Open Access
REVIEW
Low Altitude Satellite Constellation for Futuristic Aerial-Ground Communications
1
Department of Electronic Engineering, Hanbat National University, Daejeon, 34158, South Korea
2
Department of Electrical and Electronic Engineering, Brac University, Dhaka, 1212, Bangladesh
3
School of Business and Digital Technologies, Manukau Institute of Technology, Manukau, 2241, New Zealand
4
Department of Computer Science and Engineering, United International University, Dhaka, 1212, Bangladesh
* Corresponding Author: Al-Sakib Khan Pathan. Email:
(This article belongs to the Special Issue: Issues and Challenges in Futuristic Aerial-Ground Networks)
Computer Modeling in Engineering & Sciences 2023, 136(2), 1053-1089. https://doi.org/10.32604/cmes.2023.024078
Received 23 May 2022; Accepted 09 October 2022; Issue published 06 February 2023
Abstract
This paper discusses the significance and prospects of low altitude small satellite aerial vehicles to ensure smooth aerial-ground communications for next-generation broadband networks. To achieve the generic goals of fifthgeneration and beyond wireless networks, the existing aerial network architecture needs to be revisited. The detailed architecture of low altitude aerial networks and the challenges in resource management have been illustrated in this paper. Moreover, we have studied the coordination between promising communication technologies and low altitude aerial networks to provide robust network coverage. We talk about the techniques that can ensure userfriendly control and monitoring of the low altitude aerial networks to bring forth wireless broadband connectivity to a new dimension. In the end, we highlight the future research directions of aerial-ground communications in terms of access technologies, machine learning, compressed sensing, and quantum communications.Graphic Abstract
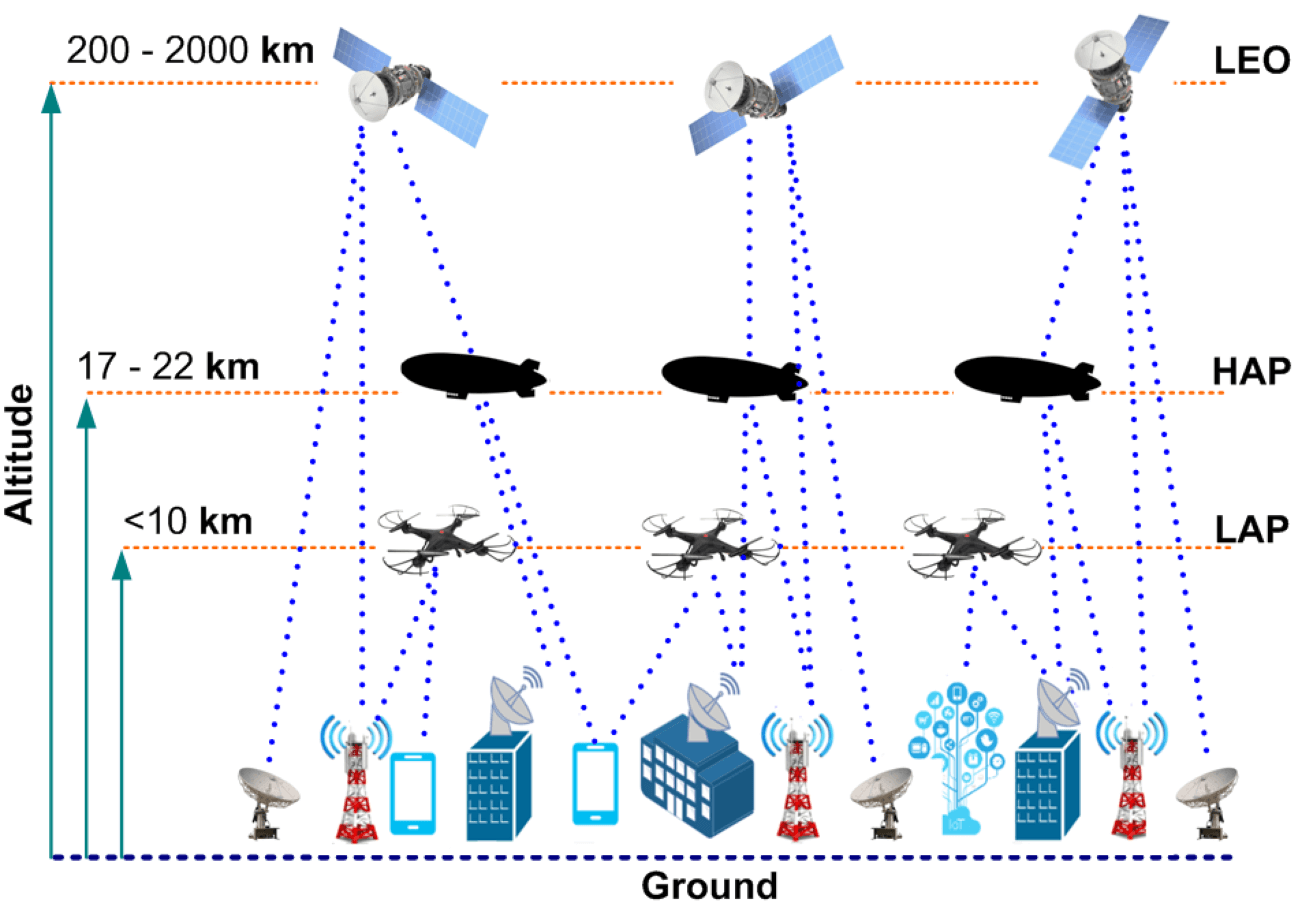
Keywords
Beyond fifth-generation (B5G) wireless networks provide a wide variety of application-focused data services to satisfy the growing appetite of mobile consumers. Reliable and secure data services in B5G wireless networks need the seamless integration of fixed and wireless terrestrial network systems, as well as modern satellite communication (SATCOM) systems. The next-generation wireless networks, known as 5G, have three primary objectives. Specifically, they include enhanced mobile broadband (eMBB), massive machine-type communication (mMTC), and ultra-reliable low-latency communication (URLLC) [1–3]. Indeed, SATCOM is excellent for broadcasting or multicasting multimedia material to a wide, geographically dispersed audience [4–6]. Mobile operators and Internet service providers may use this sort of communication to multicast material to the network edge, allowing for content caching for local distribution [4,7]. Again, edge computing can squeeze aerial interconnectivity to boost broadband communication for more people. Multi-satellite systems can offload traffic and improve infrastructure architecture in SATCOM for 5G [4,8,9]. The satellite-terrestrial network for 5G (Sat5G) and others plan to incorporate satellite into 5G [7,8].
