer staging is time-consuming, error-prone, and susceptible to physicians’ subjective awareness as well as professional expertise. To settle these deficiencies, we propose a novel deep-learning model to classify the rectal cancer stages of T2 and T3. First, a novel deep learning model (RectalNet) is constructed based on residual learning, which combines the squeeze-excitation with the asymptotic output layer and new cross-convolution layer links in the residual block group. Furthermore, a two-stage data augmentation is designed to increase the number of images and reduce deep learning’s dependence on the volume of data. The experiment results demonstrate that the proposed method is superior to many existing ones, with an overall accuracy of 0.8583. Oppositely, other traditional techniques, such as VGG16, DenseNet121, EL, and DERNet, have an average accuracy of 0.6981, 0.7032, 0.7500, and 0.7685, respectively.Keywords
Cite This Article
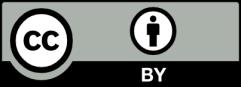