Open Access
ARTICLE
On a Novel Extended Lomax Distribution with Asymmetric Properties and Its Statistical Applications
1
Department of Statistics, Faculty of Science, King Abdulaziz University, Jeddah, 21589, Saudi Arabia
2
Université de Caen Normandie, LMNO, Campus II, Science 3, Caen, 14032, France
3
Department of Statistics, the Islamia University of Bahawalpur, Punjab, 63100, Pakistan
* Corresponding Author: Christophe Chesneau. Email:
Computer Modeling in Engineering & Sciences 2023, 136(3), 2371-2403. https://doi.org/10.32604/cmes.2023.027000
Received 08 October 2022; Accepted 02 December 2022; Issue published 09 March 2023
Abstract
In this article, we highlight a new three-parameter heavy-tailed lifetime distribution that aims to extend the modeling possibilities of the Lomax distribution. It is called the extended Lomax distribution. The considered distribution naturally appears as the distribution of a transformation of a random variable following the logweighted power distribution recently introduced for percentage or proportion data analysis purposes. As a result, its cumulative distribution has the same functional basis as that of the Lomax distribution, but with a novel special logarithmic term depending on several parameters. The modulation of this logarithmic term reveals new types of asymetrical shapes, implying a modeling horizon beyond that of the Lomax distribution. In the first part, we examine several of its mathematical properties, such as the shapes of the related probability and hazard rate functions; stochastic comparisons; manageable expansions for various moments; and quantile properties. In particular, based on the quantile functions, various actuarial measures are discussed. In the second part, the distribution’s applicability is investigated with the use of the maximum likelihood estimation method. The behavior of the obtained parameter estimates is validated by a simulation work. Insurance claim data are analyzed. We show that the proposed distribution outperforms eight well-known distributions, including the Lomax distribution and several extended Lomax distributions. In addition, we demonstrate that it gives preferable inferences from these competitor distributions in terms of risk measures.Keywords
A brief state of the art of the Lomax (Lo) distribution, as well as some of its recent extensions, is necessary to appreciate the interest of our study. To begin, the Lo distribution has two parameters and can be viewed as a variant of the generalized Pareto distribution, commonly known as the Pareto of the second type (or type II). Mathematically speaking, it is defined by the following cumulative distribution function (cdf):
where
and the hazard rate function (hrf) is specified by
The Lo distribution has been used in a variety of ways in the literature. The authors in [1], for example, have widely used it for reliability modeling and life testing. When the data are heavily tailed, it has also been employed as an alternative to the exponential distribution (see [2]). The authors in [3] investigated the Lo distribution’s record values. Some recurrence links between the moments of record values from the Lo distribution were suggested in [4]. The authors in [5] investigated the order statistics of non-identical right-truncated Lo random variables. In addition, numerous scholars have explored the Lo model from a Bayesian perspective, see, for example, [6]. The authors in [7] proposed a Bayesian estimation of the Lo distribution’s survival function. The authors in [8] examined Lo distribution data that had been progressively type-II censored for competing risks. The authors in [9] have looked at the stress-strength model estimation problem for a Lo distribution based on general progressive-censored data. The authors in [10] discussed the Lo distribution’s uses in economics, actuarial modeling, queuing difficulties, and biological sciences. The Lo distribution has been extended in various ways, with various transformations adding one or more parameters. Among these extensions, we may mention the Marshall-Olkin extended Lo distribution in [11], later study on the statistical side in [12], the exponentiated Lo distribution in [13], beta Lo distribution in [14], Poisson Lo distribution in [15], exponential Lo distribution in [16], gamma Lo distribution in [17], Weibull Lo distribution in [18], beta exponentiated Lo distribution in [19], power Lo distribution in [20], exponentiated Weibull Lo distribution in [21], Weibull generalized Lo distribution in [22], Marshall-Olkin exponential Lo distribution in [23], type II Topp-Leone power Lo distribution in [24], Marshall-Olkin length biased Lo distribution in [25], Kumaraswamy generalized power Lo distribution in [26], odd Burr Lo distribution in [27], sine power Lo distribution in [28], Nadarajah-Haghighi Lo distribution in [29], new modified inverse Lo distribution in [30], Maxwell-Lo distribution in [31], minimum Lindley Lomax distribution in [32], new Weibull inverse Lo distribution in [33], and quasi-Poisson exponentiated exponential Lo distribution in [34].