The integration of aerial networks with 5G terrestrial networks requires new technologies to provide seamless aerial-ground wireless connections [10,11]. Another concept named “Network Virtualization” shows great promise in terms of lower costs, higher flexibility, and customized service provisioning. The adoption of software-defined network (SDN) and network functions virtualization (NFV) technologies into the satellite network domain is seen as a significant approach to obtain the successful incorporation of satellite and mobile terrestrial networks, easing the formation of the B5G radio network architecture [11,19,20]. Traditional SATCOM systems, such as geostationary earth orbit (GEO) and medium earth orbit (MEO), have some complexities in providing desired services owing to their very high altitude from the ground [15]. Given that a constellation of numerous low earth orbit (LEO) satellites, alternatively high altitude platforms (HAPs), and low altitude platforms (LAPs) has received significant attention in recent times to provide massive uninterrupted broadband wireless connectivity in B5G cellular networks [21,22]. Thus, aerospace network devices have received significant attention in recent times. HAPs and LAPs aerial segments can be instrumental in managing unmanned aerial vehicle (UAV) networks, which is a hot topic in the wireless communications arena today [23,24]. HAPs and LAPs can also be useful for disaster warning, monitoring, and management purposes with a greater ability to extend the radio network coverage in the areas affected by natural calamities [25,26].
Enabling connection through SATCOM is one method that has recently gained popularity for improving ground-level communication. Table 1 provides a concise summary of recent evaluations and surveys in relation to SATCOM, allowing the reader to quickly grasp the primary focus of each of the previously conducted surveys. As far as we have investigated, there is no UAV-integrated SATCOM network in which UAVs serve as relays, as shown in Table 1. In addition, we did not find the SATCOM system that was part of the cognitive radio (CR) network. Furthermore, we did not find new B5G techniques like non-orthogonal multiple access (NOMA), intelligent reflecting surface (IRS), and Internet of Space Things (IoST). In this study, we review the prospects of futuristic SATCOM to meet the rising demands of B5G wireless networks. We analyze the integration of promising technologies to make seamless aerial-ground communication systems as well as to bring significant improvements in futuristic aerial-ground networks. The following is a summary of the major contributions:
• We classify the architecture of low altitude aerial networks.
