Open Access
ARTICLE
A Novel Detection Method for Pavement Crack with Encoder-Decoder Architecture
1 Anhui Province Key Laboratory of Intelligent Building and Building Energy Saving, Anhui Jianzhu University, Hefei, 230022, China
2 Anhui Institute of Strategic Study on Carbon Dioxide Emissions Peak and Carbon Neutrality in Urban-Rural Development, Hefei, 230022, China
3 School of Electronic and Information Engineering, Anhui Jianzhu University, Hefei, 230601, China
4 School of Mechanical and Electrical Engineering, Anhui Jianzhu University, Hefei, 230601, China
* Corresponding Author: Liangliang Su. Email:
(This article belongs to the Special Issue: Advanced Intelligent Decision and Intelligent Control with Applications in Smart City)
Computer Modeling in Engineering & Sciences 2023, 137(1), 761-773. https://doi.org/10.32604/cmes.2023.027010
Received 09 October 2022; Accepted 16 January 2023; Issue published 23 April 2023
Abstract
As a current popular method, intelligent detection of cracks is of great significance to road safety, so deep learning has gradually attracted attention in the field of crack image detection. The nonlinear structure, low contrast and discontinuity of cracks bring great challenges to existing crack detection methods based on deep learning. Therefore, an end-to-end deep convolutional neural network (AttentionCrack) is proposed for automatic crack detection to overcome the inaccuracy of boundary location between crack and non-crack pixels. The AttentionCrack network is built on U-Net based encoder-decoder architecture, and an attention mechanism is incorporated into the multi-scale convolutional feature to enhance the recognition of crack region. Additionally, a dilated convolution module is introduced in the encoder-decoder architecture to reduce the loss of crack detail due to the pooling operation in the encoder network. Furthermore, since up-sampling will lead to the loss of crack boundary information in the decoder network, a depthwise separable residual module is proposed to capture the boundary information of pavement crack. The AttentionCrack net on public pavement crack image datasets named CrackSegNet and Crack500 is trained and tested, the results demonstrate that the AttentionCrack achieves F1 score over 0.70 on the CrackSegNet and 0.71 on the Crack500 in average and outperforms the current state-of-the-art methods.Keywords
Cite This Article
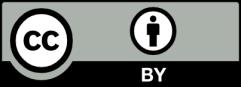