Open Access
REVIEW
Modeling Methods of 3D Model in Digital Twins
1
School of Computer Science and Engineering, Beijing Technology and Business University, Beijing, 100080, China
2
Department of Game Design, Faculty of Art, Uppsala University, Uppsala, S-75105, Sweden
* Corresponding Author: Haisheng Li. Email:
Computer Modeling in Engineering & Sciences 2023, 136(2), 985-1022. https://doi.org/10.32604/cmes.2023.023154
Received 12 April 2022; Accepted 07 September 2022; Issue published 06 February 2023
Abstract
To understand the current application and development of 3D modeling in Digital Twins (DTs), abundant literatures on DTs and 3D modeling are investigated by means of literature review. The transition process from 3D modeling to DTs modeling is analyzed, as well as the current application of DTs modeling in various industries. The application of 3D DTs modeling in the fields of smart manufacturing, smart ecology, smart transportation, and smart buildings in smart cities is analyzed in detail, and the current limitations are summarized. It is found that the 3D modeling technology in DTs has broad prospects for development and has a huge impact on all walks of life and even human lifestyles. At the same time, the development of DTs modeling relies on the development and support capabilities of mature technologies such as Big Data, Internet of Things, Cloud Computing, Artificial Intelligence, and game technology. Therefore, although some results have been achieved, there are still limitations. This work aims to provide a good theoretical support for the further development of 3D DTs modeling.Keywords
With the perfection of infrastructure applications, including Big Data (BD) and cloud computing, many advanced technologies continue to advance, such as Artificial Intelligence (AI), Internet of Things (IoT), and Digital Twins (DTs) and the maturity of deep learning and general algorithm, the development of DTs architecture has ushered in an excellent opportunity [1–3]. However, the current 3D modeling objects for real scenes focus on natural entities and man-made entities, and provide very limited information. However, production practice requires more information, especially the semantic correlation between key elements such as landscapes, forests, and fields [4–6]. The DTs model is becoming more and more popular in the infrastructure industry [7–9]. It can drive changes in our way of life, and not just the technological advantages that people talk about, the DTs model will also drive the development of the entire infrastructure industry [10–12]. DTs model will transform the design, delivery, and management of infrastructure, enabling more resilient and sustainable infrastructure. The more accessible the information of the modeling platform and the more open the platform is, the greater the opportunity for data to be repurposed and value created.
In the field of smart buildings, BIM technology is a perfect information model. It completely stores the data resources and processes data of different stages of the project in each family unit, comprehensively describes the information of building objects, and realizes automatic queries, splitting, and combining to extract real-time information [13–15]. Applying BIM in the construction industry for DTs modeling is of significant practical value, but it takes a long time to realize. For such a standardized product, DTs have many difficulties, not to mention every complex building. Building a DTs body covering the life cycle of a product helps to establish a closed-loop system from R&D, simulation, and manufacturing to use, which accelerates product development and iterative upgrades. At the same time, the DTs body can become an innovative experimental sandbox. Once due to security issues and physical conditions, many operations could not be completed on real physical entities. Now DTs make it possible to test and refine products through techniques such as performance simulation, virtual assembly, and fault rehearsal [16–18]. The construction of DTs body of the whole industry chain from customers, market demand, supply chain and logistics system, maintenance, and security, etc., and the integration of systems engineering based on models in various fields will help to promote the transformation of traditional industries to customized production models and realize an agile and flexible business model. DTs include seven aspects of information modeling, synchronization, enhancement, analysis, intelligent decision-making, access interface, and security. Although many achievements have been made so far, it is still undergoing rapid evolution [19–21]. With the common development of a series of emerging technologies, such as a new generation of information technology, advanced manufacturing technology, and new material technology, the above elements will continue to be optimized, and the development of DTs will be optimized and perfected while exploring and trying.
The adoption status of DTs 3D modeling in various industries is analyzed by means of literature review, especially the adoption of DTs modeling in smart manufacturing and smart city construction. The conclusions drawn can provide new ideas, theoretical basis, and new research directions for the future research and development of DTs 3D modeling.
2 From Realistic 3D Modeling to DTs Modeling
Real-world 3D modeling is not projecting the world completely to the computer and modeling all the geographical scene elements in detail [22–24]. The purpose of modeling is to make computers help with analysis and computation, which means making choices and trade-offs from the real world [25]. Even DTs do not require the original copy of the original, but to eliminate some factors that interfere with computer analysis and calculation while retaining the core elements. These models should reflect the essential characteristics of things, while isolating details and mechanisms that do not play an active role in analytical calculations. Therefore, real scene 3D should provide models with different granularities, different modes, and different scales regarding the needs of different management levels and different professions. At the same time, it is only possible to achieve large-scale promotion through standardization. A DTs model is a digital physical asset, process, or system and engineering information. Continuous updates of DTs models are realized via multiple sources such as sensors to reflect the status and operating conditions in near real-time. Using the DTs model, users can visualize assets, perform analytics, and generate deep insights to predict and optimize asset performance.
There are currently two typical 3D models of real scenes. One is in rural areas. Since people live in a scattered way, the buildings do not have complex rooms, floors, etc., and there are no staggered pipeline facilities in the underground. Therefore, its monolithic, semantic, and structuring is logically simple. The other is urban areas, where there are many public spaces above ground and basements, and the physical boundaries need to be clearly sorted out [26–28]. Therefore, in these two typical different scenarios, the 3D modeling accuracy of the real scene is different, the level of detail is different, and the situation is even more different. Faced with how to manage all natural resource elements as a whole and systematically and fabricate a new 3D representation model of the real scene, it is necessary to express not only the visible representation data, but also the mechanism model. For example, for water bodies, it is not only about the area, but also about the water quality. For buildings, it is not only about the number of floors and building area, but also about its structure, materials, functions, etc. The entity constructed in this way has real meaning. Otherwise, it is just a physical unit and a virtual space. By carrying professional information, it supports the most important prediction, examination, and decision-making for modeling. Otherwise, it is a reference on the map, and its value is very limited. Taking the integrated solution of real 3D modeling as an example, the modeling process is shown in Fig. 1.
Figure 1: Example of an integrated solution for 3D modeling of reality
From Fig. 1, the technology of real 3D modeling is still under research, and the development of DTs technology puts forward new requirements for 3D modeling. The biggest difference between it and real 3D is that it not only aims at natural entities and artificial entities, but also aims at other elements of human-machine ternary space to model and reflect their behaviors, namely real-time dynamics. From real-scene 3D modeling to DTs modeling, the upgrade of digital requirements has realized the transformation from data to information to knowledge and then to wisdom. For example, the “urban brain” proposed by Alibaba (Fig. 2) was originally aimed at controlling urban traffic congestion. It was later found that even if the efficiency of urban traffic congestion was improved by 10% or so, it could not be further improved, because the treatment and solution of traffic congestion were comprehensive factors.
Figure 2: Alibaba AI industry layout
Urban brain construction requires preconditions and foundations (Fig. 3), and only on the premise of establishing these infrastructures can the urban brain be truly realized. Therefore, Alibaba changed its main aim from “deal with traffic jams” to “deal with cities”. If the city is well managed, traffic congestion will naturally be solved. This comprehensive holistic view is the greatest value of DTs.
Figure 3: The foundation on which the urban brain is built
Figs. 2 and 3 show that the establishment of the urban brain is inseparable from the support of DTs technology. There are two types of models in the virtual DTs body. One is the static environment model, which makes the DTs system have a better visual performance so that people produce enough immersion. The other is the dynamic motion model, which maintains the dynamic consistency between the physical space and the virtual DTs body, which is dynamic. Taking the construction of a 3D model of the campus environment as an example, the real-scene modeling technology of tilted image is selected. The tilted photography-based real-scene modeling flow chart is shown in Fig. 4.
Figure 4: Oblique photography real scene modeling process
Fig. 4 shows that the tilt photography-based real scene modeling process is divided into two parts. One part is the data acquisition of oblique photography in the field. The other part is the processing of tilted photographic data. The change brought by DTs promotes the continuous upgrading of the urban brain, from local optimal to global optimal, from partial cognition to overall cognition, and from dominant characteristics to hidden rule discovery. The DTs modeling process is shown in Fig. 5.
Figure 5: DTs modeling process
Fig. 5 shows that the DTs model is compared with various existing digital representations, such as Computer Aided Design (CAD), BIM, and Geographic Information System (GIS). The most prominent feature is that it integrates the real-time data of the life cycle of the human-machine-object ternary space, and continuously iteratively optimizes with the changes of physical entities. In addition to accurately mapping the perception of IoT sensors and the physical entity characteristics reflected by the obtained characterization data, the DTs model also accurately maps its behavioral laws and the mechanism of mutual feedback. Moreover, in many cases, the representation data that can be obtained due to the limitations of the perception means are not complete, refined, accurate, and timely, and only model knowledge can be taken for inference. The DTs model reflects an iterative process. For example, in tunnel construction, it is necessary to extract all elements and analyze the relationship between them. In this way, it is possible to know what data is needed, what technology to use, and how to deal with emergencies, thereby effectively controlling quality, construction progress, and future economic benefits. Therefore, for the city, the DTs model is a system of continuous iterative interpretation of the virtual and the real, and the interpretation of the planning and construction itself, while the 3D modeling of the real scene is completely different.
DTs models are surpassing BIM, and engineering, operations, and information technologies of asset-centric organizations are jointed for immersive visualization and analytical visibility. Lv et al. [29] fabricated a cognitive computing system model combining cognitive computing and DBN algorithms with collaborative robots for the control system of collaborative robots. These capabilities are made possible by the fusion of capabilities or technologies in 3D visualization, reality modeling, mixed reality, and geotechnical engineering, which helps teams gain an immersive holistic view of infrastructure assets. DTs is the virtual forms of physical assets through data and simulators for real-time prediction, monitoring, control, and improvement of decision-making. Computational pipelines, multi-physics solvers, AI, and BD cybernetics bring the prospect of DTs closer to reality. DTs is computational mega-models, device shadows, mirror systems, avatars, or synchronized virtual prototypes. Undoubtedly, DTs decides how to design and operate cyber-physical intelligent systems, as well as how to advance the modularization of multidisciplinary systems to address current unresolved obstacles [30].
Manufacturing of high-performance aerospace components requires rigorous process geometry and performance-based quality control. Real-time observation, understanding, and control of the process are an integral part of optimizing the manufacturing strategy for aerospace parts. Taking the intelligent information management platform as an example, the content needed is shown in Fig. 6.
Figure 6: Aerospace intelligent information management platform
Fig. 6 shows that DTs can be used to model, monitor, and control the machining process by integrating multi-dimensional context machining process data, such as changes in geometry, material properties, and machining parameters. However, a systematic modeling scheme which can adaptively develop high-fidelity multi-scale and multi-dimensional DTs is required. Liu et al. [31] proposed a DTs modeling method according to the principle of bionics to construct the multi-physics DTs volume of machining. Thus, multiple sub-models of DTs, such as geometrical, behavioral, and procedural models, are developed. They interact with each other and constitute a true representation of the physical machining process. Cyber-Physical Production System (CPPS) may pave the way for exploring novel intelligent manufacturing solutions. DTs are the core of CPPS establishment in Industry 4.0, so an easy-to-deploy and easy-to-use DTs-based CPPS is a key stage. Shangguan et al. [32] proposed a triple human-digital twin architecture for Cyber-Physical Systems. Liu et al. [33] proposed a system framework that integrates Cyber-Physical System (CPS), DTs 3D modeling technology, event-driven distributed collaboration mechanism, and Web technology, which provides guidance for quick system configuration and easy operation of DTs-based CPPS.
DTs represent the convergence of information and physical domains to bridge materials and virtual worlds. Existing DTs modeling approaches are mainly based on modular representation, which limits the guidance of the modeling process. These approaches do not consider the components or operational rules of DTs in detail, preventing designers from applying them in their domain. With the increasing application of DTs bodies in various engineering fields (Fig. 7), an effective method of modeling multidimensional DTs bodies at the conceptual level is needed.