• We discuss the resource management of low altitude satellite networks.
• We examine recent advancements in promising satellite network technologies.
• We go through some of the outstanding concerns and challenges in SATCOM, as well as some key research prospects for the future.
The rest of the paper is organized as follows. In Section 2, the architecture of low altitude aerial networks is illustrated. In Section 3, the resource management for low altitude satellite networks has been discussed in detail. Section 4 discusses the promising technologies that can be incorporated to achieve the full benefits of satellite networks. Application scenarios for satellite networks are presented in Section 5. In Section 6, future research directions for further improvement of smooth aerial-terrestrial communications have been highlighted. Finally, Section 7 concludes the paper. Before going into the topic-wise discussions, in Table 2, we present the list of key acronyms used throughout this paper.
2 Architecture of Low Altitude Aerial Networks
In this section, we describe the comprehensive architecture of the low altitude aerial network systems. We also highlight the potential of LEO satellites, HAPs, and LAPs in providing efficient aerial-ground communication for next-generation heterogeneous wireless networks.
Fig. 1 depicts the scenario of low altitude aerial networks. LEO satellite networks provide new ways to access very high-speed Internet services while extending radio coverage to terrestrial networks. The LEO satellite orbits between 200 and 2000 km above the earth [7]. Due to the potential for delivering global wireless access with higher data rates, LEO SATCOM has recently attracted widespread study interest. The LEO SATCOM systems have far fewer requirements than their GEO counterpart, such as power consumption, deployment cost, transmission signal delays, and maintenance complications. The propagation delay of LEO satellites is greatly decreased to less than 14 ms due to their low altitude compared to MEO and GEO satellites [12,16]. Users can expect less than 50 ms of round-trip delay, which is comparable to terrestrial lines. The latency could be further reduced by deploying small satellites densely in this orbit to be connected with the most suitable and nearest terrestrial satellite terminals. The production cost can be reduced significantly if the size of the satellite is reduced. Multiple satellites can provide coverage for the same target area by grouping the small satellites in different orientations in an ultra-dense LEO satellite topology [27,28]. Hence, it would be possible for each terrestrial satellite terminal to be connected with multiple satellites at the same time. This facilitates continuous uninterrupted data services to meet the demands of B5G wireless networks [17].
Figure 1: The scenario of low altitude aerial networks
Well incorporated aerial-ground communication systems can empower extensive scopes corresponding to thousands of cells, and in this manner, support both broadcast and multicast services. Again, application-specific services can be made more robust and user-friendly. This is particularly efficient for making aerial-ground communication scalable. Unfortunately, satellite-based communications have a low penetration rate in today’s telecommunication infrastructure due to two drawbacks: end-to-end transmission control protocol/Internet protocol (TCP/IP) throughput degradation over satellite links and limited satellite capacity in point-to-point mode. Moreover, ultra-dense LEO satellite constellations have few challenges such as asynchronous signal detection by the terrestrial terminals due to the placement of satellites at various altitudes, beam overlapping among different satellites, adjacent channel interference, interference between inter-satellite links, and so on [29]. All these issues need to be mitigated to ensure smooth aerial-ground communications.
By considering their easy and cost-effective installation, HAPs and LAPs can more conveniently meet the aerial network support necessary for B5G wireless networks. HAPs are quasi-stationary aerial platforms in the stratosphere between the terrestrial ground station and space satellite that are placed at a height of 17–22 km above the ground considering the air turbulence impact of the earth [22,26]. The supporting physical mechanism that provides the lifting power is utilized to classify aerial devices used for HAPs. These platforms could be unmanned airplanes or balloons to ensure seamless communication between terrestrial transceivers and space satellites. In practice, HAPs often utilize the convenient amenities of satellite and ground communication systems in many aspects. For instance, they take advantage of large coverage area and low propagation delay compared with satellites, signal strength, transmission capacity/multicast, low upgrade cost, incremental deployment, reduced ground infrastructure, and short take-off and landing time for maintenance purposes [26].