In this article, we investigate a new extension of the Lo distribution, called the extended Lomax (ELo) distribution. It is defined by the following cdf:
where
where
The rest of the paper is as follows: Section 2 shows the functional details of the ELo distribution. Technical properties are provided in Section 3, including stochastic comparisons, moment properties, and quantile properties. Section 4 concerns an efficient parametric estimation strategy in the case where the parameters of the ELo distribution are unknown. Section 5 is devoted to the applications of the ELo distribution to insurance claims data with both goodness-of-fits and actuarial measures. A conclusion is provided in Section 6.
2.1 Some Distributional Remarks
The ELo distribution is defined by the cdf given by (4). We recall that, as introduced in [36], the LP distribution is defined by the following cdf:
with
The following result shows the link existing between the ELo and LP distribution.
Proposition 2.1. Let X be a random variable following the LP distribution. The random variable
Proof. We proceed by using the cdfs of the involved random variables. Let us denote by
We recognize the cdf of the ELo distribution, ending the proof of the proposition.
First, let us investigate the asymptotic behavior of the cdf of the ELo distribution presented in Eq. (4). We have
and, for
Since the asymptotic property
Let us examine the analytical behavior of this function, beginning with the asymptotic behavior. We have
Thus, the parameter
As a result, the right tail of the ELo distribution decreases with a “logarihtmic-polynomial” decay, which is slightly slower than the right tail of the Lo distribution.
The following proposition studies the mode properties of the ELo distribution.
Proposition 2.2.
• For
• For
In this case, the ELo distribution is unimodal.
Proof. For
Therefore, for
It is clear that for any
Fig. 1 illustrates the mathematical result aboves by displaying several plots of
Figure 1: Plots of the pdf of the ELo distribution for the following sets of values: (a)
As expected,
Figure 2: Recall of some plots of the pdf of the Lo distribution for the following sets of values: (a)
Now, consider the ratio function
Therefore, for
The hrf of the ELo distribution is defined by
The following limit holds:
The remarks made on
Thus, for all the configurations of the parameters, the hrf decays to 0 with a polynomial rate.
The shape behavior of the hrf is studied in the next proposition.
Proposition 2.3.
• For
• For
In this last case, the shape of
Proof. We have
where
Then the polynomial
and we have
which can be expressed as (19). This point is a maximum since
Fig. 3 provides various plots of
Figure 3: Plots of the hrf of the ELo distribution for the following sets of values: (a)
The UBFR property is particularly illustrated in Fig. 3b. For modeling purposes in the analysis of financial, survival, and environmental data, this is a desired property of the heavy-tailed distribution. We recall that the UBR property is not a quality of the Lo distribution, as shown in Fig. 4.
Figure 4: Recall of some plots of the hrf of the Lo distribution for the following sets of values: (a)
Some technical properties of the ELo distribution are now examined.
Following the approach presented in [38], we now study certain stochastic ordering aspects of the ELo distribution.
Proposition 3.1. The following first-order stochastic (FOS) comparisons hold:
• For
• For
• For
Proof. We proceed by studying the monotonicity of
• For
Since
• For
Since
• For
It is clear that
This ends the proof.
Thanks to Proposition 3.1, we now see how random values from the ELo distribution can be relatively located in comparison to other random values of the ELo distribution with different parameters. This could be useful in cases where the practitioner is unsure which ELo distribution to employ while dealing with data. For further details on stochastic dominance, we may refer to [39–40].
Because they can be used to explain statistical distribution properties, moment properties are important when specifying our probability distribution to work with. As a result, they help to describe the distribution. In this part, different types of moments of the ELo distribution are examined, along with their interpretation.
