Open Access
ARTICLE
COVID-19 Detection Based on 6-Layered Explainable Customized Convolutional Neural Network
1 School of Physics and Information Engineering, Jiangsu Second Normal University, Nanjing, 211200, China
2 State Key Laboratory of Millimeter Waves, Southeast University, Nanjing, 210096, China
3 Jiangsu Province Engineering Research Center of Basic Education Big Data Application, Nanjing, 211200, China
4 Department of Electrical Engineering, Federal University of Santa Catarina, Florianópolis, 88040-900, Brazil
* Corresponding Authors: Shuwen Chen. Email: ; Dimas Lima. Email:
# These authors contributed equally to this work. Jiaji Wang, Shuwen Chen and Yu Cao are regarded as co-first authors
(This article belongs to the Special Issue: Computer Modeling of Artificial Intelligence and Medical Imaging)
Computer Modeling in Engineering & Sciences 2023, 136(3), 2595-2616. https://doi.org/10.32604/cmes.2023.025804
Received 31 July 2022; Accepted 20 October 2022; Issue published 09 March 2023
Abstract
This paper presents a 6-layer customized convolutional neural network model (6L-CNN) to rapidly screen out patients with COVID-19 infection in chest CT images. This model can effectively detect whether the target CT image contains images of pneumonia lesions. In this method, 6L-CNN was trained as a binary classifier using the dataset containing CT images of the lung with and without pneumonia as a sample. The results show that the model improves the accuracy of screening out COVID-19 patients. Compared to other methods, the performance is better. In addition, the method can be extended to other similar clinical conditions.Keywords
Cite This Article
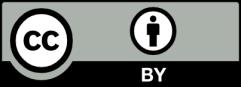
This work is licensed under a Creative Commons Attribution 4.0 International License , which permits unrestricted use, distribution, and reproduction in any medium, provided the original work is properly cited.