HAPs can be used for a variety of scenarios, including broadcast/multicast signals, extremely high-speed wireless connectivity, navigation and global positioning systems (GPS), intelligent transportation systems, surveillance, remote sensing, naval engineering, weather monitoring, emergency communications, and disaster management [22]. It is also capable of providing very high-speed backhaul links to reduce the continuously soaring traffic burden of cellular networks [30]. The purpose of creating B5G wireless systems and services is to provide seamless delivery of broadband multimedia applications across heterogeneous networks. Integrating satellite-aerial-terrestrial networks is crucial. To attain long-term profitability because to their low altitude, minimal propagation delay, and cost-effectiveness compared to the satellite constellation, additional HAP technologies must be integrated into satellite and terrestrial networks.
Even though HAP-assisted technology opens up a lot of opportunities, it also presents a lot of challenges. Some of the most important ones are high quality-of-service provisioning, context-aware services and architectures, seamless integration at the user level, service integration, different access technologies and protocols, taking advantage of heterogeneous systems, integrated traffic modeling, cross-layer approaches, and overall coordination between satellite, aerial, and terrestrial networks.
Low altitude platforms (LAPs) are effective in providing high-speed, wireless broadband connections to rural areas and in emergency situations such as natural disasters or network outages. To address the requirement for exceptionally fast data support in B5G wireless networks, a large number of macro cells equipped with fixed macro base stations (BSs) will be installed in the coverage area. The restoration of fixed cellular networks would be time-consuming and not cost-effective if network service in any macro cell is disrupted by natural disasters. In such instances, the deployment of LAPs is the alternate approach for rapid and cost-effective network restoration [31,32].
LAPs are aerial platforms that can fly at altitudes of less than 10 km, which is normally inside the troposphere [33]. These platforms often take the shape of quadcopters, balloons, or unmanned helicopters. LAPs are distinct from HAPs in both the operational altitude at which they may function and their capacity to provide wireless broadband access.
3 Resource Management: Low Altitude Satellite Networks
This section discusses how network resources could be managed in order to operate low altitude satellite networks. It also discusses the difficulties of allocating radio resources and suggests some appropriate ways of doing so.
Here, we present the information pertaining to the different frequency bands that were proposed to be utilized by the abovementioned architecture for 5G communications. The previous section discussed the architecture and infrastructure necessary to increase support for higher bandwidth of data, how LEOs, HAPs, and LAPs would need to communicate with each other. Fig. 2 depicts the popular satellite frequency bands in different applications.
Figure 2: Popular satellite frequency bands
This part discusses two of the major carrier frequencies being considered for use in 5G communication purposes, namely the C-band and the K-band. The millimeter wave (mmWave) bands and terahertz (THz) frequency bands have research activities ongoing, namely for B5G applications and solutions. In this section, a detailed comparison between the four bands will be discussed, from their advantages to their disadvantages, as well as their associated applications for different modes of communication [34–36].
The need for 5G communication arises due to the increasing volumes of data traffic generated year after year. For instance, in 2020, for instance, through utilizing techniques like multiple-input and multiple-output (MIMO) and orthogonal frequency-division multiplexing (OFDM), data handling efficiency has been pushed to certain kinds of limits, and so, going forward, with the current trend, in the upcoming years, this simply cannot be sustained by the existing infrastructure. There are ever-increasing demands for data traffic. Surges in mMTCs, as an example, alone would demand an enormous consumption of data traffic whilst requiring novel communication needs. The problem we are faced with is that the existing frequency bands that are being used as carriers (600 MHz to 2.4 GHz) cannot support the growing data traffic demands as they could in the past up to 4G. Therefore, 5G is indeed a much more radical shift in communication technology, which would prepare the world and equip it with the starting tools necessary to accommodate for and satiate the ever-growing demands in traffic load consumption amongst other, even unheard of, communication needs [37,38].