Hereafter, we designate by X a random variable with the ELo distribution. The following result suggests a clear and simple finite sum expression for the
Proposition 3.2. For any integer
Proof. Since the ELo distribution has the support
By performing the change of variable
For the remaining integral term, upon the change of variable
Therefore, by combining the above equalities, we get
The stated result is obtained, ending the proof.
It is worth noting from Proposition 3.2 that the ELo distribution does not admit moments of all orders.
In particular, according to Proposition 3.2, for
and
Similarly, Proposition 3.2 allows the variance, moments skewness and kurtosis of X to be expressed in terms of
Among the possible generalizations of the moments are the unconditional moments, which naturally appear in various survival measures and are more suitable for use in a practical setting with censored data. On this topic, we may refer to [41,42].
The next result expresses the
Proposition 3.3. For any integer r and fixed
where
Proof. We have
For
By performing the change of variable
For the remaining integral term, by using the incomplete gamma function and doing the change of variable
Therefore, by combining the above equalities, we get
The stated result is obtained, ending the proof of Proposition 3.3.
Based on the conditional moments, we can define the moments of the residual life of the ELo distribution, and the mean residual life (MRL) function in particular. We can refer to [43–45] to support the importance of this last function in various branches of probability and statistics.
Because the ELo distribution does not admit moments of all orders, its quantile properties are fascinating to investigate.
The next result is a closed-form expression for the quantile function (qf) of the ELo distribution.
Proposition 3.4. The qf of the ELo distribution is expressed as
where
Proof. The qf is readily defined as
The desired expression is established, ending the proof of Proposition 3.4.
There are several interests in having a closed-form expression of the qf. First of all, the main quartiles of the ELo distribution can be exhibited. In particular, the median is obtained as
The qf and random values from the uniform distribution over [0, 1] can be used to generate random values from a random variable X following the ELo distribution.
Since the ELo distribution does not admit moments of all orders, quantile measures of skewness and kurtosis, as proposed in [46,47] respectively can be useful. They are respectively defined as
and
In our heavy-tailed distribution setting, we propose to focus on actuarial measures based on the qf. The first measure is the value at risk (VaR). In the setting of the ELo distribution, it is simply defined by
The second mesure is the expected shortfall (ES) introduced by [48], and generally considered as a better measure than VaR. It is defined by
Fig. 5 presents the shape of these actual measures for various values of the parameters with respect to q.
Figure 5: Plots of (a)
According to Fig. 5, both
In addition to the above quantile material, advanced quantile modeling can be done using the qf. See [49] for further details.
We now want to estimate the unknown parameters
Let
Clearly, the ELo distribution does not belong to the exponential family form; the Pitman-Koopman theorem applies. The ML estimates (MLEs) of
Alternatively, they may be defined as
where
They can be obtained by solving the following non-linear equations with respect to
where
and
Unfortunately, because the above equations have no explicit solutions, any numerical approximation technique can be utilized to obtain them. The related standard errors (SEs) can be determined through the calculation of the estimated Fisher information matrix. To accomplish the above calculations, the
The ML method has the advantage of ensuring interesting features for MLEs, such as asymptotic unbiasedness and normality. In particular, the asymptotic unbiasedness provides theoretical guarantees on the fact that, for n large enough, the MLEs must be close to the true unknown parameter values. However, there is no solid guarantee for small n. The asymptotic normality allows us to construct confidence intervals and statistical tests on the unknown parameters based on the normal or normal-transformaiton distribution [50]. Contains more information about these features. We can estimate all of the underlying functions of the ELo distribution using MLEs. In particular, an estimate of the cdf and pdf are given by
The above methodology is for complete samples. Other types of samples, such as censored data samples, can be investigated with appropriate modifications to the definition of the likelihood function. On this topic, we may refer the reader to [7,8,11,51].
The rest of this section is devoted to simulated tests proving the nice behavior of the MLEs.