Figure 7: DTs connects old and new infrastructure
Fig. 7 shows that the new and old infrastructures have different technological standards due to different construction time and procedures. After visualization of the interior of the building through DTs, the connection between the new and old infrastructures can be easily and intuitively carried out. Thus, Wu et al. [34] proposed a conceptual modeling method for DTs based on a 5D DTs framework that can illustrate relationships that are complex between DTs objects and their attributes. It worked well in modeling the DTs volume of intelligent vehicles at the conceptual level. A virtual representation of the product lifecycle is provided, enabling the prediction and optimization of the production systems and their components. Schroeder et al. [35] proposed a flexible and universal method using model-driven engineering. This method has two levels. First, DTs are modeled as a combination of components providing basic functions, such as identification, storage, communication, and simulation. Second, aggregated DT is a hierarchical combination of other DTs. AutomationML was used to propose a general reference architecture and specific implementation methods based on these concepts.
Although the ambiguity of the traditional document-based approach is enhanced by Model-Based Systems Engineering (MBSE), there is certain management complexity. The core concern is how to effectively assess, predict, and manage it during the designing. Due to inconsistencies between the physical actual design process and virtual theoretical simulations, the contemporary complexity measurement method is not accurate. DTs offer an approach to address this problem by bridging physical and virtual. To combine DTs with MBSE for complexity analysis and prediction, Wang et al. [36] introduced an integrated framework named 5D DTs system design from a knowledge perspective based on previous work, which provides design complexity measurement, effort estimation, and change propagation prediction.
Building a DTs platform network means that a comprehensive perspective on products, manufacturing, supply chain, profitability, etc., is provided, which is conducive to sustainable model fabrication. Li et al. [37] explored how enterprises apply the DTs platform to generate benefits, as well as their coupling relationships in various aspects. A 5D framework of sustainable business models was constructed, and the coupling relationship was analyzed. Taking Haier as an example, the mechanism of the Haier DTs platform is explored, an integration framework under coupling perspective is constructed. The results showed that through DTs platform networks, companies can address the issue of focusing on a single product life cycle and form a comprehensive network that promotes overall sustainable upgrades.
In summary, the DTs model can span the entire life cycle of various industries. For capital expenditure projects, DTs models provide a risk-free way to simulate construction, testing, logistics, and manufacturing sequences with the supply chain. It can also optimize the system design, so that project participants can clearly understand the ability to adapt and recover when extreme situations occur. For operating expenditure projects, the DTs model will truly become a 3D operating system for the control center, capable of tracking project progress in real time regarding the changes in time. In addition, the adoption of AI, IoT, and machine learning enables immersive digital operations in various industries. DTs models will facilitate analytical visibility and help designers generate deeper insights, which increase the productivity of modeling system operators, helping them predict and avoid problems and respond quickly.
3 Adoption Status of DTs Modeling
With the increasing popularity of IoT in smart city applications, DTs is constantly evolving as a complement to its physical counterpart. Lv et al. [38] pointed out that an intelligent industrial environment developed under a new generation network CPS can achieve a high concentration of information. DTs refer to the use of digital, networked, and sensor technologies to create a DTs body for physical equipment. The operating data and status of physical devices in the physical world can be fed back to the DTs body. The operation of physical equipment can be monitored, optimized, and predicted through DTs. The dynamic simulation function of the DTs system can simulate the feasibility, efficiency, and possible manufacturing problems of the design model in the manufacturing process, and feed it back to the design for modification. In this link, such as the design of car sludge models and DTs, 3D scanning can improve the conversion efficiency of physical and digital entities. DTs can be used in 3D IoT smart factories, new smart city construction, smart medical care, digital energy, digital archives, warehousing, and logistics visualization. It can provide digital data for business optimization in all walks of life to help understand, predict, and optimize performance, and improve business results. The specific application is shown in Fig. 8.
Figure 8: Adoption fields of DTs modeling
Fig. 8 shows that the DTs-based modeling method has gradually been applied in various industries. The DTs management system puts forward higher requirements for cognitive technology. To better build a full-time IoT cognitive management system in the industrial ecosystem and complete the multi-level development trend of physical target operations, the multi-directional accurate detection and cognitive technology not only require more accurate and reliable accurate measurement technology physically, but also need to take into account the collaborative interaction between cognitive data and information, so that the spatial location and unique identification of objects in the industrial ecosystem can be determined, ensuring the reliability and control of machinery and equipment. The creation of the DTs entity model is aimed at completing the role of the business process. For different model technologies, the most important competencies are dedicated tools and 3D model libraries. The component standard of the data-breeding 3D model library can provide the target component 3D model library with important content such as staff, machinery and equipment, raw materials, raw materials, and natural environment of the place. As the foundation and key of DTs, the basic theories, models, and related products of solid model construction and the development trend of the 3D model library are the key technologies of DTs, all of which are reasonable support points for key technologies of DTs.
3.1 DTs Modeling Contributes to Intelligent Manufacturing
DT is one of the most promising enabling technologies for intelligent manufacturing and Industry 4.0 [39–41]. DT is characterized by seamless integration between cyberspace and physical space. To understand the development and application of DTs in industry, Taos et al. [42] comprehensively reviewed the latest progress of DTs related research, including the key components of DTs, the current development of DTs, and the main application of DTs in industry. Rabah et al. [43] described part of the collaboration between industry and research fields to develop DTs and virtual reality industrial solutions as part of the Predictive maintenance framework. In this context, the proof-of-concept developed in specific industrial applications is carefully designed. With the progress of the new generation of information technology, especially BD and DTs technology, intelligent manufacturing is becoming the focus of global manufacturing transformation and upgrading. Comprehensive analysis of manufacturing BD is beneficial to all aspects of the manufacturing industry [44–46]. The application example is shown in Fig. 9.
Figure 9: Intelligent manufacturing data intelligent integration planning scheme
From Fig. 9, the manufacturing industry combined with BD can make the operation status of equipment and personnel in the factory clear as crystal, and provide security guarantee for hidden dangers that have not occurred. In addition, DTs pave the way for the physical convergence of the manufacturing industry, which is an important bottleneck to achieve intelligent manufacturing. Qi et al. [47] reviewed BD and DTs bodies in manufacturing, including their concepts and applications in product design, production planning, manufacturing, and predictive maintenance. On this basis, the similarities and differences between BD and DTs are compared from general and data perspectives. Since BD and DTs can be complementary, how they can be integrated together to facilitate intelligent manufacturing is discussed.
With the application of a new generation of information technology in industry and manufacturing, the era of manufacturing driven by BD is coming [48–50]. However, while BD is available across the entire product lifecycle, from product design to manufacturing to services, it is found that the current research on product life cycle data is mainly focused on physical products rather than virtual models. Tao et al. [51] proposed a new method of product design, manufacturing, and service driven by DTs. The detailed application method and framework of DTs driven product design, manufacturing, and service are analyzed. In addition, three cases are given to illustrate the future application of DTs body in three stages of the product. More than ever before, manufacturers today need to adapt to changing customer needs, rising resource costs, and increasing uncertainty [52–54]. One promising approach to these problems is the digitization of manufacturing systems. The key elements of digitization are network physical system and network physical production system. In the past few years, DTs have become synonymous with network physical systems and the network portion of network physical production systems. Kunath et al. [55] discussed the conceptual framework of DTs based on manufacturing system and the potential application of order management process decision support system.
Lohtander et al. [56] used micro manufacturing units, intended to apply it in the DTs research environment to explore how to build the DTs body from scratch and what information was needed to describe the realistic behavior of the digital model of the micromanufacturing units. DTs are based on actual machines and simulated digital models [57–59]. The basic model of the micro manufacturing unit DTs body will be built using production and part simulation software (FlexSim), allowing the machine to be integrated immediately into an industrial environment and to control all parameters of the production system later. Malik et al. [60] proposed a DTs framework to support the design, construction, and control of human-machine collaboration. In our work, computer simulations are used to develop digital equivalents of human-machine collaborative work environments for assembly work. Digital counterparts are kept up to date throughout the life cycle of the production system, enabling rapid and secure embedding by constantly mirroring the physical system for continuous improvement. The case of a manufacturing company with an HMI team to develop and validate the DTs framework is presented.
Intelligent manufacturing is regarded as the next generation of manufacturing mode, which is empowered by the new generation of information technologies such as the IoT, BD analysis, edge computing, and AI, with strong learning and cognitive abilities. The structural composition is shown in Fig. 10.
Figure 10: DTs provides a technical support framework for intelligent manufacturing
From Fig. 10, only with DTs can the factory truly realize intelligence and visualization. To further explore intelligent manufacturing and take autonomous manufacturing units as the realization scenario, Zhang et al. [61] proposed a data- and knowledge-driven DTs manufacturing unit framework to support autonomous manufacturing through intelligent perception, simulation, understanding, prediction, optimization, and control strategies. With the development of sensor and data processing technology, network physical system-based intelligent manufacturing is the development trend in the manufacturing industry. DTs has always been regarded as the implementation method of network physical system [62–64]. Given the complexity and uncertainty of discrete manufacturing plants, carbon emission data integration and automatic low-carbon control of manufacturing systems are two major challenges. To achieve carbon emission reduction in intelligent manufacturing workshops, Zhang et al. [65] proposed a DTs-driven carbon emission prediction and low-carbon control of intelligent manufacturing workshops. It includes the DTs model for low-carbon manufacturing shop, DTs data interaction and fusion for low-carbon manufacturing, as well as DTs-driven carbon emission prediction and low-carbon control.
With the rapid development of virtual technology and data acquisition technology, DTs technology has been proposed and gradually become one of the key research directions of intelligent manufacturing [66–68]. However, the research of DTs for product life cycle management is still in the theoretical stage, the application framework and application methods are not clear, and the lack of reference application cases is also a problem. Zheng et al. [69] systematically reviewed the related research and application of DTs technology. Then, they explained the concept and characteristics of DTs in broad and narrow sense. On this basis, an application framework of DT in product life cycle management was proposed. In the new digital era, all kinds of things and even the whole world can be digitized as models [70–72]. Simulations were conducted for a variety of purposes, and the results can provide intelligent support for various decisions. DT is an important form of modeling and simulation application in the new digital era. It has been widely used in intelligent manufacturing, factories, buildings, smart cities, and many other fields, showing strong vitality. The next step in its development direction is comprehensive digital engineering. Zhang et al. [73] reviewed the development history of DTs technology, analyzed its current situation and development prospects, and introduced the latest digital engineering strategy of the US Department of Defense. The opportunities and challenges of modeling and simulation in the new digital era were presented in the results.
The current lack of effective methods to develop products, processes, and operational models based on virtual and physical fusion in production has resulted in poor performance of production management in terms of intelligence, real-time capabilities, and predictability [74–76]. Bao et al. [77] proposed a method for modeling and operating DTs bodies in the manufacturing environment. Firstly, the concept and extension of DTs body in the manufacturing environment were described, and the realization methods of virtual physical fusion and information integration were provided for factories. Secondly, the modeling methods of product DTs, process DTs, and operation DTs were proposed, and the interoperation modes among these DTs bodies were explained. Third, AutomationML was used to model structural part processing units in order to specify how operations were performed among products, processes, and resources. DTs bodies, along with the IoT, data mining, and machine learning technologies, offer great potential for the transition from today’s manufacturing paradigm to intelligent manufacturing. Production control in the petrochemical industry involves complex environment and high demand for timeliness. Therefore, agility and intelligent control are important components of intelligent manufacturing in the petrochemical industry. Min et al. [78] proposed a framework and method for constructing DTs body based on IoT in the petrochemical industry, machine learning, and practice cycle of information exchange between physical factory and virtual DTs model, so as to realize production control optimization. Unlike traditional production control methods, this novel approach integrated machine learning and real-time industrial BD to train and optimize DTs models.
