Open Access
ARTICLE
Ensemble Model for Spindle Thermal Displacement Prediction of Machine Tools
1 Department of Mechanical Engineering, National Chung Cheng University, Chiayi, 62102, Taiwan
2 Advanced Institute of Manufacturing with High-Tech Innovations (AIM-HI), National Chung Cheng University, Chiayi, 62102, Taiwan
* Corresponding Author: Her-Terng Yau. Email:
(This article belongs to the Special Issue: Computational Mechanics Assisted Modern Urban Planning and Infrastructure)
Computer Modeling in Engineering & Sciences 2023, 137(1), 319-343. https://doi.org/10.32604/cmes.2023.026860
Received 29 September 2022; Accepted 04 January 2023; Issue published 23 April 2023
Abstract
Numerous factors affect the increased temperature of a machine tool, including prolonged and high-intensity usage, tool-workpiece interaction, mechanical friction, and elevated ambient temperatures, among others. Consequently, spindle thermal displacement occurs, and machining precision suffers. To prevent the errors caused by the temperature rise of the Spindle from affecting the accuracy during the machining process, typically, the factory will warm up the machine before the manufacturing process. However, if there is no way to understand the tool spindle's thermal deformation, the machining quality will be greatly affected. In order to solve the above problem, this study aims to predict the thermal displacement of the machine tool by using intelligent algorithms. In the practical application, only a few temperature sensors are used to input the information into the prediction model for real-time thermal displacement prediction. This approach has greatly improved the quality of tool processing. However, each algorithm has different performances in different environments. In this study, an ensemble model is used to integrate Long Short-Term Memory (LSTM) with Support Vector Machine (SVM). The experimental results show that the prediction performance of LSTM-SVM is higher than that of other machine learning algorithms.Keywords
Cite This Article
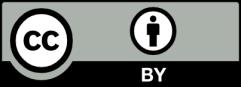
This work is licensed under a Creative Commons Attribution 4.0 International License , which permits unrestricted use, distribution, and reproduction in any medium, provided the original work is properly cited.