We carry out a Monte Carlo simulation study in order to underline the accuracy of the MLEs parameters of the ELo distribution. To this end,
respectively, where
Figure 6: Plots of the simulation results on the estimates of the parameters of the ELo distribution for Set 1: (a) Average MLEs, (b) MSEs, (c) Absolute biases and (d) Biases
Figure 7: Plots of the simulation results on the estimates of the parameters of the ELo distribution for Set 2: (a) Average MLEs, (b) MSEs, (c) Absolute biases and (d) Biases
Figure 8: Plots of the simulation results on the estimates of the parameters of the ELo distribution for Set 3: (a) Average MLEs, (b) MSEs, (c) Absolute biases and (d) Biases
These tables and figures reveal that MLEs perform well for estimating the parameters of the ELo distribution. Indeed, as sample size increases, both the bias and MSE are reduced. Therefore, the MLEs and their asymptotic properties can be used quite efficiently. It is worth noting that the MLEs for the parameters in Sets 2 and 3 have better behavior than those in Set 1, especially regarding the estimation of
We complete the above analysis with a precise study of the mean absolute error (MAE) defined by
where
The results are presented in Table 4.
Table 4 is supported graphically by Fig. 9.
Figure 9: Plots of the simulation results on the MAEs for Sets 1, 2, and 3: (a) Set 1, (b) Set 2, and (c) Set 3
As expected, the results show a nice performance of the considered estimates based on the MAE measure.
In the rest of the study, they are used for data fitting purposes and actuarial measure estimation.
In this section, various applications of the ELo distribution are given based on two insurance claim data sets.
This part is devoted to the efficiency of the ELo distribution in the fit of data sets with a heavy tail. To this end, we consider two Kenya car insurance claim data sets from the following link (https://data.world/datasets/insurance/). The first Data Set contains the number of claims from 2012 to 2015, named Data Set 1, while the second Data Set contains the third party theft and fire number of claims from 2012 to 2015, named Data Set 2. The boxplots of these data sets are presented in Fig. 10.
Figure 10: Box plot of (a) Data Set 1 and (b) Data Set 2
From Fig. 10, we observe that the distribution of the data for the two data sets is far too extreme to be in adequation with a normal distribution, with several extreme values. Heavy-tailed distributions are ideal for capturing them in particular and revealing the information behind them.
In addition, with these data, we aim to compare the ELo distribution, with some well-established heavy-tailed distributions, namely the complimentary Dagum Poisson (CDP), Poisson Lomax (PLo), exponentiated Lomax (ExLo), Burr-XII (BX11), Fréchet (Fr), Dagum (Da), inverse Weibull (IW) and Lo distributions. The reader is referred to [52] for a detailed discussion of statistical size distributions used in economics and actuarial sciences. It is worth noting that more extended Lo distributions have been tested for the considered data, but due to unsatisfactory results, they are not presented in the study.
As sketched in the previous section, the
MLEs and their respective SEs for the ELo, CDP, PLo, ExLo, BX11, Fr, Da, IW and Lo distributions which are calculated for the two data sets. They are displayed in Table 5. It is worth mentioning that the scale parameters of the CDP and Da distributions are considered as units.
The values of the GoFs are reported in Tables 6 and 7 for Data Sets 1 and 2, respectively.
According to Tables 6 and 7, the proposed ELo distribution fits both data sets better than all other competitor distributions because it has the lowest AIC, BIC, HQIC,
Figure 11: Plots of the estimated pdfs of the ELo distribution for (a) Data Set 1 and (b) Data Set 2
Figure 12: Plots of the estimated cdfs of the ELo distribution for (a) Data Set 1 and (b) Data Set 2
The estimated functions of the ELo distribution have good fits, which supports the numerical results of the study.
This is also confirmed by a quantile estimation analysis; we plot the quantile-quantile (Q-Q) plots of both data sets in Fig. 13.
Figure 13: Q-Q-plots for the ELo distribution for (a) Data Set 1 and (b) Data Set 2
It is clear that the scatter plots are well adjusted by the respective Q-Q lines, proving the fit hability of the ELo distribution.