With the deepening of the application of information technology in the manufacturing industry, the informatization of manufacturing system has developed from unit digital manufacturing to integrated network manufacturing, and then to comprehensive digital, network, and intelligent manufacturing. Modeling and simulation technology, as a comprehensive information technology integrating computer, model theory, and scientific calculation, plays an irreplaceable role in the development of manufacturing informatization and is widely used in every stage of product life cycle, including design, production, testing, maintenance, procurement, and sales. Zhang et al. [79] reviewed the research and application of modeling and simulation technology in manufacturing industry, and analyzed typical simulation technology in manufacturing industry from the aspects of manufacturing unit simulation, manufacturing integration simulation, and manufacturing intelligence simulation. With significant advances in information technology, DTs are gaining attention because it provides a support tool to enable digitally-driven and cloud-enabled manufacturing. Given the nonlinear dynamics and uncertainties involved in mechanical degradation, the correct design and adaptability of DTs models remain a challenge. Wang et al. [80] proposed a DTs reference model for rotating machinery fault diagnosis. The requirements of DTs model construction were discussed, and a parameter sensitivity analysis-based model updating scheme was proposed to improve the adaptability of the model. Experimental data were collected from rotor systems simulating unbalance faults and their progression. The data was then fed into a DTs model of the rotor system to investigate its ability to quantify and locate unbalances for fault diagnosis. The results showed that the constructed DTs rotor model can realize an accurate diagnosis and adaptive degradation analysis.
The DTs system integrates data sources such as enterprise security monitoring, Global Positioning System (GPS), GIS, equipment, energy consumption, and assets by building a 3D virtual simulation scene. Simulating factory operating status and real-time changes in the digital world can provide effective means for enterprise collaborative management and production scheduling [81,82]. DTs are built on the product’s entire life cycle, so when the product is delivered to customers for use, the DTs system still plays a role in performance monitoring. The digitization of manufacturing has a history of nearly 60 years, accumulating many digital models. Based on these digital models, every device, every part, and every sensor in the factory of the future will have a digital replica. Through the DTs body, the operation mode of these physical devices can be precisely understood. Through the seamless matching of the digital model and the physical equipment, the data of the equipment monitoring system can be obtained in real time, then realizing fault prediction and maintenance. Industry 4.0 intelligent manufacturing system is composed of human-network-physical system, which integrates human, network, and physical system. DTs visualization architecture realizes human-computer interaction in manufacturing, which involves the modeling of DTs on both the physical side of the network and the human-network side. However, there is a lack of attention on full-lifecycle functional services and lightweight architectures.
The digitalization of manufacturing has greatly promoted the continuous improvement of productivity. Cutting tools powered by DTs give solutions to digital demands. Xie et al. [83] proposed a DTs-driven data flow structure for each state of the cutting tool’s life cycle to ensure the possibility of continuous process and tool improvement. Manufacturers have two service models to meet customer needs. In addition, the physical and virtuous tool wear data were jointed, providing guidance for the future progression of smart manufacturing. DTs have attracted increasing attention in the past few years. Modeling is the foundation to put DTs into practice. In this context, modeling of DTs workshops regarded as the basic units for intelligent manufacturing is explored. However, current exploration has rarely focused on the multi-scale features of workshops, which hinders the effective adoption of DTs workshops. In response to the problem of how to implement model building regarding time scale and spatial scale, Zhang et al. [84] proposed a multi-layer modeling method to support the transition from the unit layer to the system layer to the system layer model building. In addition, the mechanism by which the model changes over time was also discussed. Then, the model assembly, model fusion, and model update were analyzed. Finally, a satellite workshop was selected as a case to verify the model’s correctness and feasibility.
Production systems are imperative in smart manufacturing. Processing data is becoming a key technical component to enhance the intelligent manufacturing industry. Many manufacturing resources are developed using virtual resources associated with physical resources throughout their life cycle [85]. DTs workshop is the main component of intelligent manufacturing, and an example of the structure is shown in Fig. 11.
Figure 11: DTs workshop practical example
Fig. 11 shows that the DTs workshop, as the main body of twin manufacturing, directly determines the improvement of industrial production efficiency and energy consumption. With the emergence of IoT, BD, virtual reality, AI, and 5G, physical and virtual resource interconnection has become possible. DTs has potential to achieve cyber-physical production systems in Industry 4.0. Zhang et al. [86] introduced the vision of integrating physical resources into CPPS via DTs and AutomationML. To illustrate the adoption of ICT, the general architecture of DTs-based CPPS was discussed. Using the proposed information fusion, a case of expert fault diagnosis of aircraft engine was given. Secondly, an AutomationML-based CPPS information modeling method was proposed.
The concept of DTs, as an idea for new generation digital manufacturing research, is crucial in Industry 4.0. As the premise of digital manufacturing, the collection, modeling, and utilization of historical data of machining process and part inspection are of great significance to provide data support. However, due to the structural heterogeneity of the data, the modeling method of the part DTs body is not comprehensive, which hinders the real-time adjustment during the assembly. To this end, Bao et al. [87] proposed assembly-oriented modeling for part DTs. The model-based definition method obtains the design information of the part from the 3D model, and predefines and identifies the machining features. In addition, the assembly constraint relationship in the assembly unit in which parts participate is obtained, so as to complete the deviation transfer analysis, and filter the assembly key features as the carrier of processing and inspection data. Fan et al. [88] developed a component-oriented part DTs framework to show the major components and data flows used to create DTs principals with information filtering and subsequent management. The architecture of DTs visualization for flexible manufacturing was introduced, and it was illustrated how to explore the relationship between 3D visualized human-computer interaction and DTs scene information in the proposed architecture. In addition, visualization methods for high-value information related to life cycle planning, design, commissioning, and service phases were explored in depth.
One of the key enabling technologies of DTs is data modeling of physical products in order to closely integrate the physical world with cyberspace [89]. Therefore, it becomes critical to manage physical products as a modeling method for manufacturing data that faithfully reflects the physical state of the product. Dai et al. [90] addressed the problem of modeling formed parts during machining which hinders long-term development. Furthermore, an ontology-based data modeling method for manufacturing parts was proposed as a suggestion to create DTs for manufacturing parts. Thus, standardized process was given for creating DTs, classifications, what to model, and how to model manufactured parts by specifying information. Process planning is critical to realize intelligent product manufacturing. DTs are adopted to guide the process planning to realize the monitoring, simulation, prediction, and control of the physical space. Zhao et al. [91] introduced DTs Process Model (DTPM) for manufacturing. The real-time acquisition of data and the management of simulation data were discussed. Regarding the data fusion of physical and virtual space, a hierarchical model and mapping strategy of multi-source heterogeneous data were proposed to generate DTs data. Then, the visualization functions in DTPM process planning were analyzed. The effectiveness was verified by selecting the design process of the key components of the marine diesel engine.
As sharing economy becomes a mutually beneficial economic model, shared manufacturing is proposed supported by advanced information and manufacturing under the concept of sharing economy. Manufacturing resource allocation coordinates cross-organizational resources to provide on-demand services for individualized needs. However, several challenges still hinder the resource allocation. The traditional centralized optimization method has a limited decision-making model, and the autonomous decision-making power of resource providers is hard to maintain. Therefore, they are difficult to adapt to cross-organizational resource coordination. Furthermore, in the resource allocation, the credit of resource providers is rarely considered, which is not conducive to promoting reliable trade. Thus, Wang et al. [92] proposed an integrated architecture to facilitate resource allocation. DTs-driven service models were fabricated for seamless monitoring and shared manufacturing resource controlling. Numerical control machine tools are the mother machines of the industry. If the troubleshooting is not timely, numerical control machine failure can lead to loss of accuracy and affect production. Therefore, the reliability of numerical control machine tools is crucial. Predictive maintenance can avoid breakdowns and loss of life. Due to less concern on state diversity and consistency of numerical control machine tools, it is hard to harvest accurate, timely, and intelligent results. To achieve reliable predictive maintenance of numerical control, Luo et al. [93] investigated a DTs-driven hybrid approach. This approach was based on a hybrid approach driven by the DTs model and Siamese data. Then, a DTs-based hybrid predictive maintenance model was developed.
For physical devices and network models, DTs can implement hardware-in-the-loop simulation. Manufacturing system reconfiguration cost can be reduced if design flaws are discovered during the deployment of traditional irreversible design methods [94,95]. Liu et al. [96] proposed a DTs-based four-fold function for the design of process-based intelligent manufacturing systems in Industry 4.0, namely configuration design-motion planning-control development-optimization decoupling. The accuracy of workshop scheduling is remarkably affected by uncertain factors. DTs combine planning and scheduling. The concept of DTs is effectively used to comprehensively control the uncertain factors in production activities, so that the actual production can be accurately guided. Wang et al. [97] first established the management and control of the DTs-based planning and scheduling system. Finally, the key technologies of the planning process were expounded. To verify the effectiveness, in combination with the frame workshop design, a planning and scheduling system suitable for enterprises was developed. The rapid development of new-generation information technologies such as BD analysis, IoT, edge computing, and AI has driven manufacturing from traditional to intelligent manufacturing with autonomy and self-optimization, and new requirements such as learning and cognitive ability are put forward for the manufacturing unit, which is called the minimum realization unit of intelligent manufacturing. Therefore, Zhou et al. [98] proposed a framework for knowledge-driven DTs intelligent manufacturing cells. The framework can support autonomous manufacturing through intellisense, simulation, prediction, optimization, and control strategies. In addition, DTs model, dynamic knowledge base, and knowledge-based intelligent skills that support the above strategies are adopted. Thus, the framework is capable of self-thinking, self-decision, self-execution, and self-improvement.
To achieve defect-free production, Gaikwad et al. [99] combined predictions from physical models with in-situ sensor features and meanwhile detect the additive defects in manufactured parts. The instantaneous spatiotemporal distribution of temperature in thin-walled titanium alloy parts was predicted using a graph theory-based computational heat transfer model. Subsequently, the above physically derived thermal trends were combined with in-situ temperature measurements obtained from pyrometers in a supervised machine learning framework (SVM). DTs provide feasible implementation paths for the realization of smart manufacturing and CPSs. DTs are applied in the design, production, and service. Workshop DTs is digitally mapped models of the corresponding physical workshop. Applying digital micro-distortion at the production stage is a challenge. In response to the existing problems, Zhuang et al. [100] first reviewed the evolution of DTs, such as the adoption status in the production stage. Then, an implementation framework for the construction of workshop DTs was proposed. The new generation of machines is supposed to be digital, efficient, networked, and intelligent. Intelligent machine tools powered by DTs provide excellent solutions for intelligent manufacturing. Tong et al. [101] proposed an intelligent machine tool DTs-based real-time machining data service. Multi-sensor fusion was adopted for real-time data acquisition. Data transfer and storage were achieved via MTConnect protocol. Various applications were developed for data visualization in the body of DTs, including processing trajectories, processing status, and energy consumption.
In smart manufacturing, several challenges have arisen in production management, including the utilization of information and the elimination of dynamic disturbances. The DTs-Driven Production Management System (DTPMS) can dynamically simulate and optimize the production, and realize real-time synchronization, high fidelity, and real-virtual integration in cyber-physical production. Ma et al. [102] focused on how to build a DTPMS for production lifecycle management. First, it was demonstrated how to integrate DTs and simulation platforms. Secondly, the DTPMS framework can support the CPS of the production workshop. Finally, DTPMS was utilized in the production of heavy-duty vehicle gearboxes. The processing plan as the guidance can ensure the quality of product processing. Existing methods are inefficient and cannot quickly handle machining plan changes caused by unpredictable production events in a real-time manner, inevitably causing time and financial loss to the business. To demonstrate the evolution characteristics of product processing, Liu et al. [103] proposed a method for constructing a DTs process model regarding the knowledge evolution processing characteristics. Three key technologies, including the related structure of process knowledge, the expression of evolutionary geometric features, and the correlation mechanism between them, were solved. Then, DTs process model construction was explained. The organization and management of multi-source heterogeneous data were analyzed. Finally, a case study of complex machined parts showed that machining time was reduced by 7% and machining stability was improved by 40%. Costantini et al. [104] found that the EU-funded IoTwins project aims to build a solid platform that manufacturers can use to develop hybrid DTs of their assets, deploying them as close as possible to data sources. Location (on IoT gateways or edge nodes) and leverage cloud-based resources to offload computationally intensive tasks such as big data analysis and ML model training. The authors introduced the main research goals of the IoTwins project and discussed its reference architecture, platform capabilities, and building components. Finally, they used case to prove how manufacturers can exploit the potential of the IoTwins platform to develop and execute distributed DTs for the purpose of predictive maintenance.
