Open Access
ARTICLE
SepFE: Separable Fusion Enhanced Network for Retinal Vessel Segmentation
1 College of Computer Science and Technology, Hengyang Normal University, Hengyang, 421002, China
2 Hunan Provincial Key Laboratory of Intelligent Information Processing and Application, Hengyang, 421002, China
* Corresponding Author: Ge Jiao. Email:
(This article belongs to the Special Issue: Artificial Intelligence for Mobile Edge Computing in IoT)
Computer Modeling in Engineering & Sciences 2023, 136(3), 2465-2485. https://doi.org/10.32604/cmes.2023.026189
Received 22 August 2022; Accepted 25 November 2022; Issue published 09 March 2023
Abstract
The accurate and automatic segmentation of retinal vessels from fundus images is critical for the early diagnosis and prevention of many eye diseases, such as diabetic retinopathy (DR). Existing retinal vessel segmentation approaches based on convolutional neural networks (CNNs) have achieved remarkable effectiveness. Here, we extend a retinal vessel segmentation model with low complexity and high performance based on U-Net, which is one of the most popular architectures. In view of the excellent work of depth-wise separable convolution, we introduce it to replace the standard convolutional layer. The complexity of the proposed model is reduced by decreasing the number of parameters and calculations required for the model. To ensure performance while lowering redundant parameters, we integrate the pre-trained MobileNet V2 into the encoder. Then, a feature fusion residual module (FFRM) is designed to facilitate complementary strengths by enhancing the effective fusion between adjacent levels, which alleviates extraneous clutter introduced by direct fusion. Finally, we provide detailed comparisons between the proposed SepFE and U-Net in three retinal image mainstream datasets (DRIVE, STARE, and CHASEDB1). The results show that the number of SepFE parameters is only 3% of U-Net, the Flops are only 8% of U-Net, and better segmentation performance is obtained. The superiority of SepFE is further demonstrated through comparisons with other advanced methods.Keywords
Cite This Article
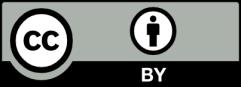
This work is licensed under a Creative Commons Attribution 4.0 International License , which permits unrestricted use, distribution, and reproduction in any medium, provided the original work is properly cited.