5.2 Numerical Illustration of VaR and ES
Here, since Data Sets 1 and 2 are well fitted by the ELo distribution, we provide estimates of the unknown associated risk measures VaR and ES. More precisely, a comparative study of VaR and ES for the ELo distribution with other heavy-tailed distributions: the ExLo, Lo and Da distributions, is performed by taking the MLEs of the parameters. It is worth emphasizing that a distribution with higher values of the risk measures is said to have a heavier tail.
Tables 8 and 9 provide the estimates of VaRs and ESs for the considered distributions based on Data Sets 1 and 2.
From these tables, it can be concluded that the ELo distribution has higher values of both the risk measures as compared to its counterparts, the ExLo, Lo, and Da distributions. The graphical demonstration of this statement can be seen in Figs. 14 and 15, where it is also revealed that the ELo distribution has a heavier tail than the ExLo, Lo, and Da distributions. The reader is referred to [53] for a detailed discussion of VaR and ES and their computation by using an R package.
Figure 14: Plots of the estimated (a) ES and (b) VaR of the conidered distributions for Data Set 1
Figure 15: Plots of the estimated (a) ES and (b) VaR of the conidered distributions for Data Set 2
In this paper, we introduced a new three-parameter heavy-tailed extension of the Lomax distribution. It can be derived from a simple transformation of an existing unit distribution: the log-weighted power distribution. Some mathematical and statistical properties are derived, including the shapes of related probability and hazard rate functions; stochastic comparisons; manageable expansions for various moments; and quantile properties. Unknown parameters are estimated using the maximum likelihood method. A complete simulation study validates the accuracy of the obtained estimates. Two applications, each with its own plots, are provided to demonstrate the importance of the extended Lomax distribution. In particular, we show that it outperforms eight comparable distributions in the literature. Because of the heavy-tailed nature of the considered distribution, actuarial measures of importance are emphasized. Based on these actuarial measures, we show that it yields superior conclusions from fair competitor distributions.
We believe that the proposed methodology can be used quite efficiently to analyze data presenting a heavy-tail, especially for those having acceptable results with the Lomax distribution; the extended Lomax distribution will probably do the analysis in a more precise manner. Possible further work includes bivariatization and discretization of the extended Lomax distribution for different modeling objectives.
Acknowledgement: The authors would like to thank the three reviewers for their constructive comments on the paper.
Funding Statement: This project was funded by the Deanship Scientific Research (DSR), King Abdulaziz University, Jeddah, under the Grant No. KEP-PhD:21-130-1443. The authors acknowledge with thanks DSR for technical and financial support.
Availability of Data and Materials: The data used in this article are freely available in the mentioned references.
Conflicts of Interest: The authors declare that they have no conflicts of interest to report regarding the present study.
References
1. Balkema, A. A., de Haan, L. (1974). Residual life time at great age. The Annals of Probability, 2(5), 792–804. https://doi.org/10.1214/aop/1176996548 [Google Scholar] [CrossRef]
2. Bryson, M. C. (1974). Heavy-tailed distributions: Properties and tests. Technometrics, 16(1), 61–68. https://doi.org/10.1080/00401706.1974.10489150 [Google Scholar] [CrossRef]