To sum up, in the wave of intelligent manufacturing, traditional 2D scene application can no longer meet the information requirements of multi-equipment, multi-process process, and process simulation. The specific research results are summarized in Table 1.
Real-time data of factory operation can be received through 3D DTs model. In addition to presenting real and real-time data information, it can conduct real-time comparison and monitoring of equipment operation process, find abnormal situations in advance, and automatically execute relevant plans to realize early warning and predictable maintenance, thus avoiding accidents and ensuring continuity and stability of production process. It can also reflect the real operation logic through machine learning and other technologies to realize data analysis and intelligent decision analysis in various production scenarios. To realize intelligent manufacturing industry, it is necessary to realize the interconnection of all elements, the whole industrial chain, and the whole value chain. The consistency of information flow and physical flow can be realized through state perception and data fusion, and dynamic scheduling and optimization of factories can be realized by means of industrial intelligence and autonomous control, thereby realizing collaborative lean management between multiple workshops and organizations.
3.2 DTs Modeling in Smart City Construction
Insight-driven real-time traffic management is becoming an important part of building and sustaining smarter cities globally. Many automated traffic management solutions have been proposed by information technology solutions and services organizations, and their main problem is that they are passive and therefore, an inefficient solution for an increasingly connected and dynamic urban environment [105,106]. Digitisation makes infrastructure and cities smarter. The use of physical space and energy, the transfer of information, the management of users, assets and processes, and the operation of businesses and companies have been progressively digitized. The main challenges of smart cities are their definition, scope, and interconnectedness. There are different approaches to smart city implementation, from collaborative multidisciplinary environments, adding information and communication technology to their physical structures, to higher abstract decisions using BD. Serrano [107] proposed the concept of digitalization as a service, in which any complete digitalization can be realized independently of the relevant physical infrastructure in the cloud environment. Digitisation as a service will enable interoperable virtual digital infrastructure. In addition, they reviewed current digital systems, transmission networks, servers, and management systems.
With the emergence of a new generation of information technology in industry and product design, the era of product design driven by BD has come [108]. Fig. 12 shows the example of smart park constructed by combining industrial park and DTs.
Figure 12: Smart park DTs application platform
As can be seen from Fig. 12, during the initial construction of the smart park, various new technologies, such as cloud computing, IoT, big data, and AI, are surging, each acting independently. These technologies applied in various fields have no unified standard for the information system constructed by them and are incompatible with each other. Besides, the access is blocked, leading to the isolated island of information. With the help of DTs technology, every link of the smart park is very transparent. From government decision-making and services, to people’s lifestyles, to the industrial layout and planning of the city, to the operation and management of the city, DTs technology can accelerate the smart development process of the industry and even the city. Thus, DTs play a critical role in the management of smart parks. However, BD-driven product design mainly emphasizes the analysis of physical data rather than virtual models, in other words, the fusion between the physical and virtual space of the product usually does not exist. DTs is a new and rapidly evolving technology that connects the physical and virtual worlds, and has recently attracted a lot of attention around the world. Tao et al. [109] proposed a new DTs-based product design method. Smart cities aim to solve their inherent problems while reducing costs and improving quality of life. Through the fourth industrial revolution technology, the advantages of smart cities are estimated to overcome the cost of cities through urban platformization. Although cities have traditionally been the subject of creation rather than consumption, smart cities are now key industries that create value accounting for more than 60% of their GNP from the perspective of production [110–112]. Zhao et al. [113] proposed a context-aware autonomous control method for micro-point press by establishing a DTs-driven network physical system. The key enabling technology of network space and physical device pairing was discussed, and a high-precision on-line detection and control system-based dynamic adjustment model of micro-point punching piezoelectric ceramics was proposed.
DTs in big cities are changing from static to dynamic, from static data to dynamic, and from current analysis to predictive analysis. Its logic is the same as the development trend of AI technology. That is, Internet BD can identify the present, and AI can predict the future. Similarly, if AI technology is “bred” by data information, the key to large DTs cities is also data information. Under the current general trend of digital transformation, the DTs building, which is an effective integration of DTs and the construction industry, has become the core engine for the upgrading of the construction industry, and is also the necessary foundation for the construction of DTs cities. DTs building employs BIM and cloud computing, BD, IoT, AI, virtual simulation, and other DTs body enabling technologies. Combining advanced lean construction project management theories and methods, a business development strategy driven by DTs is formed. It integrates various aspects and manages life cycle of buildings. It realizes the whole-process, all-factor, all-participation, and people-oriented development of living environment and the intelligent adoption of better life experience. In this way, a new ecosystem of enterprise, industry, and DTs urban applications will be established. An example of the DTs building ecosystem platform is shown in Fig. 13.
Figure 13: Example of DTs building ecosystem platform
With the continuous progress and mature adoption of new technologies of DTs and additive manufacturing, it empowers the digital transformation of the construction industry. Utilizing DTs virtual buildings and energy modeling technology can transform the design, management, and maintenance of buildings. For example, real-time energy consumption data of physical buildings can be collected through the adoption of various sensors and intelligent sensing technologies. With reference to building design, HVAC, lighting, power supply, and weather data, building owners are provided with the best solutions for the various equipment usage through calculation, thus reducing energy consumption. At present, the operation mode in building operation in China is still carried out by combining traditional manpower with some building intelligent subsystems. This operation and maintenance method is relatively timely to deal with the faults of construction equipment, but the ability of early warning and analysis of construction equipment faults is still inadequate. Ideal building operation and maintenance service should be “prevention first, maintenance second”. Therefore, DTs provide efficient technical support for intelligent building operation and maintenance and predictive maintenance of building facilities through its comprehensive analysis and prediction capabilities. Digital architecture will have an unignorable impact on the industry as core engine for the transformation and upgrading of it. Nevertheless, the promotion of digital architecture is by no means something that one company can accomplish. Construction industry can be digitized, online, and intelligent on digital building platform, eventually realizing the form of fully intelligent future buildings.
The construction industry faces many challenges including low productivity and poor technological progress. Advances in DTs have been exploited tremendously in digitally advanced industries. DTs have the potential to transform the construction industry and solve challenges, so they have attracted a lot of attention and are growing rapidly. To analyze the DTs applications in the construction industry, Opoku et al. [114] adopted systematic review combined with scientific mapping methods to comprehensively review and analyze DTs concepts, techniques, and applications in the construction industry. After a comprehensive search and careful selection of multiple databases according to the proposed criteria, 22 academic publications on the adoption of DTs were identified and categorized. Angjeliu et al. [115] investigated the structural system integrity of historic masonry buildings, aiming to develop a program that creates a model that integrates experimental physical reality to analyze the structural response of the system. The key elements of the inspection were the construction of an accurate 3D model, the selection of materials, and the construction stages. Relationships to structural investigations, field observations, and measurements were explored. An ideal approximation of the real geometry was achieved, with efficient discretization in the finite element model.
High-precision Products (HPP) with multi-disciplinary coupling are applied in aerospace, marine, and chemical fields. Due to the complex and compact structure of HPP, the assembly process requires high precision. The traditional assembly is of low assembly efficiency and poor quality-consistency. In response to these deficiencies, Sun et al. [116] proposed a DTs-driven assembly and debugging method for HPP. First, the theoretical framework of DTs-driven assembly and debugging was introduced, whose effectiveness and feasibility were verified. Virtual DTs bodies can be employed to optimize the assembly process online without stopping the assembly process involved in the production line [117–119]. Al-Ali et al. [120] proposed an end-to-end conceptual model of DTs representing their complementary physical objects. The multi-layered nature of the proposed DTs model is also introduced. The hardware and software techniques utilized to construct such models were explained.
In the future, based on security and building DTs, the integration of fire emergency data and information resources can realize the unified management and control of urban firefighting capabilities and information, thereby creating an intelligent urban firefighting emergency command system. It can assist on-site staff in choosing the best rescue route and fire rescue work, and improve disaster prevention, disposal, and emergency rescue capabilities. At the same time, it can also formulate a DTs-based fire drill plan, conduct business training for firefighters, and improve the emergency response capabilities of firefighters. When an emergency occurs during the operation of a real physical city and crowd evacuation and emergency rescue are required, relevant simulation software can be used to quickly conduct simulation analysis in combination with building DTs to find the optimal crowd evacuation path and emergency rescue plan. For example, in the prevention and control of the new crown epidemic, the community DTs building model can be used to realize the combination of health information and home isolation status of residents in community buildings and realize dynamic alarm and normal management. In this way, it can provide important technical and data resource guarantees for community-level anti-epidemic staff to reduce stress and community network-based smart management.
DTs city is expected to reflect and influence city functions and processes to enhance their realization, operability, and management. Advances in DTs are growing rapidly and make viable contributions to the progression of smart cities. The benefits of DTs cities are assessed regarding the real-time data from pre-installed IoT sensors such as traffic, energy usage, air pollution, and water quality, used to manage complex urban systems. However, sensor-based real-world information may not be sufficient to provide dynamic spatiotemporal information about physical vulnerabilities. An example of the implementation path for DTs cities is shown in Fig. 14.
Figure 14: Example of DTs urban modeling implementation path
From Fig. 14, understanding the current state of physical vulnerability in cities can support urban decision makers in analyzing the potential risks associated with urban areas, enabling data-driven infrastructure management during extreme weather events. Shahat et al. [121] proposed to guide future research on urban DTs with the aim of reaching the highest level of comprehensive and complete urban DTs body. Data processing, inclusion of urban socio-economic components, and mutual integration between the two counterparts of DTs are the future research directions for realizing and utilizing fully mirrored urban DTs. Advances in remote sensing technology have resulted in inexpensive city-scale light detection and ranging point clouds that can be used to model urban objects to create DTs cities. Xue et al. [122] proposed a new unsupervised method called object symmetric cross-section clustering, to process city-scale light detection and ranging point clouds as object hierarchies according to their characteristic cross-sections.
In an era of rapid urban growth and expansion, planning is critical for cities to improve their competitiveness while supporting the well-being of their citizens, and this is where DTs comes in handy [123–125]. As a step towards creating DTs cities for effective risk-informed decision-making, Ham et al. [126] proposed a new framework to bring the reality information of visual data into 3D virtual cities for model updating with interactive and immersive visualization. Unstructured visual data was collected and analyzed from participatory sensing to estimate geospatial information on distant vulnerable objects representing the physical vulnerability of cities. DTs bodies were originally designed to improve manufacturing processes using simulations with high-accuracy individual component models. However, it is now possible to create DTs smart cities. 3D model of a city can be posted online, and this open and public model allows for additional virtual feedback loops where citizens can interact and report feedback on changes to the city’s plan. White et al. [127] presented a body of public and open DTs in Dublin Docklands, Ireland. The model was also demonstrated for the planning of urban skylines and green Spaces, allowing users to interact and report feedback on plan changes.
Various research has focused on ICT and AI solutions to enhance disaster management, fragmented without a common vision of a convergent paradigm. Fan et al. [128] proposed a vision for the DTs model of disaster cities. This paradigm enables an interdisciplinary fusion of crisis informatics and ICT in disaster management, enabling the integration of AI algorithms, to improve assessment, decision-making, and coordination. Moreover, it can increase visibility into the cyber dynamics of complex disaster management and humanitarian operations. Disaster city DTs are used as a unified paradigm. The mentioned DTs include multi-data sensing for data collection, data integration and analysis, multi-player game-theoretic decision making, and dynamic network analysis [129]. From an architectural and engineering/construction perspective, activities related to avoiding double taxation are still in their early stages regarding buildings and other infrastructure assets. Less attention is paid to the operations and maintenance phases, which are the longest time spans in an asset’s life cycle. A systematic and clear architecture, validated by building practical use cases for DTs, will be the most important step in the efficient operation and maintenance of buildings and cities. Regarding the current research on multi-layer architectures, Lu et al. [130] proposed a system architecture for DTs specifically designed for building and urban levels.
