Open Access
ARTICLE
3D Human Pose Estimation Using Two-Stream Architecture with Joint Training
1 National and Local Joint Engineering Laboratory of Computer Aided Design, School of Software Engineering, Dalian University, Dalian, 116622, China
2 Dalian Maritime University, Dalian, 116023, China
* Corresponding Author: Dongsheng Zhou. Email:
(This article belongs to the Special Issue: Recent Advances in Virtual Reality)
Computer Modeling in Engineering & Sciences 2023, 137(1), 607-629. https://doi.org/10.32604/cmes.2023.024420
Received 30 May 2022; Accepted 22 December 2022; Issue published 23 April 2023
Abstract
With the advancement of image sensing technology, estimating 3D human pose from monocular video has become a hot research topic in computer vision. 3D human pose estimation is an essential prerequisite for subsequent action analysis and understanding. It empowers a wide spectrum of potential applications in various areas, such as intelligent transportation, human-computer interaction, and medical rehabilitation. Currently, some methods for 3D human pose estimation in monocular video employ temporal convolutional network (TCN) to extract inter-frame feature relationships, but the majority of them suffer from insufficient inter-frame feature relationship extractions. In this paper, we decompose the 3D joint location regression into the bone direction and length, we propose the TCG, a temporal convolutional network incorporating Gaussian error linear units (GELU), to solve bone direction. It enables more inter-frame features to be captured and makes the utmost of the feature relationships between data. Furthermore, we adopt kinematic structural information to solve bone length enhancing the use of intra-frame joint features. Finally, we design a loss function for joint training of the bone direction estimation network with the bone length estimation network. The proposed method has extensively experimented on the public benchmark dataset Human3.6M. Both quantitative and qualitative experimental results showed that the proposed method can achieve more accurate 3D human pose estimations.Keywords
Cite This Article
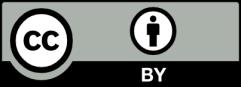
This work is licensed under a Creative Commons Attribution 4.0 International License , which permits unrestricted use, distribution, and reproduction in any medium, provided the original work is properly cited.