3. Ahsanullah, M. (1991). Record values of Lomax distribution. Statistica Neerlandica, 41(1), 21–29. https://doi.org/10.1111/j.1467-9574.1991.tb01290.x [Google Scholar] [CrossRef]
4. Balakrishnan, N., Ahsanullah, M. (1994). Relations for single and product moments of record values from Lomax distribution. Sankhya B, 56(2), 140–146. [Google Scholar]
5. Childs, A., Balakrishnan, N., Moshref, M. (2001). Order statistics from non-identical right truncated Lomax random variables with applications. Statistical Papers, 42(2), 187–206. https://doi.org/10.1007/s003620100050 [Google Scholar] [CrossRef]
6. Arnold, B. C., Castillo, E., Sarabia, J. M. (1998). Bayesian analysis for classical distributions using conditionally specified priors. Sankhya B, 60(2), 228–245. [Google Scholar]
7. Howlader, H. A., Hossain, A. M. (2002). Bayesian survival estimation of Pareto distribution of the second kind based on failure censored data. Computational Statistics & Data Analysis, 38(3), 301–314. https://doi.org/10.1016/S0167-9473(01)00039-1 [Google Scholar] [CrossRef]
8. Cramer, E., Schmied, A. B. (2001). Progressively type-II censored competing risks data from Lomax distributions. Computational Statistics & Data Analysis, 55(3), 1285–1303. https://doi.org/10.1016/j.csda.2010.09.017 [Google Scholar] [CrossRef]
9. Al-Zahrani, B., Al-Sobhi, M. (2013). On parameters estimation of Lomax distribution under general progressive censoring. Journal of Quality and Reliability Engineering, 2013, 7, 431541. https://doi.org/10.1155/2013/431541 [Google Scholar] [CrossRef]
10. Johnson, N., Kotz, S., Balakrishnan, N. (1994). Continuous univariate distribution, 2nd edition, vol. 1. New York: Wiley. [Google Scholar]
11. Ghitany, M. E., Al-Awadhi, F. A., Alkhalfan, L. A. (2007). Marshall-Olkin extended Lomax distribution and its application to censored data. Communications in Statistics−Theory and Methods, 36(10), 1855–1866. https://doi.org/10.1080/03610920601126571 [Google Scholar] [CrossRef]
12. Al-Zahrani, B., Sagor, H. (2015). Statistical analysis of the Lomax-logarithmic distribution. Journal of Statistical Computation and Simulation, 85(9), 1883–1901. https://doi.org/10.1080/00949655.2014.907800 [Google Scholar] [CrossRef]
13. Abdul-Moniem, I. B. (2012). Recurrence relations for moments of lower generalized order statistics from exponentiated Lomax distribution and its characterization. Journal of Mathematical and Computational Science, 2, 999–1011. [Google Scholar]
14. Rajab, M., Aleem, M., Nawaz, T., Daniyal, M. (2013). On five parameter beta Lomax distribution. Journal of Statistics, 20(1), 102–118. [Google Scholar]
15. Al-Jarallah, R. A., Ghitany, M. E., Gupta, R. C. (2014). A proportional hazard Marshall-Olkin extended family of distributions and its application to Gompertz distribution. Communications in Statistics-Theory and Methods, 43(20), 4428–4443. https://doi.org/10.1080/03610926.2012.715226 [Google Scholar] [CrossRef]
16. El-Bassiouny, A. H., Abdo, N. F., Shahen, H. S. (2015). Exponential Lomax distribution. International Journal of Computer Applications, 121(13), 24–29. https://doi.org/10.5120/21602-4713 [Google Scholar] [CrossRef]
17. Cordeiro, G. M., Ortega, E. M., Popovic, B. V. (2015). The gamma-Lomax distribution. Journal of Statistical Computation and Simulation, 85(2), 305–319. https://doi.org/10.1080/00949655.2013.822869 [Google Scholar] [CrossRef]
18. Tahir, M. H., Cordeiro, G. M., Mansoor, M., Zubair, M. (2015). The Weibull-Lomax distribution: Properties and applications. Hacettepe Journal of Mathematics and Statistics, 44(2), 455–474. [Google Scholar]
19. Mead, M. E. (2016). On five-parameter Lomax distribution: Properties and applications. Pakistan Journal of Statistics and Operation Research, 12(1), 185–200. https://doi.org/10.18187/pjsor.v11i4.1163 [Google Scholar] [CrossRef]