COVID-19 has sparked a crisis affecting all populations, and a response plan is considered an effective way to control the spread of the virus. Research suggested that urban DTs can be beneficial in addressing this crisis because it can build a virtual replica to simulate climate conditions, response policies, and people’s trajectories to help plan efficient and inclusive decision-making. However, urban DTs systems decide things relying on long-term data collection, limiting their advantages in the face of emergency crises. Pang et al. [131] proposed a framework that fuses urban DTs with federated learning for a novel collaborative paradigm that allows data sharing among multiple urban DTs. DT is a key enabler for the implementation of Industry 4.0 in smart cities, which connects disparate objects by leveraging advanced IoT. However, DTs should provide more than just numerical representations.
Two-way data exchange and real-time self-management distinguish DTs from other information modeling. Digital transportation is increasingly required as it becomes a core technology in many industrial sectors post-COVID-19. Sepasgozar [132] clarified the concept of DTs and differentiated them from other 3D modeling techniques and digital information systems, and examined the current state of DTs and provided directions for future investigations. Architecture based on DTs modeling can provide data such as building height, roof surface, and insolation, which allows city planners to analyze which buildings have high solar energy production potential and are suitable for installing building energy storage materials such as solar panels. Further analysis allows city smart grid managers to estimate how much solar energy each community generates in a day, as well as the savings in electrical energy and costs. At the same time, the correctness of the above analysis can also be verified by cross-referencing with the historical data of adjacent buildings. Seasonal adjustments can be implemented to reflect more accurate and refined forecasts of community electricity transmission and distribution needs. In addition, the usage data of the lighting system and HVAC system in each building can be obtained in real time by adopting the intelligent sensor system, to accurately understand the specific power consumption and peak data of buildings and urban communities, thereby providing the basis for the implementation of intelligent transmission and distribution of urban power grids.
Most research about DTs focuses only on the manufacture and proposed explicit frameworks and architectures that face the challenge of supporting different levels of integration through agile processes [133–135]. Furthermore, no empirical work focused on exploring the relationship between DTs and large-scale personalization. Aheleroff et al. [136] developed a reference architecture for DTs and applied it in industrial cases. DT is of smart planned maintenance, real-time monitoring, remote control, and predictive capabilities [137–139]. The results showed a significant relationship between the ability of DTs as a service and large-scale personalization.
To sum up, according to the various adoption scenarios of DTs cities, there are many smart applications under DTs buildings, such as smart community management, smart hospital management, and smart parking management. In smart cities, DTs buildings can transform traditional buildings from inanimate reinforced concrete structures into self-adaptive and evolvable “living bodies” with comprehensive perception and AI through swarm intelligence and DTs. Thus, an open smart building ecosystem with deep integration of human, machine, and material can be formed to provide efficient technical support for smart city construction. The future DTs building should be an intelligent building that learns and thinks, which can communicate and interact with people naturally, and can adapt to various scenarios. As part of a DTs city, it can be highly interconnected at a high structural level.
4 DTs Modeling Research Limitations and Future Prospects
Overall, DTs are still in the early stage. DTs need to conduct global perception, execute monitoring, integrate historical accumulated data for calculation, and output information quickly and timely. The first is a high reliance on the data and information collected by sensors. In terms of data perception, accurate global perception of machines in factories is still difficult at the current level of technology, let alone other fields. Physical entity data is not detailed, leading to missing digital copy and errors in the predictions and judgments made by the digital copy. To solve which, chips, sensors, and IoT are required. As for software, algorithms and the integration of various software are required. The current bottleneck of DTs comes from various aspects. Nevertheless, it can bring infinite possibilities for industrial manufacturing and future life.
According to the applications of DTs modeling in various industries mentioned above, the business value of DTs is increasing. However, it relies on mature technical capabilities such as BD, IoT, cloud computing, AI, and game technology. Comprehensive technology providers that can provide these technologies in a package can therefore provide better products, better iterative capabilities, and natural competitive advantages. Then, in the future, maybe every commodity and everyone can have their own DTs body, which will completely change our future production and living conditions. The development prospect of DTs is bright. DTs can help companies improve customer experience and even help drive new business innovation by understanding customer needs and improving existing products, operations, and services. DTs has been widely used in various scenarios, mainly in design and monitoring scenarios. Smart cities, Industry 4.0, and the intelligent driving industry are all industries that apply advanced DTs, and are also the focus of the layout of many technology giants. DTs are widely adopted in an increasing number of enterprises, especially those that move from product sales to bundled sales of products and services, or those that sell as services. As enterprise capabilities and maturity increase, more enterprises will adopt DTs to optimize processes, make data-driven decisions, and revise new products, services, and business models in the future. To play the full potential of DTs in a long term, data must be integrated with the entire ecosystem.
As the development trend of DTs has accelerated in recent years, more and more companies have begun to adopt DTs to optimize processes and make database decisions in real-time, becoming a pioneer in the adoption of DTs in capital-intensive industries such as manufacturing, utilities, and energy [140–142]. If early practitioners showed a first-mover advantage in many industry sectors, others followed. DTs’ full-potential adoption may require integrating systems and data across the ecosystem. A complete customer lifecycle or supply chain digital simulation will be established, which provides an insightful macro view of operations. However, integrating external entities into the internal digital ecosystem is also needed. Today, companies are still unpleased with external integration beyond point-to-point connections. Enterprises hope to use blockchain to break down silos of information, validate information, and enter DTs, which will free up previously inaccessible data, making simulations detailed and dynamic, and generating greater potential.
From real 3D modeling to DTs modeling, only high technology is not enough to achieve nationwide widespread popularization and standardized application. More targeted and multi-disciplinary integration of intelligence, automation, and especially low-cost technology is needed. However, obtaining data is not the purpose. To realize the value of data, it needs the efficient use of real-life 3D model data with multiple levels of detail and real-time sensing data of the IoT sensor network. Data-model-knowledge integration processing technology is required to form a general intelligent system, which integrates many mechanism models with people’s knowledge and experience. The technical realization of DTs relies on integration of novel technologies and interdisciplinary knowledge. The key methods involved are modeling, BD analysis, machine learning, and simulation. At present, a series of problems such as different statistical calibers of data in various departments within the enterprise, low self-collection efficiency, and low real-time performance of data are common, affecting the accuracy of enterprise DTs characterization. In the future, through the introduction of emerging machine learning technologies, deep feature extraction of multi-dimensional heterogeneous data can be realized, which will greatly improve the efficiency of data analysis and make it gradually possible to build complex DTs for enterprises.
Funding Statement: The authors received no specific funding for this study.
Conflicts of Interest: The authors declare they have no conflicts of interest to report regarding the present study.
References
1. Qi, Q., Tao, F., Hu, T., Anwer, N., Liu, A. et al. (2021). Enabling technologies and tools for digital twin. Journal of Manufacturing Systems, 58, 3–21. DOI 10.1016/j.jmsy.2019.10.001. [Google Scholar] [CrossRef]
2. Redelinghuys, A. J. H., Basson, A. H., Kruger, K. (2020). A six-layer architecture for the digital twin: A manufacturing case study implementation. Journal of Intelligent Manufacturing, 31(6), 1383–1402. DOI 10.1007/s10845-019-01516-6. [Google Scholar] [CrossRef]
3. Leng, J., Liu, Q., Ye, S., Jing, J., Wang, Y. et al. (2020). Digital twin-driven rapid reconfiguration of the automated manufacturing system via an open architecture model. Robotics and Computer-Integrated Manufacturing, 63, 101895. DOI 10.1016/j.rcim.2019.101895. [Google Scholar] [CrossRef]
4. Bhatti, G., Mohan, H., Singh, R. R. (2021). Towards the future of smart electric vehicles: Digital twin technology. Renewable and Sustainable Energy Reviews, 141, 110801. DOI 10.1016/j.rser.2021.110801. [Google Scholar] [CrossRef]
5. Wang, Y., Wang, S., Yang, B., Zhu, L., Liu, F. (2020). Big data driven hierarchical digital twin predictive remanufacturing paradigm: Architecture, control mechanism, application scenario and benefits. Journal of Cleaner Production, 248, 119299. DOI 10.1016/j.jclepro.2019.119299. [Google Scholar] [CrossRef]
6. Fujii, T. Y., Hayashi, V. T., Arakaki, R., Ruggiero, W. V., Bulla Jr, R. et al. (2021). A digital twin architecture model applied with MLOps techniques to improve short-term energy consumption prediction. Machines, 10(1), 23. DOI 10.3390/machines10010023. [Google Scholar] [CrossRef]
7. Tu, Z., Qiao, L., Nowak, R., Lv, H., Lv, Z. (2022). Digital twins-based automated pilot for energy-efficiency assessment of intelligent transportation infrastructure. IEEE Transactions on Intelligent Transportation Systems, 1–11. DOI 10.1109/TITS.2022.3166585. [Google Scholar] [CrossRef]
8. Zhang, J., Deng, T., Jiang, H., Chen, H., Qin, S. et al. (2021). Bi-level dynamic scheduling architecture based on service unit digital twin agents. Journal of Manufacturing Systems, 60, 59–79. DOI 10.1016/j.jmsy.2021.05.007. [Google Scholar] [CrossRef]
