Open Access
ARTICLE
A Shifting Strategy for Electric Commercial Vehicles Considering Mass and Gradient Estimation
1 School of Mechanical and Electrical Engineering, Guilin University of Electronic Technology, Guilin, 541004, China
2 Commercial Vehicle Technology Center, Dongfeng Liuzhou Automobile Co., Ltd., Liuzhou, 545005, China
3 School of Mechanical and Automotive Engineering, Guangxi University of Science and Technology, Liuzhou, 545005, China
* Corresponding Author: Shanchao Wang. Email:
(This article belongs to the Special Issue: AI and Machine Learning Modeling in Civil and Building Engineering)
Computer Modeling in Engineering & Sciences 2023, 137(1), 489-508. https://doi.org/10.32604/cmes.2023.025169
Received 26 June 2022; Accepted 15 December 2022; Issue published 23 April 2023
Abstract
The extended Kalman filter (EKF) algorithm and acceleration sensor measurements were used to identify vehicle mass and road gradient in the work. Four different states of fixed mass, variable mass, fixed slope and variable slope were set to simulate real-time working conditions, respectively. A comprehensive electric commercial vehicle shifting strategy was formulated according to the identification results. The co-simulation results showed that, compared with the recursive least square (RLS) algorithm, the proposed algorithm could identify the real-time vehicle mass and road gradient quickly and accurately. The comprehensive shifting strategy formulated had the following advantages, e.g., avoiding frequent shifting of vehicles up the hill, making full use of motor braking down the hill, and improving the overall performance of vehicles.Graphical Abstract
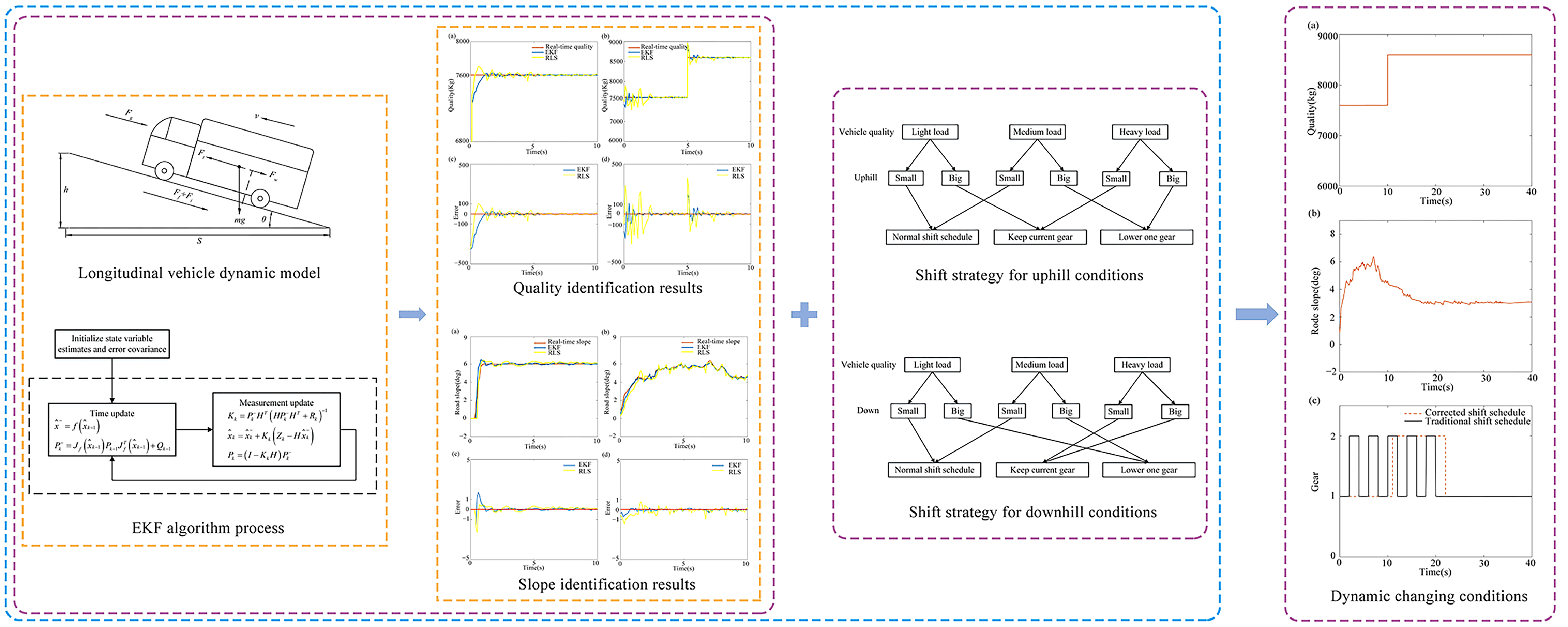
Keywords
Cite This Article
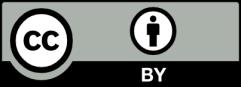