Open Access
ARTICLE
IRMIRS: Inception-ResNet-Based Network for MRI Image Super-Resolution
1
Department of Electrical Engineering, Balochistan University of Engineering and Technology, Khuzdar, 89100, Pakistan
2
Department of Computer Systems Engineering, Balochistan University of Engineering and Technology, Khuzdar, 89100, Pakistan
3
Department of Mechanical Engineering, Balochistan University of Engineering and Technology, Khuzdar, 89100, Pakistan
4
Department of Mechanical Engineering, National Taiwan University of Science and Technology, 10607, Taiwan
5
Department of Information Systems, Kyungsung University, Busan, 613010, South Korea
* Corresponding Author: Zuhaibuddin Bhutto. Email:
Computer Modeling in Engineering & Sciences 2023, 136(2), 1121-1142. https://doi.org/10.32604/cmes.2023.021438
Received 14 January 2022; Accepted 24 June 2022; Issue published 06 February 2023
Abstract
Medical image super-resolution is a fundamental challenge due to absorption and scattering in tissues. These challenges are increasing the interest in the quality of medical images. Recent research has proven that the rapid progress in convolutional neural networks (CNNs) has achieved superior performance in the area of medical image super-resolution. However, the traditional CNN approaches use interpolation techniques as a preprocessing stage to enlarge low-resolution magnetic resonance (MR) images, adding extra noise in the models and more memory consumption. Furthermore, conventional deep CNN approaches used layers in series-wise connection to create the deeper mode, because this later end layer cannot receive complete information and work as a dead layer. In this paper, we propose Inception-ResNet-based Network for MRI Image Super-Resolution known as IRMRIS. In our proposed approach, a bicubic interpolation is replaced with a deconvolution layer to learn the upsampling filters. Furthermore, a residual skip connection with the Inception block is used to reconstruct a high-resolution output image from a low-quality input image. Quantitative and qualitative evaluations of the proposed method are supported through extensive experiments in reconstructing sharper and clean texture details as compared to the state-of-the-art methods.Keywords
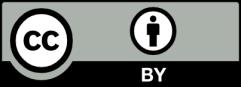