9. Pan, Y. H., Qu, T., Wu, N. Q., Khalgui, M., Huang, G. Q. (2021). Digital twin based real-time production logistics synchronization system in a multi-level computing architecture. Journal of Manufacturing Systems, 58, 246–260. DOI 10.1016/j.jmsy.2020.10.015. [Google Scholar] [CrossRef]
10. Dang, H. V., Tatipamula, M., Nguyen, H. X. (2021). Cloud-based digital twinning for structural health monitoring using deep learning. IEEE Transactions on Industrial Informatics, 18(6), 3820–3830. DOI 10.1109/TII.2021.3115119. [Google Scholar] [CrossRef]
11. Fahim, M., Sharma, V., Cao, T. V., Canberk, B., Duong, T. Q. (2022). Machine learning-based digital twin for predictive modeling in wind turbines. IEEE Access, 10, 14184–14194. DOI 10.1109/ACCESS.2022.3147602. [Google Scholar] [CrossRef]
12. Keskin, B., Salman, B., Koseoglu, O. (2022). Architecting a BIM-based digital twin platform for airport asset management: A model-based system engineering with SysML approach. Journal of Construction Engineering and Management, 148(5), 04022020. DOI 10.1061/(ASCE)CO.1943-7862.0002271. [Google Scholar] [CrossRef]
13. Liu, Z., Chi, Z., Osmani, M., Demian, P. (2021). Blockchain and building information management (BIM) for sustainable building development within the context of smart cities. Sustainability, 13(4), 2090. DOI 10.3390/su13042090. [Google Scholar] [CrossRef]
14. Ozturk, G. B. (2020). Interoperability in building information modeling for AECO/FM industry. Automation in Construction, 113, 103122. DOI 10.1016/j.autcon.2020.103122. [Google Scholar] [CrossRef]
15. Darko, A., Chan, A. P., Yang, Y., Tetteh, M. O. (2020). Building information modeling (BIM)-based modular integrated construction risk management–Critical survey and future needs. Computers in Industry, 123, 103327. DOI 10.1016/j.compind.2020.103327. [Google Scholar] [CrossRef]
16. Jiang, Z., Lv, H., Li, Y., Guo, Y. (2021). A novel application architecture of digital twin in smart grid. Journal of Ambient Intelligence and Humanized Computing, 1–17. [Google Scholar]
17. Lopez, J., Rubio, J. E., Alcaraz, C. (2021). Digital twins for intelligent authorization in the B5G-enabled smart grid. IEEE Wireless Communications, 28(2), 48–55. DOI 10.1109/MWC.001.2000336. [Google Scholar] [CrossRef]
18. Lim, K. Y. H., Zheng, P., Chen, C. H., Huang, L. (2020). A digital twin-enhanced system for engineering product family design and optimization. Journal of Manufacturing Systems, 57, 82–93. DOI 10.1016/j.jmsy.2020.08.011. [Google Scholar] [CrossRef]
19. Mostafa, F., Tao, L., Yu, W. (2021). An effective architecture of digital twin system to support human decision making and AI-driven autonomy. Concurrency and Computation: Practice and Experience, 33(19), e6111. DOI 10.1002/cpe.6111. [Google Scholar] [CrossRef]
20. Bauer, P., Stevens, B., Hazeleger, W. (2021). A digital twin of earth for the green transition. Nature Climate Change, 11(2), 80–83. DOI 10.1038/s41558-021-00986-y. [Google Scholar] [CrossRef]
21. Kovalyov, S. P. (2020). Information architecture of the power system digital twin. Sistemy i Sredstva Informatiki Systems and Means of Informatics, 30(1), 66–81. [Google Scholar]
22. Yuan, Z., Li, Y., Tang, S., Li, M., Guo, R. et al. (2021). A survey on indoor 3D modeling and applications via RGB-D devices. Frontiers of Information Technology & Electronic Engineering, 22(6), 815–826. DOI 10.1631/FITEE.2000097. [Google Scholar] [CrossRef]
23. Ding, L., Jiang, W., Zhou, Y., Zhou, C., Liu, S. (2020). BIM-Based task-level planning for robotic brick assembly through image-based 3D modeling. Advanced Engineering Informatics, 43, 100993. DOI 10.1016/j.aei.2019.100993. [Google Scholar] [CrossRef]
24. Pan, D., Xu, Z., Lu, X., Zhou, L., Li, H. (2020). 3D scene and geological modeling using integrated multi-source spatial data: Methodology, challenges, and suggestions. Tunnelling and Underground Space Technology, 100, 103393. DOI 10.1016/j.tust.2020.103393. [Google Scholar] [CrossRef]
25. Gan, B., Zhang, C., Chen, Y., Chen, Y. C. (2021). Research on role modeling and behavior control of virtual reality animation interactive system in internet of things. Journal of Real-Time Image Processing, 18(4), 1069–1083. DOI 10.1007/s11554-020-01046-y. [Google Scholar] [CrossRef]
26. Yu, L., Fu, X., Xu, H., Fei, S. (2020). High-precision camera pose estimation and optimization in a large-scene 3D reconstruction system. Measurement Science and Technology, 31(8), 085401. DOI 10.1088/1361-6501/ab816c. [Google Scholar] [CrossRef]
27. Major, P., Li, G., Hildre, H. P., Zhang, H. (2021). The use of a data-driven digital twin of a smart city: A case study of Ålesund, Norway. IEEE Instrumentation & Measurement Magazine, 24(7), 39–49. DOI 10.1109/MIM.2021.9549127. [Google Scholar] [CrossRef]
28. Li, C., Yu, L., Fei, S. (2020). Large-scale, real-time 3D scene reconstruction using visual and IMU sensors. IEEE Sensors Journal, 20(10), 5597–5605. DOI 10.1109/JSEN.7361. [Google Scholar] [CrossRef]
29. Lv, Z., Qiao, L. (2020). Deep belief network and linear perceptron based cognitive computing for collaborative robots. Applied Soft Computing, 92, 106300. DOI 10.1016/j.asoc.2020.106300. [Google Scholar] [CrossRef]
30. Rasheed, A., San, O., Kvamsdal, T. (2020). Digital twin: Values, challenges and enablers from a modeling perspective. IEEE Access, 8, 21980–22012. DOI 10.1109/Access.6287639. [Google Scholar] [CrossRef]
31. Liu, S., Bao, J., Lu, Y., Li, J., Lu, S. et al. (2021). Digital twin modeling method based on biomimicry for machining aerospace components. Journal of Manufacturing Systems, 58, 180–195. DOI 10.1016/j.jmsy.2020.04.014. [Google Scholar] [CrossRef]
32. Shangguan, D., Chen, L., Su, C., Ding, J., Liu, C. (2022). A triple human-digital twin architecture for cyber-physical systems. Computer Modeling in Engineering & Sciences, 131(3), 1557–1578. DOI 10.32604/cmes.2022.018979. [Google Scholar] [CrossRef]
33. Liu, C., Jiang, P., Jiang, W. (2020). Web-based digital twin modeling and remote control of cyber-physical production systems. Robotics and Computer-Integrated Manufacturing, 64, 101956. DOI 10.1016/j.rcim.2020.101956. [Google Scholar] [CrossRef]
34. Wu, C., Zhou, Y., Pessôa, M. V. P., Peng, Q., Tan, R. (2021). Conceptual digital twin modeling based on an integrated five-dimensional framework and TRIZ function model. Journal of Manufacturing Systems, 58, 79–93. DOI 10.1016/j.jmsy.2020.07.006. [Google Scholar] [CrossRef]
35. Schroeder, G. N., Steinmetz, C., Rodrigues, R. N., Henriques, R. V. B., Rettberg, A. et al. (2020). A methodology for digital twin modeling and deployment for Industry 4.0. Proceedings of the IEEE, 109(4), 556–567. DOI 10.1109/JPROC.2020.3032444. [Google Scholar] [CrossRef]
36. Wang, H., Li, H., Wen, X., Luo, G. (2021). Unified modeling for digital twin of a knowledge-based system design. Robotics and Computer-Integrated Manufacturing, 68, 102074. DOI 10.1016/j.rcim.2020.102074. [Google Scholar] [CrossRef]
37. Li, X., Cao, J., Liu, Z., Luo, X. (2020). Sustainable business model based on digital twin platform network: The inspiration from haier’s case study in China. Sustainability, 12(3), 936. DOI 10.3390/su12030936. [Google Scholar] [CrossRef]
38. Lv, Z., Han, Y., Singh, A. K., Manogaran, G., Lv, H. (2020). Trustworthiness in industrial IoT systems based on artificial intelligence. IEEE Transactions on Industrial Informatics, 17(2), 1496–1504. DOI 10.1109/TII.9424. [Google Scholar] [CrossRef]
39. Ali, M. I., Patel, P., Breslin, J. G., Harik, R., Sheth, A. (2021). Cognitive digital twins for smart manufacturing. IEEE Intelligent Systems, 36(2), 96–100. DOI 10.1109/MIS.2021.3062437. [Google Scholar] [CrossRef]
40. Zhuang, C., Liu, J., Xiong, H. (2018). Digital twin-based smart production management and control framework for the complex product assembly shop-floor. The International Journal of Advanced Manufacturing Technology, 96(1), 1149–1163. DOI 10.1007/s00170-018-1617-6. [Google Scholar] [CrossRef]
41. Ante, L. (2021). Digital twin technology for smart manufacturing and Industry 4.0: A bibliometric analysis of the intellectual structure of the research discourse. Manufacturing Letters, 27, 96–102. DOI 10.1016/j.mfglet.2021.01.003. [Google Scholar] [CrossRef]
42. Tao, F., Zhang, H., Liu, A., Nee, A. Y. (2018). Digital twin in industry: State-of-the-art. IEEE Transactions on Industrial Informatics, 15(4), 2405–2415. DOI 10.1109/TII.9424. [Google Scholar] [CrossRef]
43. Rabah, S., Assila, A., Khouri, E., Maier, F., Ababsa, F. et al. (2018). Towards improving the future of manufacturing through digital twin and augmented reality technologies. Procedia Manufacturing, 17, 460–467. DOI 10.1016/j.promfg.2018.10.070. [Google Scholar] [CrossRef]
44. Liu, J., Zhou, H., Liu, X., Tian, G., Wu, M. et al. (2019). Dynamic evaluation method of machining process planning based on digital twin. IEEE Access, 7, 19312–19323. DOI 10.1109/ACCESS.2019.2893309. [Google Scholar] [CrossRef]
45. Luo, W., Hu, T., Zhang, C., Wei, Y. (2019). Digital twin for CNC machine tool: Modeling and using strategy. Journal of Ambient Intelligence and Humanized Computing, 10(3), 1129–1140. DOI 10.1007/s12652-018-0946-5. [Google Scholar] [CrossRef]
46. Ma, X., Tao, F., Zhang, M., Wang, T., Zuo, Y. (2019). Digital twin enhanced human-machine interaction in product lifecycle. Procedia Cirp, 83, 789–793. DOI 10.1016/j.procir.2019.04.330. [Google Scholar] [CrossRef]
47. Qi, Q., Tao, F. (2018). Digital twin and big data towards smart manufacturing and Industry 4.0: 360 degree comparison. IEEE Access, 6, 3585–3593. DOI 10.1109/ACCESS.2018.2793265. [Google Scholar] [CrossRef]
48. Li, X., Liu, H., Wang, W., Zheng, Y., Lv, H. et al. (2022). Big data analysis of the internet of things in the digital twins of smart city based on deep learning. Future Generation Computer Systems, 128, 167–177. DOI 10.1016/j.future.2021.10.006. [Google Scholar] [CrossRef]
49. Rathore, M. M., Shah, S. A., Shukla, D., Bentafat, E., Bakiras, S. (2021). The role of ai, machine learning, and big data in digital twinning: A systematic literature review, challenges, and opportunities. IEEE Access, 9, 32030–32052. DOI 10.1109/Access.6287639. [Google Scholar] [CrossRef]
50. Liu, Q., Zhang, H., Leng, J., Chen, X. (2019). Digital twin-driven rapid individualised designing of automated flow-shop manufacturing system. International Journal of Production Research, 57(12), 3903–3919. DOI 10.1080/00207543.2018.1471243. [Google Scholar] [CrossRef]
51. Tao, F., Cheng, J., Qi, Q., Zhang, M., Zhang, H. et al. (2018). Digital twin-driven product design, manufacturing and service with big data. The International Journal of Advanced Manufacturing Technology, 94(9), 3563–3576. DOI 10.1007/s00170-017-0233-1. [Google Scholar] [CrossRef]
52. Zhou, X., Xu, X., Liang, W., Zeng, Z., Shimizu, S. et al. (2021). Intelligent small object detection for digital twin in smart manufacturing with industrial cyber-physical systems. IEEE Transactions on Industrial Informatics, 18(2), 1377–1386. DOI 10.1109/TII.2021.3061419. [Google Scholar] [CrossRef]
53. Ren, Z., Wan, J., Deng, P. (2022). Machine-learning-driven digital twin for lifecycle management of complex equipment. IEEE Transactions on Emerging Topics in Computing, 10(1), 9–22. DOI 10.1109/TETC.2022.3143346. [Google Scholar] [CrossRef]
54. Wang, T., Li, J., Kong, Z., Liu, X., Snoussi, H. et al. (2021). Digital twin improved via visual question answering for vision-language interactive mode in human–machine collaboration. Journal of Manufacturing Systems, 58, 261–269. DOI 10.1016/j.jmsy.2020.07.011. [Google Scholar] [CrossRef]
