Open Access
ARTICLE
Evaluating the Potentials of PLSR and SVR Models for Soil Properties Prediction Using Field Imaging, Laboratory VNIR Spectroscopy and Their Combination
1
Center of Mapping and Remote Sensing, Tunis, 1073, Tunisia
2
Department of Information Technology, College of Computer and Information Sciences, Princess Nourah bint Abdulrahman
University, P.O.Box 84428, Riyadh, 11671, Saudi Arabia
3
Digital Research Centre of Sfax, Sfax, 3021, Tunisia
4
Higher School of Agriculture of Mograne, LR03AGR02, SPADD, University of Carthage, Carthage, 1054, Tunisia
* Corresponding Author: Hela Elmannai. Email:
Computer Modeling in Engineering & Sciences 2023, 136(2), 1399-1425. https://doi.org/10.32604/cmes.2023.023164
Received 12 April 2022; Accepted 14 October 2022; Issue published 06 February 2023
Abstract
Pedo-spectroscopy has the potential to provide valuable information about soil physical, chemical, and biological properties. Nowadays, we may predict soil properties using VNIR field imaging spectra (IS) such as Prisma satellite data or laboratory spectra (LS). The primary goal of this study is to investigate machine learning models namely Partial Least Squares Regression (PLSR) and Support Vector Regression (SVR) for the prediction of several soil properties, including clay, sand, silt, organic matter, nitrate NO3-, and calcium carbonate CaCO3, using five VNIR spectra dataset combinations (% IS, % LS) as follows: C1 (0% IS, 100% LS), C2 (20% IS, 80% LS), C3 (50% IS, 50% LS), C4 (80% IS, 20% LS) and C5 (100% IS, 0% LS). Soil samples were collected at bare soils and at the upper (0–30 cm) layer. The data set has been split into a training dataset 80% of the collected data (n = 248) and a validation dataset 20% of the collected data (n = 61). The proposed PLSR and SVR models were trained then tested for each dataset combination. According to our results, SVR outperforms PLSR for both: C1 (0% IS, 100% LS) and C5 (100% IS, 0% LS). For Soil Organic Matter (SOM) prediction, it achieves (R2 = 0.79%, RMSE = 1.42%) and (R2 = 0.76%, RMSE = 1.3%), respectively. The data fusion has improved the soil property prediction. The highest improvement was obtained for the SOM property (R2 = 0.80%, RMSE = 1.39) when using the SVR model and applying the second Combination C2 (20% of IS and 80% LS).Graphic Abstract
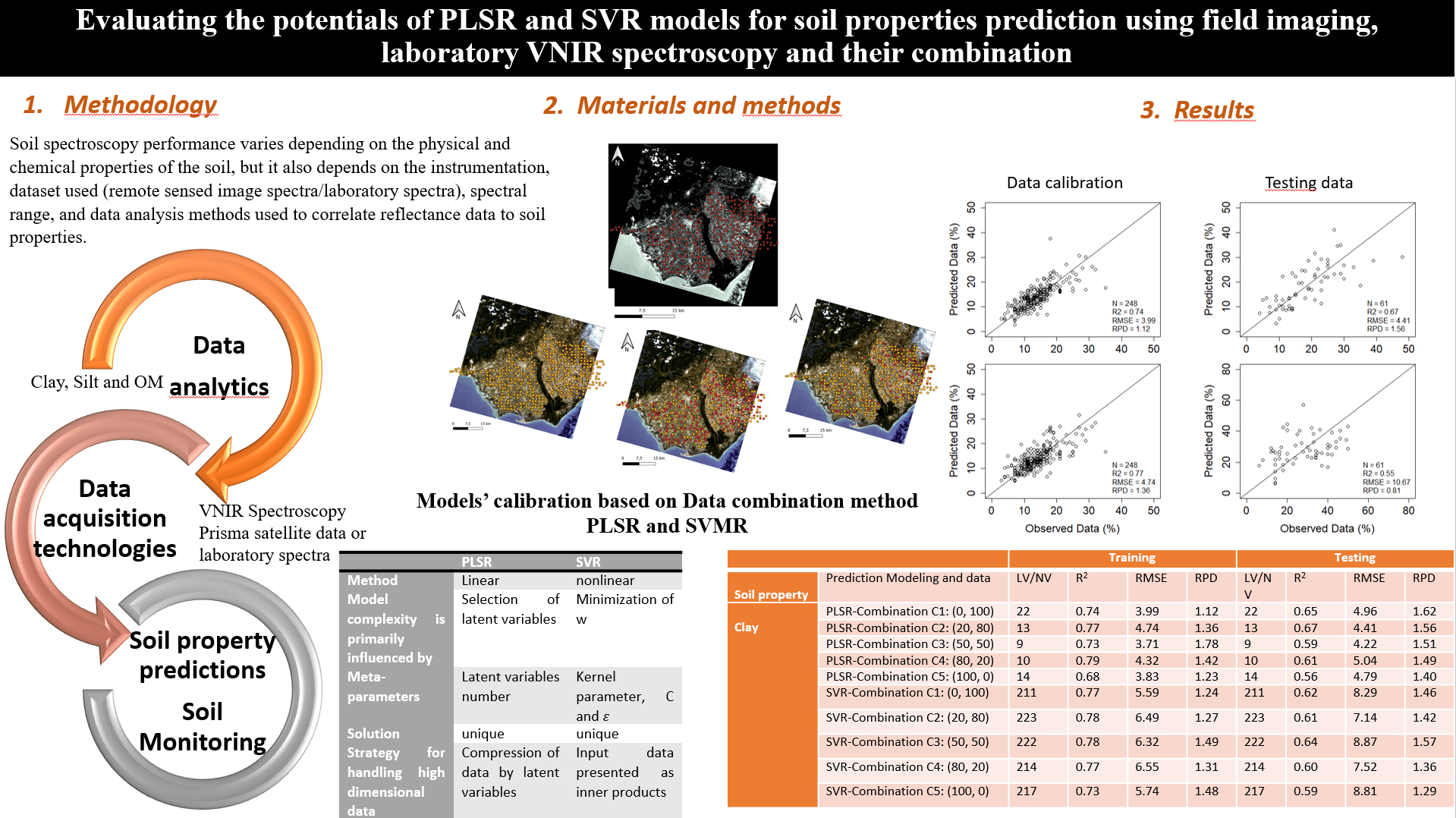
Keywords
Cite This Article
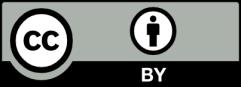
This work is licensed under a Creative Commons Attribution 4.0 International License , which permits unrestricted use, distribution, and reproduction in any medium, provided the original work is properly cited.