20. Rady, E. H. A., Hassanein, W. A., Elhaddad, T. A. (2016). The power Lomax distribution with an application to bladder cancer data. SpringerPlus, 5(1), 1–22. https://doi.org/10.1186/s40064-016-3464-y [Google Scholar] [PubMed] [CrossRef]
21. Hassan, A. S., Abd-Allah, M. (2018). Exponentiated Weibull-Lomax distribution: Properties and estimation. Journal of Data Science, 16(2), 277–298. [Google Scholar]
22. Elbiely, M. M., Yousof, H. M. (2019). A new extension of the Lomax distribution and its applications. Journal of Statistics and Applications, 2(1), 18–34. [Google Scholar]
23. Nagarjuna, B. V., Vardhan, R. V. (2020). Marshall-Olkin exponential Lomax distribution: Properties and its application. Stochastic Modelling and Applications, 24, 161–177. [Google Scholar]
24. Al-Marzouki, S., Jamal, F., Chesneau, C., Elgarhy, M. (2020). Type II Topp-Leone power Lomax distribution with applications. Mathematics, 8(1), 1–26. [Google Scholar]
25. Mathew, J., Chesneau, C. (2020). Some new contributions on the Marshall-Olkin length biased Lomax distribution: Theory, modelling and data analysis. Mathematical and Computational Applications, 25(79), 1–21. https://doi.org/10.3390/mca25040079 [Google Scholar] [CrossRef]
26. Nagarjuna, B. V., Vardhan, R. V., Chesneau, C. (2021a). Kumaraswamy generalized power Lomax distribution and its applications. Stats, 4(1), 28–45. https://doi.org/10.3390/stats4010003 [Google Scholar] [CrossRef]
27. Ali, M. M., Yousof, H. M., Ibrahim, M. (2021). A new Lomax type distribution: Properties, copulas, applications, Bayesian and non-Bayesian estimation methods. International Journal of Statistical Sciences, 21(2), 61–104. [Google Scholar]
28. Nagarjuna, B. V., Vardhan, R. V., Chesneau, C. (2021b). On the accuracy of the sine power Lomax model for data fitting. Modelling, 2(1), 78–104. https://doi.org/10.3390/modelling2010005 [Google Scholar] [CrossRef]
29. Nagarjuna, V. B. V., Vardhan, R. V., Chesneau, C. (2022). Nadarajah-Haghighi Lomax distribution and its applications. Mathematical and Computational Applications, 27(30), 1–13. https://doi.org/10.3390/mca27020030 [Google Scholar] [CrossRef]
30. Almarashi, A. M. (2021). A new modified inverse Lomax distribution: Properties, estimation and applications to engineering and medical data. Computer Modeling in Engineering & Sciences, 127(2), 621–643. https://doi.org/10.32604/cmes.2021.014407 [Google Scholar] [CrossRef]
31. Abiodun, A. A., Ishaq, A. I. (2022). On Maxwell-Lomax distribution: Properties and applications. Arab Journal of Basic and Applied Sciences, 29(1), 221–232. https://doi.org/10.1080/25765299.2022.2093033 [Google Scholar] [CrossRef]
32. Khan, S., Hamedani, G. G., Reyad, H. M., Jamal, F., Shafiq, S. et al. (2022). The minimum Lindley Lomax distribution: Properties and applications. Mathematical and Computational Applications, 27(1), 16. https://doi.org/10.3390/mca27010016 [Google Scholar] [CrossRef]
33. Falgore, J. Y., Doguwa, S. I. (2022). On new Weibull inverse Lomax distribution with applications. Baghdad Science Journal, 19(3), 0528. [Google Scholar]
34. Aboraya, M., Ali, M. M., Yousof, H. M., Ibrahim, M. (2022). A novel Lomax extension with statistical properties, copulas, different estimation methods and applications. Bulletin of the Malaysian Mathematical Sciences Society, 45(Suppl 1), 85–120. [Google Scholar]
35. Lomax, K. S. (1954). Business failures; another example of the analysis of failure data. Journal of the American Statistical Association, 49, 847–852. https://doi.org/10.1080/01621459.1954.10501239 [Google Scholar] [CrossRef]
