Open Access
ARTICLE
MAQMC: Multi-Agent Deep Q-Network for Multi-Zone Residential HVAC Control
1
School of Electronic and Information Engineering, Suzhou University of Science and Technology, Suzhou, 215009, China
2
Jiangsu Province Key Laboratory of Intelligent Building Energy Eciency, Suzhou University of Science and Technology,
Suzhou, 215009, China
3
School of Architecture and Urban Planning, Suzhou University of Science and Technology, Suzhou, 215009, China
4 Chongqing Industrial Big Data Innovation Center Co., Ltd., Chongqing, 400707, China
* Corresponding Authors: Qiming Fu. Email: ; Jianping Chen. Email:
(This article belongs to the Special Issue: Advanced Intelligent Decision and Intelligent Control with Applications in Smart City)
Computer Modeling in Engineering & Sciences 2023, 136(3), 2759-2785. https://doi.org/10.32604/cmes.2023.026091
Received 15 August 2022; Accepted 01 November 2022; Issue published 09 March 2023
Abstract
The optimization of multi-zone residential heating, ventilation, and air conditioning (HVAC) control is not an easy task due to its complex dynamic thermal model and the uncertainty of occupant-driven cooling loads. Deep reinforcement learning (DRL) methods have recently been proposed to address the HVAC control problem. However, the application of single-agent DRL for multi-zone residential HVAC control may lead to non-convergence or slow convergence. In this paper, we propose MAQMC (Multi-Agent deep Q-network for multi-zone residential HVAC Control) to address this challenge with the goal of minimizing energy consumption while maintaining occupants’ thermal comfort. MAQMC is divided into MAQMC2 (MAQMC with two agents:one agent controls the temperature of each zone, and the other agent controls the humidity of each zone) and MAQMC3 (MAQMC with three agents:three agents control the temperature and humidity of three zones, respectively). The experimental results show that MAQMC3 can reduce energy consumption by 6.27% and MAQMC2 by 3.73% compared with the fixed point; compared with the rule-based, MAQMC3 and MAQMC2 respectively can reduce 61.89% and 59.07% comfort violation. In addition, experiments with different regional weather data demonstrate that the well-trained MAQMC RL agents have the robustness and adaptability to unknown environments.Keywords
Cite This Article
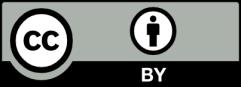
This work is licensed under a Creative Commons Attribution 4.0 International License , which permits unrestricted use, distribution, and reproduction in any medium, provided the original work is properly cited.