55. Kunath, M., Winkler, H. (2018). Integrating the digital twin of the manufacturing system into a decision support system for improving the order management process. Procedia Cirp, 72, 225–231. DOI 10.1016/j.procir.2018.03.192. [Google Scholar] [CrossRef]
56. Lohtander, M., Garcia, E., Lanz, M., Volotinen, J., Ratava, J. et al. (2018). Micro manufacturing unit–creating digital twin objects with common engineering software. Procedia Manufacturing, 17, 468–475. DOI 10.1016/j.promfg.2018.10.071. [Google Scholar] [CrossRef]
57. Mortlock, T., Muthirayan, D., Yu, S. Y., Khargonekar, P. P., Al Faruque, M. A. (2021). Graph learning for cognitive digital twins in manufacturing systems. IEEE Transactions on Emerging Topics in Computing, 10(1), 34–45. DOI 10.1109/TETC.2021.3132251. [Google Scholar] [CrossRef]
58. Qamsane, Y., Phillips, J. R., Savaglio, C., Warner, D., James, S. C. et al. (2022). Open process automation-and digital twin-based performance monitoring of a process manufacturing system. IEEE Access, 10, 60823–60835. DOI 10.1109/ACCESS.2022.3179982. [Google Scholar] [CrossRef]
59. Park, K. T., Yang, J., Noh, S. D. (2021). VREDI: Virtual representation for a digital twin application in a work-center-level asset administration shell. Journal of Intelligent Manufacturing, 32(2), 501–544. DOI 10.1007/s10845-020-01586-x. [Google Scholar] [CrossRef]
60. Malik, A. A., Bilberg, A. (2018). Digital twins of human robot collaboration in a production setting. Procedia Manufacturing, 17, 278–285. DOI 10.1016/j.promfg.2018.10.047. [Google Scholar] [CrossRef]
61. Zhang, C., Zhou, G., He, J., Li, Z., Cheng, W. (2019). A data-and knowledge-driven framework for digital twin manufacturing cell. Procedia CIRP, 83, 345–350. DOI 10.1016/j.procir.2019.04.084. [Google Scholar] [CrossRef]
62. Cao, X., Zhao, G., Xiao, W. (2022). Digital twin–oriented real-time cutting simulation for intelligent computer numerical control machining. Proceedings of the Institution of Mechanical Engineers, Part B: Journal of Engineering Manufacture, 236(1–2), 5–15. DOI 10.1177/0954405420937869. [Google Scholar] [CrossRef]
63. Groshev, M., Guimarães, C., Martín-Pérez, J., de la Oliva, A. (2021). Toward intelligent cyber-physical systems: Digital twin meets artificial intelligence. IEEE Communications Magazine, 59(8), 14–20. DOI 10.1109/MCOM.001.2001237. [Google Scholar] [CrossRef]
64. Xie, G., Yang, K., Xu, C., Li, R., Hu, S. (2021). Digital twinning based adaptive development environment for automotive cyber-physical systems. IEEE Transactions on Industrial Informatics, 18(2), 1387–1396. DOI 10.1109/TII.2021.3064364. [Google Scholar] [CrossRef]
65. Zhang, C., Ji, W. (2019). Digital twin-driven carbon emission prediction and low-carbon control of intelligent manufacturing job-shop. Procedia CIRP, 83, 624–629. DOI 10.1016/j.procir.2019.04.095. [Google Scholar] [CrossRef]
66. Guo, H., Zhu, Y., Zhang, Y., Ren, Y., Chen, M. et al. (2021). A digital twin-based layout optimization method for discrete manufacturing workshop. The International Journal of Advanced Manufacturing Technology, 112(5), 1307–1318. DOI 10.1007/s00170-020-06568-0. [Google Scholar] [CrossRef]
67. Park, K. T., Lee, S. H., Noh, S. D. (2021). Information fusion and systematic logic library-generation methods for self-configuration of autonomous digital twin. Journal of Intelligent Manufacturing, 1–31. DOI 10.1007/s10845-021-01795-y. [Google Scholar] [CrossRef]
68. Alexopoulos, K., Nikolakis, N., Chryssolouris, G. (2020). Digital twin-driven supervised machine learning for the development of artificial intelligence applications in manufacturing. International Journal of Computer Integrated Manufacturing, 33(5), 429–439. DOI 10.1080/0951192X.2020.1747642. [Google Scholar] [CrossRef]
69. Zheng, Y., Yang, S., Cheng, H. (2019). An application framework of digital twin and its case study. Journal of Ambient Intelligence and Humanized Computing, 10(3), 1141–1153. DOI 10.1007/s12652-018-0911-3. [Google Scholar] [CrossRef]
70. Conde, J., Munoz-Arcentales, A., Alonso, A., Lopez-Pernas, S., Salvachua, J. (2021). Modeling digital twin data and architecture: A building guide with fiware as enabling technology. IEEE Internet Computing, 26(3), 7–14. DOI 10.1109/MIC.2021.3056923. [Google Scholar] [CrossRef]
71. Liang, Z., Wang, S., Peng, Y., Mao, X., Yuan, X. et al. (2022). The process correlation interaction construction of digital twin for dynamic characteristics of machine tool structures with multi-dimensional variables. Journal of Manufacturing Systems, 63, 78–94. DOI 10.1016/j.jmsy.2022.03.002. [Google Scholar] [CrossRef]
72. Wang, K., Wang, Y., Li, Y., Fan, X., Xiao, S. et al. (2022). A review of the technology standards for enabling digital twin. Digital Twin, 2(4), 4. DOI 10.12688/digitaltwin. [Google Scholar] [CrossRef]
73. Zhang, B., Li, X., Wan, X. X., (2019). From digital twin to digital engineering modeling and simulation entering a new era. Journal of System Simulation, 31(3), 369. [Google Scholar]
74. Leng, J., Wang, D., Shen, W., Li, X., Liu, Q. et al. (2021). Digital twins-based smart manufacturing system design in Industry 4.0: A review. Journal of Manufacturing Systems, 60, 119–137. DOI 10.1016/j.jmsy.2021.05.011. [Google Scholar] [CrossRef]
75. Wang, K. J., Lee, Y. H., Angelica, S. (2021). Digital twin design for real-time monitoring–A case study of die cutting machine. International Journal of Production Research, 59(21), 6471–6485. DOI 10.1080/00207543.2020.1817999. [Google Scholar] [CrossRef]
76. Liu, D., Du, Y., Chai, W., Lu, C., Cong, M. (2022). Digital twin and data-driven quality prediction of complex Die-casting manufacturing. IEEE Transactions on Industrial Informatics, 1–12. DOI 10.1109/TII.2022.3168309. [Google Scholar] [CrossRef]
77. Bao, J., Guo, D., Li, J., Zhang, J. (2019). The modelling and operations for the digital twin in the context of manufacturing. Enterprise Information Systems, 13(4), 534–556. DOI 10.1080/17517575.2018.1526324. [Google Scholar] [CrossRef]
78. Min, Q., Lu, Y., Liu, Z., Su, C., Wang, B. (2019). Machine learning based digital twin framework for production optimization in petrochemical industry. International Journal of Information Management, 49, 502–519. DOI 10.1016/j.ijinfomgt.2019.05.020. [Google Scholar] [CrossRef]
79. Zhang, L., Zhou, L., Ren, L., Laili, Y. (2019). Modeling and simulation in intelligent manufacturing. Computers in Industry, 112, 103123. DOI 10.1016/j.compind.2019.08.004. [Google Scholar] [CrossRef]
80. Wang, J., Ye, L., Gao, R. X., Li, C., Zhang, L. (2019). Digital twin for rotating machinery fault diagnosis in smart manufacturing. International Journal of Production Research, 57(12), 3920–3934. DOI 10.1080/00207543.2018.1552032. [Google Scholar] [CrossRef]
81. Xiao, H., He, L., Li, J., Zou, C., Shao, C. (2021). Permeability prediction for porous sandstone using digital twin modeling technology and lattice boltzmann method. International Journal of Rock Mechanics and Mining Sciences, 142, 104695. DOI 10.1016/j.ijrmms.2021.104695. [Google Scholar] [CrossRef]
82. Zhang, L., Zhou, L., Horn, B. K. (2021). Building a right digital twin with model engineering. Journal of Manufacturing Systems, 59, 151–164. DOI 10.1016/j.jmsy.2021.02.009. [Google Scholar] [CrossRef]
83. Xie, Y., Lian, K., Liu, Q., Zhang, C., Liu, H. (2021). Digital twin for cutting tool: Modeling, application and service strategy. Journal of Manufacturing Systems, 58, 305–312. DOI 10.1016/j.jmsy.2020.08.007. [Google Scholar] [CrossRef]
84. Zhang, H., Qi, Q., Tao, F. (2022). A multi-scale modeling method for digital twin shop-floor. Journal of Manufacturing Systems, 62, 417–428. DOI 10.1016/j.jmsy.2021.12.011. [Google Scholar] [CrossRef]
85. Chen, G., Zhu, J., Zhao, Y., Hao, Y., Yang, C. et al. (2021). Digital twin modeling for temperature field during friction stir welding. Journal of Manufacturing Processes, 64, 898–906. DOI 10.1016/j.jmapro.2021.01.042. [Google Scholar] [CrossRef]
86. Zhang, H., Yan, Q., Wen, Z. (2020). Information modeling for cyber-physical production system based on digital twin and AutomationML. The International Journal of Advanced Manufacturing Technology, 107(3), 1927–1945. DOI 10.1007/s00170-020-05056-9. [Google Scholar] [CrossRef]
87. Bao, Q., Zhao, G., Yu, Y., Dai, S., Wang, W. (2022). Ontology-based modeling of part digital twin oriented to assembly. Proceedings of the Institution of Mechanical Engineers, Part B: Journal of Engineering Manufacture, 236(1–2), 16–28. DOI 10.1177/0954405420941160. [Google Scholar] [CrossRef]
88. Fan, Y., Yang, J., Chen, J., Hu, P., Wang, X. et al. (2021). A digital-twin visualized architecture for flexible manufacturing system. Journal of Manufacturing Systems, 60, 176–201. DOI 10.1016/j.jmsy.2021.05.010. [Google Scholar] [CrossRef]
89. Yu, J., Song, Y., Tang, D., Dai, J. (2021). A digital twin approach based on nonparametric Bayesian network for complex system health monitoring. Journal of Manufacturing Systems, 58, 293–304. DOI 10.1016/j.jmsy.2020.07.005. [Google Scholar] [CrossRef]
90. Dai, S., Zhao, G., Yu, Y., Zheng, P., Bao, Q. et al. (2021). Ontology-based information modeling method for digital twin creation of as-fabricated machining parts. Robotics and Computer-Integrated Manufacturing, 72, 102173. DOI 10.1016/j.rcim.2021.102173. [Google Scholar] [CrossRef]
91. Zhao, P., Liu, J., Jing, X., Tang, M., Sheng, S. et al. (2020). The modeling and using strategy for the digital twin in process planning. IEEE Access, 8, 41229–41245. DOI 10.1109/Access.6287639. [Google Scholar] [CrossRef]
92. Wang, G., Zhang, G., Guo, X., Zhang, Y. (2021). Digital twin-driven service model and optimal allocation of manufacturing resources in shared manufacturing. Journal of Manufacturing Systems, 59, 165–179. DOI 10.1016/j.jmsy.2021.02.008. [Google Scholar] [CrossRef]
93. Luo, W., Hu, T., Ye, Y., Zhang, C., Wei, Y. (2020). A hybrid predictive maintenance approach for CNC machine tool driven by digital twin. Robotics and Computer-Integrated Manufacturing, 65, 101974. DOI 10.1016/j.rcim.2020.101974. [Google Scholar] [CrossRef]
94. Singh, S., Shehab, E., Higgins, N., Fowler, K., Reynolds, D. et al. (2021). Data management for developing digital twin ontology model. Proceedings of the Institution of Mechanical Engineers, Part B: Journal of Engineering Manufacture, 235(14), 2323–2337. DOI 10.1177/0954405420978117. [Google Scholar] [CrossRef]
95. Hu, C., Fan, W., Zeng, E., Hang, Z., Wang, F. et al. (2021). Digital twin-assisted real-time traffic data prediction method for 5G-enabled internet of vehicles. IEEE Transactions on Industrial Informatics, 18(4), 2811–2819. DOI 10.1109/TII.2021.3083596. [Google Scholar] [CrossRef]
96. Liu, Q., Leng, J., Yan, D., Zhang, D., Wei, L. et al. (2021). Digital twin-based designing of the configuration, motion, control, and optimization model of a flow-type smart manufacturing system. Journal of Manufacturing Systems, 58, 52–64. DOI 10.1016/j.jmsy.2020.04.012. [Google Scholar] [CrossRef]
97. Wang, Y., Wu, Z. (2020). Model construction of planning and scheduling system based on digital twin. The International Journal of Advanced Manufacturing Technology, 109(7), 2189–2203. DOI 10.1007/s00170-020-05779-9. [Google Scholar] [CrossRef]
