Open Access
REVIEW
Application of U-Net and Optimized Clustering in Medical Image Segmentation: A Review
1 School of Physics and Information Engineering, Jiangsu Second Normal University, Nanjing, 211200, China
2 State Key Laboratory of Millimeter Waves, Southeast University, Nanjing, 210096, China
3 Jiangsu Province Engineering Research Center of Basic Education Big Data Application, Nanjing, 211200, China
4 School of Data Science, Perdana University, Serdang, Selangor, 43400, Malaysia
* Corresponding Authors: Shuwen Chen. Email: ; Mackenzie Brown. Email:
# These authors contributed equally to this work. Jiaqi Shao, Shuwen Chen and Jin Zhou are regarded as co-first authors
(This article belongs to the Special Issue: Computer Modeling of Artificial Intelligence and Medical Imaging)
Computer Modeling in Engineering & Sciences 2023, 136(3), 2173-2219. https://doi.org/10.32604/cmes.2023.025499
Received 17 July 2022; Accepted 08 November 2022; Issue published 09 March 2023
Abstract
As a mainstream research direction in the field of image segmentation, medical image segmentation plays a key role in the quantification of lesions, three-dimensional reconstruction, region of interest extraction and so on. Compared with natural images, medical images have a variety of modes. Besides, the emphasis of information which is conveyed by images of different modes is quite different. Because it is time-consuming and inefficient to manually segment medical images only by professional and experienced doctors. Therefore, large quantities of automated medical image segmentation methods have been developed. However, until now, researchers have not developed a universal method for all types of medical image segmentation. This paper reviews the literature on segmentation techniques that have produced major breakthroughs in recent years. Among the large quantities of medical image segmentation methods, this paper mainly discusses two categories of medical image segmentation methods. One is the improved strategies based on traditional clustering method. The other is the research progress of the improved image segmentation network structure model based on U-Net. The power of technology proves that the performance of the deep learning-based method is significantly better than that of the traditional method. This paper discussed both advantages and disadvantages of different algorithms and detailed how these methods can be used for the segmentation of lesions or other organs and tissues, as well as possible technical trends for future work.Keywords
Cite This Article
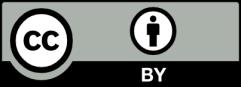
This work is licensed under a Creative Commons Attribution 4.0 International License , which permits unrestricted use, distribution, and reproduction in any medium, provided the original work is properly cited.