Open Access
ARTICLE
Filter Bank Networks for Few-Shot Class-Incremental Learning
School of Electronic, Electric and Communication Engineering, University of Chinese Academy of Sciences, Beijing, 140018, China
* Corresponding Author: Jianbin Jiao. Email:
(This article belongs to the Special Issue: Deep Learning for Marine and Underwater Environment: Theory, Method, and Applications)
Computer Modeling in Engineering & Sciences 2023, 137(1), 647-668. https://doi.org/10.32604/cmes.2023.026745
Received 28 September 2022; Accepted 14 December 2022; Issue published 23 April 2023
Abstract
Deep Convolution Neural Networks (DCNNs) can capture discriminative features from large datasets. However, how to incrementally learn new samples without forgetting old ones and recognize novel classes that arise in the dynamically changing world, e.g., classifying newly discovered fish species, remains an open problem. We address an even more challenging and realistic setting of this problem where new class samples are insufficient, i.e., Few-Shot Class-Incremental Learning (FSCIL). Current FSCIL methods augment the training data to alleviate the overfitting of novel classes. By contrast, we propose Filter Bank Networks (FBNs) that augment the learnable filters to capture fine-detailed features for adapting to future new classes. In the forward pass, FBNs augment each convolutional filter to a virtual filter bank containing the canonical one, i.e., itself, and multiple transformed versions. During back-propagation, FBNs explicitly stimulate fine-detailed features to emerge and collectively align all gradients of each filter bank to learn the canonical one. FBNs capture pattern variants that do not yet exist in the pretraining session, thus making it easy to incorporate new classes in the incremental learning phase. Moreover, FBNs introduce model-level prior knowledge to efficiently utilize the limited few-shot data. Extensive experiments on MNIST, CIFAR100, CUB200, and Mini-ImageNet datasets show that FBNs consistently outperform the baseline by a significant margin, reporting new state-of-the-art FSCIL results. In addition, we contribute a challenging FSCIL benchmark, Fishshot1K, which contains 8261 underwater images covering 1000 ocean fish species. The code is included in the supplementary materials.Keywords
Cite This Article
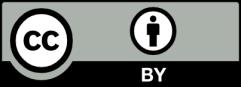