Open Access
ARTICLE
Predicting the Thickness of an Excavation Damaged Zone around the Roadway Using the DA-RF Hybrid Model
1
School of Resources and Safety Engineering, Central South University, Changsha, 410083, China
2
Laboratory 3SR, CNRS UMR 5521, Grenoble Alpes University, Grenoble, 38000, France
* Corresponding Authors: Jian Zhou; Email: ;
(This article belongs to the Special Issue: Computational Intelligent Systems for Solving Complex Engineering Problems: Principles and Applications)
Computer Modeling in Engineering & Sciences 2023, 136(3), 2507-2526. https://doi.org/10.32604/cmes.2023.025714
Received 26 July 2022; Accepted 28 October 2022; Issue published 09 March 2023
Abstract
After the excavation of the roadway, the original stress balance is destroyed, resulting in the redistribution of stress and the formation of an excavation damaged zone (EDZ) around the roadway. The thickness of EDZ is the key basis for roadway stability discrimination and support structure design, and it is of great engineering significance to accurately predict the thickness of EDZ. Considering the advantages of machine learning (ML) in dealing with high-dimensional, nonlinear problems, a hybrid prediction model based on the random forest (RF) algorithm is developed in this paper. The model used the dragonfly algorithm (DA) to optimize two hyperparameters in RF, namely mtry and ntree, and used mean absolute error (MAE), root mean square error (RMSE), determination coefficient (R2), and variance accounted for (VAF) to evaluate model prediction performance. A database containing 217 sets of data was collected, with embedding depth (ED), drift span (DS), surrounding rock mass strength (RMS), joint index (JI) as input variables, and the excavation damaged zone thickness (EDZT) as output variable. In addition, four classic models, back propagation neural network (BPNN), extreme learning machine (ELM), radial basis function network (RBF), and RF were compared with the DA-RF model. The results showed that the DARF mold had the best prediction performance (training set: MAE = 0.1036, RMSE = 0.1514, R2 = 0.9577, VAF = 94.2645; test set: MAE = 0.1115, RMSE = 0.1417, R2 = 0.9423, VAF = 94.0836). The results of the sensitivity analysis showed that the relative importance of each input variable was DS, ED, RMS, and JI from low to high.Keywords
Cite This Article
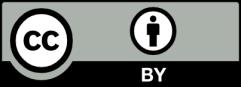