36. Chesneau, C. (2021). On a logarithmic weighted power distribution: Theory, modelling and applications. Journal of Mathematical Sciences: Advances and Applications, 67(1), 1–59. [Google Scholar]
37. Gómez-Déniz, E., Sordo, M. A., Calderín-Ojeda, E. (2014). The logLindley distribution as an alternative to the beta regression model with applications in insurance. Insurance: Mathematics and Economics, 54, 49–57. [Google Scholar]
38. Shaked, M., Shanthikumar, J. G. (1994). Stochastic orders and their applications. Boston: Academic Press [Google Scholar]
39. Bawa, V. S. (1975). Optimal rules for ordering uncertain prospects. Journal of Financial Economics, 2(1), 95–121. https://doi.org/10.1016/0304-405X(75)90025-2 [Google Scholar] [CrossRef]
40. Müller, A., Stoyan, D. (2002). Comparison methods for stochastic models and risks. Chichester: John Wiley & Sons. [Google Scholar]
41. Chamberlain, G. (1987). Asymptotic efficiency in estimation with conditional moment restrictions. Journal of Econometrics, 34(3), 305–334. https://doi.org/10.1016/0304-4076(87)90015-7 [Google Scholar] [CrossRef]
42. Kim, J. H. T. (2010). Conditional tail moments of the exponential family and its related distributions. North American Actuarial Journal, 14, 198–216. https://doi.org/10.1080/10920277.2010.10597585 [Google Scholar] [CrossRef]
43. Oakes, D., Desu, M. M. (1990). A note on residual life. Biometrica, 77, 409–410. https://doi.org/10.1093/biomet/77.2.409 [Google Scholar] [CrossRef]
44. Dress, H., Reiss, R. D. (1996). Residual life functionals at greate age. Communications in Statistics-Theory and Methods, 25(4), 823–835. https://doi.org/10.1080/03610929608831734 [Google Scholar] [CrossRef]
45. Ebrahimi, N. (1998). Estimating the finite population versions of mean residual life-time function and hazard function using Bayes method. Annals of the Institute of Statistical Mathematics, 50(1), 15–27. https://doi.org/10.1023/A:1003493112915 [Google Scholar] [CrossRef]
46. Galton, F. (1883). Enquiries into human faculty and its development. London: Macmillan & Company. [Google Scholar]
47. Moors, J. J. (1988). A quantile alternative for kurtosis. Statistician, 37, 25–32. https://doi.org/10.2307/2348376 [Google Scholar] [CrossRef]
48. Artzner, P., Delbaen, F., Eber, J. M., Heath, D. (1999). Coherent measures of risk. Mathematical Finance, 9(3), 203–228. https://doi.org/10.1111/1467-9965.00068 [Google Scholar] [CrossRef]
49. Gilchrist, W. (1997). Modelling with quantile distribution functions. Journal of Applied Statistics, 24(1), 113–122. https://doi.org/10.1080/02664769723927 [Google Scholar] [CrossRef]
50. Casella, G., Berger, R. L. (1990). Statistical inference. Bel Air, CA, USA: Brooks/Cole Publishing Company. [Google Scholar]
51. Algarni, A., Almarashi, A. M. (2022). A new Rayleigh distribution: Properties and estimation based on progressive type-II censored data with an application. Computer Modeling in Engineering & Sciences, 130(1), 379–396. https://doi.org/10.32604/cmes.2022.017714 [Google Scholar] [CrossRef]
52. Kleiber, C., Kotz, S. (2003). Statistical size distributions in economics and actuarial science. Wiley series in probability and statistics. Hoboken, New Jersey: Wiley Interscience, John Wiley and Sons Inc. [Google Scholar]
53. Chen, X., Chi, Y., Tan, K. S. (2016). The design of an optimal retrospective rating plan. ASTIN Bulletin, 46(1), 141–163. https://doi.org/10.1017/asb.2015.19 [Google Scholar] [CrossRef]
Cite This Article
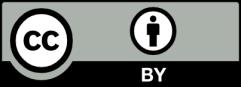
This work is licensed under a Creative Commons Attribution 4.0 International License , which permits unrestricted use, distribution, and reproduction in any medium, provided the original work is properly cited.