The most important band in 5G, arguably, is the C-band, and it is also known as the mid band. According to IEEE’s classification, the C-band uses microwave frequencies in between the range of frequencies of 4–8 GHz and has wavelengths in the range of 3.75–7.5 cm for wireless communications. However, despite this fact, the U.S. Federal Communications Commission C-band proceeding and auction designates 3.7–4.2 GHz as the C-band. The C-band can be used for many SATCOM transmissions, some Wi-Fi devices as well as some cordless telephones, and in addition, some types of surveillance and weather radar systems. In the USA, operators such as Verizon and AT&T use C-band within the range of 3.7 to 3.98 GHz. It is also important to note that frequencies which are on the low-bands will not become obsolete or go out of order, but rather, they will continue to provide support to the mid-band as backhaul providing important services alike [10,13,14].
Over the course of time, the C-band has gained enormous popularity and is the most preferred form of 5G frequency carrier to be utilized in most countries. There are numerous reasons for its popularity, which can be summarized as its ability to balance speed, capacity, and coverage, as well as its penetration power for densely populated areas with high connectivity demand. The C-band will be the most important band in 5G communications, primarily due to its wide network coverage as well as its ability to retain its robustness even during inclement weather conditions, where higher bands suffer terribly due to attenuation and path-loss such as rain fading and scattering [39,40].
The C-band can be thought of as a doorway which allows for integration of lower, existing bands with their higher counterparts (which have greater bandwidths and frequencies). This is the most appropriate and dependable band for ensuring high service availability while sacrificing speed (which the higher bands can give), a required but worthwhile tradeoff. Even in spite of this compromise, it is still certainly a step up in comparison with the existing 4G standard. The C-band will be able to support speeds of upwards of 100 Mbps initially and increase to 10 Gbps speeds over time and in the long run when integrated successfully with mmWaves or Ka-band waves as carriers. It is this band which will connect the lower bands of existing frequencies to those of higher ones. High-speed connections with reasonably good coverage can be obtained due to sufficient open frequency space delivery [41,42].
Previously, target applications were primarily designed for TV broadcasting and telephony; however, in today’s world, this has shifted significantly towards and into Internet services [43]. With recent advancements in 5G technology, the need for a heterogeneous satellite-terrestrial network is becoming increasingly important. The K-band in communications consists of a Ku-band and a Ka-band. These two bands fall under the portion of the electromagnetic spectrum with microwave frequencies spanning ranges between 12–18 GHz and 26.5–40 GHz, respectively. Today, conventional SATCOMs essentially make use of frequency bands in the range of 1 to 40 GHz. Due to low frequency band saturation, relatively higher frequency bands, those above 20 GHz, such as the Ka-band (27–40 GHz), have been explored to greater depths and degrees, as the Ka-band can provide stable broadband services [44]. Newer satellite networks should provide mobile satellite services (MSS) to be compatible with terrestrial mobile networks integrating 5G technology [12].
Altitudes between 500 and 1500 km are typically preserved for LEO satellites. The reduced propagation delay of LEOs enables real-time communications with high throughput [45]. The reduced propagation delay of LEOs enables real-time communications with high throughput. A low satellite altitude also reduces signal attenuation as well as power requirements for satellites. Both of these parameters are important for making terminal devices smaller [46]. LEO satellites will play a vital role in future ubiquitous systems. As a solution to broadband as well, several companies are using different parts of the Ka-band for satellite constellations of LEOs such as Oneweb, Telesat, and Space-X’s Starlink as examples [18].
In the past, due to technological constraints, traditional satellite networks determined user-scheduling and data forwarding issues to ground hub stations [47]. Today, however, it is well within reason to expect that the on-board processing schemes will be feasible to use in the sky as there is a significant advancement in application-specific integrated circuits as well as powerful central processing unit technologies. If we can achieve communication networking for the on-board payload, it is possible to reduce the total end-to-end latency of satellite systems by eliminating unnecessary round-trip delays (like those for receiving the control signal) [48]. In this way, we can conserve valuable radio spectrum by decreasing the need for feeder links from/to gateways. Furthermore, with the on-board processing scheme, satellite networks would also be able to support higher throughput in multiple beams. Potentially, inter-satellite links can be supported by these up/down links by efficiently exploiting routing and scheduling designs, and in doing so, it is possible to achieve high-throughput satellites which can offer services up to 100 Gbps. With new and cutting-edge technology, it can also handle a large volume of on-board computation [47].
