Open Access
ARTICLE
Research on Short-Term Load Forecasting of Distribution Stations Based on the Clustering Improvement Fuzzy Time Series Algorithm
1 College of Information Engineering, Zhejiang University of Technology, Hangzhou, 310023, China
2 Electric Power Research Institute of State Grid Anhui Electric Power Co., Ltd., Hefei, 230601, China
3 State Grid Zhejiang Electric Power Co., Ltd., Wencheng County Power Supply Company, Wenzhou, 325000, China
4 Zhejiang Tusheng Power Transmission and Transformation Engineering Co., Ltd., Tusheng Technology Branch, Wenzhou, 325000, China
* Corresponding Author: Youbing Zhang. Email:
Computer Modeling in Engineering & Sciences 2023, 136(3), 2221-2236. https://doi.org/10.32604/cmes.2023.025396
Received 09 July 2022; Accepted 03 November 2022; Issue published 09 March 2023
Abstract
An improved fuzzy time series algorithm based on clustering is designed in this paper. The algorithm is successfully applied to short-term load forecasting in the distribution stations. Firstly, the K-means clustering method is used to cluster the data, and the midpoint of two adjacent clustering centers is taken as the dividing point of domain division. On this basis, the data is fuzzed to form a fuzzy time series. Secondly, a high-order fuzzy relation with multiple antecedents is established according to the main measurement indexes of power load, which is used to predict the short-term trend change of load in the distribution stations. Matlab/Simulink simulation results show that the load forecasting errors of the typical fuzzy time series on the time scale of one day and one week are [−50, 20] and [−50, 30], while the load forecasting errors of the improved fuzzy time series on the time scale of one day and one week are [−20, 15] and [−20, 25]. It shows that the fuzzy time series algorithm improved by clustering improves the prediction accuracy and can effectively predict the short-term load trend of distribution stations.Keywords
Cite This Article
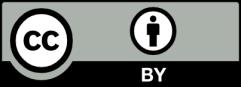
This work is licensed under a Creative Commons Attribution 4.0 International License , which permits unrestricted use, distribution, and reproduction in any medium, provided the original work is properly cited.