98. Zhou, G., Zhang, C., Li, Z., Ding, K., Wang, C. (2020). Knowledge-driven digital twin manufacturing cell towards intelligent manufacturing. International Journal of Production Research, 58(4), 1034–1051. DOI 10.1080/00207543.2019.1607978. [Google Scholar] [CrossRef]
99. Gaikwad, A., Yavari, R., Montazeri, M., Cole, K., Bian, L. et al. (2020). Toward the digital twin of additive manufacturing: Integrating thermal simulations, sensing, and analytics to detect process faults. IISE Transactions, 52(11), 1204–1217. DOI 10.1080/24725854.2019.1701753. [Google Scholar] [CrossRef]
100. Zhuang, C., Miao, T., Liu, J., Xiong, H. (2021). The connotation of digital twin, and the construction and application method of shop-floor digital twin. Robotics and Computer-Integrated Manufacturing, 68, 102075. DOI 10.1016/j.rcim.2020.102075. [Google Scholar] [CrossRef]
101. Tong, X., Liu, Q., Pi, S., Xiao, Y. (2020). Real-time machining data application and service based on IMT digital twin. Journal of Intelligent Manufacturing, 31(5), 1113–1132. DOI 10.1007/s10845-019-01500-0. [Google Scholar] [CrossRef]
102. Ma, J., Chen, H., Zhang, Y., Guo, H., Ren, Y. et al. (2020). A digital twin-driven production management system for production workshop. The International Journal of Advanced Manufacturing Technology, 110(5), 1385–1397. DOI 10.1007/s00170-020-05977-5. [Google Scholar] [CrossRef]
103. Liu, J., Zhao, P., Jing, X., Cao, X., Sheng, S. et al. (2022). Dynamic design method of digital twin process model driven by knowledge-evolution machining features. International Journal of Production Research, 60(7), 2312–2330. DOI 10.1080/00207543.2021.1887531. [Google Scholar] [CrossRef]
104. Costantini, A., Di Modica, G., Ahouangonou, J. C., Duma, D. C., Martelli, B. et al. (2022). IoTwins: Toward implementation of distributed digital twins in Industry 4.0 settings. Computers, 11(5), 67. DOI 10.3390/computers11050067. [Google Scholar] [CrossRef]
105. Mylonas, G., Kalogeras, A., Kalogeras, G., Anagnostopoulos, C., Alexakos, C. et al. (2021). Digital twins from smart manufacturing to smart cities: A survey. IEEE Access, 9, 143222–143249. DOI 10.1109/ACCESS.2021.3120843. [Google Scholar] [CrossRef]
106. Ramu, S. P., Boopalan, P., Pham, Q. V., Maddikunta, P. K. R., Huynh-The, T. et al. (2022). Federated learning enabled digital twins for smart cities: Concepts, recent advances, and future directions. Sustainable Cities and Society, 79, 103663. DOI 10.1016/j.scs.2021.103663. [Google Scholar] [CrossRef]
107. Serrano, W. (2018). Digital systems in smart city and infrastructure: Digital as a service. Smart Cities, 1(1), 134–154. DOI 10.3390/smartcities1010008. [Google Scholar] [CrossRef]
108. Kim, S., Suh, C. K. (2019). Author co-citation analysis for digital twin studies. The Journal of Information Systems, 28(3), 39–58. [Google Scholar]
109. Tao, F., Sui, F., Liu, A., Qi, Q., Zhang, M. et al. (2019). Digital twin-driven product design framework. International Journal of Production Research, 57(12), 3935–3953. DOI 10.1080/00207543.2018.1443229. [Google Scholar] [CrossRef]
110. Yun, Y., Lee, M. (2019). Smart city 4.0 from the perspective of open innovation. Journal of Open Innovation: Technology, Market, and Complexity, 5(4), 92. [Google Scholar]
111. Fang, Y., Peng, C., Lou, P., Zhou, Z., Hu, J. et al. (2019). Digital-twin-based job shop scheduling toward smart manufacturing. IEEE Transactions on Industrial Informatics, 15(12), 6425–6435. DOI 10.1109/TII.9424. [Google Scholar] [CrossRef]
112. Raes, L., Michiels, P., Adolphi, T., Tampere, C., Dalianis, A. et al. (2022). DUET: A framework for building interoperable and trusted digital twins of smart cities. IEEE Internet Computing, 26(3), 43–50. DOI 10.1109/MIC.2021.3060962. [Google Scholar] [CrossRef]
113. Zhao, R., Yan, D., Liu, Q., Leng, J., Wan, J. et al. (2019). Digital twin-driven cyber-physical system for autonomously controlling of micro punching system. IEEE Access, 7, 9459–9469. DOI 10.1109/Access.6287639. [Google Scholar] [CrossRef]
114. Opoku, D. G. J., Perera, S., Osei-Kyei, R., Rashidi, M. (2021). Digital twin application in the construction industry: A literature review. Journal of Building Engineering, 40, 102726. DOI 10.1016/j.jobe.2021.102726. [Google Scholar] [CrossRef]
115. Angjeliu, G., Coronelli, D., Cardani, G. (2020). Development of the simulation model for digital twin applications in historical masonry buildings: The integration between numerical and experimental reality. Computers & Structures, 238, 106282. DOI 10.1016/j.compstruc.2020.106282. [Google Scholar] [CrossRef]
116. Sun, X., Bao, J., Li, J., Zhang, Y., Liu, S. et al. (2020). A digital twin-driven approach for the assembly-commissioning of high precision products. Robotics and Computer-Integrated Manufacturing, 61, 101839. DOI 10.1016/j.rcim.2019.101839. [Google Scholar] [CrossRef]
117. Židek, K., Piteľ, J., Adámek, M., Lazorík, P., Hošovský, A. (2020). Digital twin of experimental smart manufacturing assembly system for Industry 4.0 concept. Sustainability, 12(9), 3658. DOI 10.3390/su12093658. [Google Scholar] [CrossRef]
118. Sun, X., Liu, S., Bao, J., Li, J., Liu, Z. (2021). A performance prediction method for a high-precision servo valve supported by digital twin assembly-commissioning. Machines, 10(1), 11. DOI 10.3390/machines10010011. [Google Scholar] [CrossRef]
119. Bellavista, P., Giannelli, C., Mamei, M., Mendula, M., Picone, M. (2021). Application-driven network-aware digital twin management in industrial edge environments. IEEE Transactions on Industrial Informatics, 17(11), 7791–7801. DOI 10.1109/TII.2021.3067447. [Google Scholar] [CrossRef]
120. Al-Ali, A. R., Gupta, R., Zaman Batool, T., Landolsi, T., Aloul, F. et al. (2020). Digital twin conceptual model within the context of internet of things. Future Internet, 12(10), 163. DOI 10.3390/fi12100163. [Google Scholar] [CrossRef]
121. Shahat, E., Hyun, C. T., Yeom, C. (2021). City digital twin potentials: A review and research agenda. Sustainability, 13(6), 3386. DOI 10.3390/su13063386. [Google Scholar] [CrossRef]
122. Xue, F., Lu, W., Chen, Z., Webster, C. J. (2020). From LiDAR point cloud towards digital twin city: Clustering city objects based on gestalt principles. ISPRS Journal of Photogrammetry and Remote Sensing, 167, 418–431. DOI 10.1016/j.isprsjprs.2020.07.020. [Google Scholar] [CrossRef]
123. Budel, R. (2019). Siblings make sense of smart cities [urban planning]. Engineering & Technology, 14(1), 42–45. DOI 10.1049/et.2019.0103. [Google Scholar] [CrossRef]
124. Scherrenbacher, S. (2019). No smart factories without a digital twin. ATZproduction Worldwide, 6(4), 36–39. DOI 10.1007/s38312-019-0043-6. [Google Scholar] [CrossRef]
125. Корякина, Е. А., Сидоров, А. Ю., Кулакова, Н. С. (2019). Направления цифровых технологий перехода устойчиворазвивающихся городов в smart city.
, (5), 782–785. [Google Scholar]
126. Ham, Y., Kim, J. (2020). Participatory sensing and digital twin city: Updating virtual city models for enhanced risk-informed decision-making. Journal of Management in Engineering, 36(3), 04020005. DOI 10.1061/(ASCE)ME.1943-5479.0000748. [Google Scholar] [CrossRef]
127. White, G., Zink, A., Codecá, L., Clarke, S. (2021). A digital twin smart city for citizen feedback. Cities, 110, 103064. DOI 10.1016/j.cities.2020.103064. [Google Scholar] [CrossRef]
128. Fan, C., Zhang, C., Yahja, A., Mostafavi, A. (2021). Disaster city digital twin: A vision for integrating artificial and human intelligence for disaster management. International Journal of Information Management, 56, 102049. DOI 10.1016/j.ijinfomgt.2019.102049. [Google Scholar] [CrossRef]
129. Fuller, A., Fan, Z., Day, C., Barlow, C. (2020). Digital twin: Enabling technologies, challenges and open research. IEEE Access, 8, 108952–108971. DOI 10.1109/Access.6287639. [Google Scholar] [CrossRef]
130. Lu, Q., Parlikad, A. K., Woodall, P., Don Ranasinghe, G., Xie, X. et al. (2020). Developing a digital twin at building and city levels: Case study of west cambridge campus. Journal of Management in Engineering, 36(3), 05020004. DOI 10.1061/(ASCE)ME.1943-5479.0000763. [Google Scholar] [CrossRef]
131. Pang, J., Huang, Y., Xie, Z., Li, J., Cai, Z. (2021). Collaborative city digital twin for the COVID-19 pandemic: A federated learning solution. Tsinghua Science and Technology, 26(5), 759–771. DOI 10.26599/TST.2021.9010026. [Google Scholar] [CrossRef]
132. Sepasgozar, S. M. (2021). Differentiating digital twin from digital shadow: Elucidating a paradigm shift to expedite a smart, sustainable built environment. Buildings, 11(4), 151. DOI 10.3390/buildings11040151. [Google Scholar] [CrossRef]
133. Minerva, R., Lee, G. M., Crespi, N. (2020). Digital twin in the IoT context: A survey on technical features, scenarios, and architectural models. Proceedings of the IEEE, 108(10), 1785–1824. DOI 10.1109/PROC.5. [Google Scholar] [CrossRef]
134. Rolle, R., Martucci, V., Godoy, E. (2020). Architecture for digital twin implementation focusing on Industry 4.0. IEEE Latin America Transactions, 18(5), 889–898. DOI 10.1109/TLA.9907. [Google Scholar] [CrossRef]
135. Mykoniatis, K., Harris, G. A. (2021). A digital twin emulator of a modular production system using a data-driven hybrid modeling and simulation approach. Journal of Intelligent Manufacturing, 32(7), 1899–1911. DOI 10.1007/s10845-020-01724-5. [Google Scholar] [CrossRef]
136. Aheleroff, S., Xu, X., Zhong, R. Y., Lu, Y. (2021). Digital twin as a service (DTaaS) in Industry 4.0: An architecture reference model. Advanced Engineering Informatics, 47, 101225. DOI 10.1016/j.aei.2020.101225. [Google Scholar] [CrossRef]
137. Huang, S., Wang, G., Yan, Y. (2022). Building blocks for digital twin of reconfigurable machine tools from design perspective. International Journal of Production Research, 60(3), 942–956. DOI 10.1080/00207543.2020.1847340. [Google Scholar] [CrossRef]
138. Pei, F. Q., Tong, Y. F., Yuan, M. H., Ding, K., Chen, X. H. (2021). The digital twin of the quality monitoring and control in the series solar cell production line. Journal of Manufacturing Systems, 59, 127–137. DOI 10.1016/j.jmsy.2021.02.001. [Google Scholar] [CrossRef]
139. Yang, X., Ran, Y., Zhang, G., Wang, H., Mu, Z. et al. (2022). A digital twin-driven hybrid approach for the prediction of performance degradation in transmission unit of CNC machine tool. Robotics and Computer-Integrated Manufacturing, 73, 102230. DOI 10.1016/j.rcim.2021.102230. [Google Scholar] [CrossRef]
140. Liu, L., Zhang, X., Wan, X., Zhou, S., Gao, Z. (2022). Digital twin-driven surface roughness prediction and process parameter adaptive optimization. Advanced Engineering Informatics, 51, 101470. DOI 10.1016/j.aei.2021.101470. [Google Scholar] [CrossRef]
141. Qamsane, Y., Moyne, J., Toothman, M., Kovalenko, I., Balta, E. C. et al. (2021). A methodology to develop and implement digital twin solutions for manufacturing systems. IEEE Access, 9, 44247–44265. DOI 10.1109/ACCESS.2021.3065971. [Google Scholar] [CrossRef]
142. Xu, J., Guo, T. (2021). Application and research on digital twin in electronic cam servo motion control system. The International Journal of Advanced Manufacturing Technology, 112(3), 1145–1158. DOI 10.1007/s00170-020-06553-7. [Google Scholar] [CrossRef]
Cite This Article
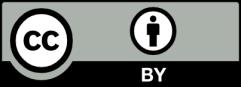
This work is licensed under a Creative Commons Attribution 4.0 International License , which permits unrestricted use, distribution, and reproduction in any medium, provided the original work is properly cited.