The advantage of higher frequency bands, such as the Ka-band, is that a higher bandwidth is available. As for the novel idea of operating in the Ka-band over the Ku-band, there are some significant and hard-to-achieve advantages that can be obtained in the Ka-band and not in the Ku-band or lower frequency bands. Conventional networks of satellites operate in the Ku-band and its associated lower frequencies. However, alongside more available bandwidth at the higher Ka-band frequencies, the Ka-band antennas have relatively higher gains as compared to Ku-band antennas (or even the lower frequency bands), which are similar and of comparable size. In other words, the same antennas of the same size in the Ka-band will have higher gains.
The disadvantage, on the other hand, is that attenuation of the signals can take place rather rapidly as opposed to lower band frequencies (and conversely, higher wavelengths), such as the C-band and Ku-band, making it unsuitable for use in ubiquitous connectivity. This is one of the reasons that the C-band is so popular in 5G. In addition, another disadvantage to be addressed is that inclement weather, such as heavy rainfall or path-loss through trees or buildings, can significantly attenuate Ka-band signals. It is therefore imperative for well-designed ground systems to be implemented when constructed. From maritime communications, we can derive information on how to achieve that, as they have been using the K-band for establishing SATCOM links (Ku-band) for a long time. This needs to now be extended to the world of 5G communications. The network links’ reliability and resources need to be addressed in order to mitigate these adverse weather effects [49,50].
The high attenuation of K-band frequencies makes them unsuitable for long-range transmission. The K-band is usually used for SATCOMs, astronomical observations, and radars. In this frequency range, radars can provide short range, high resolution, and high throughput. Combining the C-band for wider coverage along with the K-band, more specifically the Ka-band, will be a significant research area in the upcoming days. For instance, it could be a good way to improve seamless integration by delivering both wide network coverage and the 5G promise of ultra-low latency with incredibly high speeds. It is also necessary to develop modulation and coding schemes for both the integration of bands and to look into ways of making the Ka-band frequencies prone to less attenuation in bad weather conditions. An automated cognitive network environment can also help switch seamlessly between the C-band and K-band as deemed necessary [51,52].
Figs. 3 and 4 show the signal-to-noise ratio (SNR) for mmWave and THz links at various distances (5, 30, and 100 m) [53]. To investigate the influence of a higher carrier frequency on propagation loss, the SNR is calculated as the ratio of received signal power to noise power (without beamforming gain). The carrier frequency decreases linearly in Fig. 3. This is because mmWave is solely concerned with spreading loss. Fig. 4 shows a drop in carrier frequency with fast fluctuations. This is because a THz connection consists of both spreading and molecule absorption losses [54–56]. The attenuation caused by the propagation properties of a wave across the medium is known as the spreading loss (or free-space loss). The molecular absorption loss of ambient gases is quite high due to molecular resonances in the THz band induced by water vapor and oxygen [57,58].
Figure 3: Impact of transmitter-receiver pair distance on SNR for mmWave link
Figure 4: Impact of transmitter-receiver pair distance on SNR for THz link
3.2 Antenna Gain and Narrow Spot Beam
Because of the difficulties in working with it in the past, Ka-band was not widely used. However, by incorporating advanced and adaptive modulation and coding techniques, Ka-band systems can now meet high data-rate requirements. Traditional mobile antenna systems, like the reflector antennas that are available, are not suitable for satellite service applications in mobile environments or stationary environments needing low-impact devices. These drawbacks necessitate the development of terminal technologies for satellite services like phased array technology. The technology for mobile SATCOM is becoming more prevalent in the commercial markets for mobile satellite terminals. These arrays are steered electronically with extremely high tracking accuracy as well as having the ability to maintain a very low profile [59]. Well-designed terminals for mobile applications can allow for continuous communication with multiple satellites.
Beam-forming technology that is both reliable and secure, as well as constant broadband connections, are crucial to achieving these overarching aims. The needs may be successfully met by satellite networks [60–62]. With higher frequencies in the Ka-band, lighter weight and proportionally smaller antennas could reduce the costs associated with materials for the antennas [49]. This reduction can allow for the Ka-band to be available for mobile platforms in SATCOM. The benefit of using Ka-band satellites is that they can support very narrow spot beams which have widths of 3 dB at 0.5° to 1.5° [49]. This allows for a larger frequency reuse and a larger frequency allocation, which can increase the throughput. These spot beams are narrow and can be turned toward users for high-speed or low-latency communications [49]. Utilizing more focused, narrower spot beams can provide higher equivalent isotropic radiated power, signal gain, and as a result, better signal link quality as well as higher data rates for smaller terminals. When compared to the Ku-band, the Ka-band had overall improvements in its link quality (by efficiently using narrow spot beams) in the range of 6 to 10 dB [49].
The SATCOM link passing through the atmosphere can get degraded because of various weather conditions such as rain, water vapour, ice, clouds, hail, and snow [63]. As a result, the greatest challenge in utilizing the Ka-band over the Ku-band is the high rain attenuation as well as the higher rainfall rates, shown in Fig. 5, especially in tropical regions. As a result, attenuation per unit length rises in the Ka-band due to the higher electromagnetic wave absorption. At the Ka-band, rain fade along with attenuation will be higher and this high rain fade will have effects on the adaptive coding and modulation algorithms (which are some measures of mitigation) built into satellite models. At the ground segment, mitigation techniques can improve link quality while environmental factors can reduce link quality. The Ka-band in SATCOM can facilitate new and relatively smaller mobile terminals which can use high-throughput applications as opposed to the Ku-band. However, because of the relatively larger rain attenuation, the Ka-band link’s design analysis is more complex than lower frequency bands to compare for link availability. Sensitivity and trade-off analysis determine the feasibility of using Ka-band in SATCOM in different regions [49].
Figure 5: Basic model of rain fading for satellite communication
Absorption and scattering create considerable attenuation above 10 GHz. Ka-band can carry a lot of data and provide high-speed communication, but it is weather sensitive [64,65]. Rain not only attenuates the signal throughout the transmission route, but its form may also affect its polarization. Surface rainfall consists of a range of raindrops, best represented by mean drop size and droplet concentration. The drop size distribution (DSD) of rain is crucial for evaluating rain intensity and latent heating profiles using remote sensing data and for parameterizing rain microphysics in numerical weather forecasting models. At mmWave frequencies, rain attenuation depends on DSD. In weather data, there are 300 values for the number of particles with a diameter between 0.1 and 30 mm and a particle size bin of 0.1 mm, along with their average velocity [66].
Apart from rain fade/fading and attenuation, other Ka-band operation elements to consider are as follows:
1. There is a fallback possibility to lower frequency bands during severe fade conditions. Then, there could be a real need to change transmission plans, which would necessitate the crossing over of multiple spot beams to cover the operational area [67].
2. Users’ movements can degrade performance. This is inevitable as the channel variation from multipath and mobile environments is faster than the round-trip delay of the GEO satellite link. For future MSS systems, further studies are required on the impact of super high-frequency relative to high speed environments so that a stable performance can be provided [68]. In Lutz’s and Loo’s channel models, high frequency band SATCOM systems, with rain attenuation considerations as well as user movements, were taken into account and investigated [68].
3. Rayleigh fading with a Doppler effect also needs to be taken into account for terrestrial communications.
3.4 Propagation Delay and Doppler Shift
In this section, the propagation delay and the Doppler shift of the relay node (RN) signals’ resources are investigated. Due to the rotation of the earth and the movement of LEOs, there is a Doppler shift from the original signal sent from the gateway (GW) to the LEO and then again when the signal is sent back. The round-trip-time (RTT) delay experienced by the terrestrial BSs compared with the altitude of the LEO satellites is called propagation delay. The first parameter discussed is the propagation delay, which can be given by the 1-way delay as:
where
Fig. 6 shows that the RTT delay decreases with increasing eNB elevation angles and GW elevation angles. At 0 degrees, the RTT time delay is the highest at 44 ms and starts leveling off at 26 ms near 45 degrees with relative constancy at 90 degrees. The change can be seen to be more non-linear and more similar to an exponential decrease.
Figure 6: Round-trip-time delay changes with eNB elevation angle
In Fig. 7, it can be seen that the RTT delay increases as the altitude of the LEO satellites increases. Different plots are shown for different pairs of elevation angles with respect to eNB and GW elevation angles. It should be noted that the changes are linear with respect to altitude. For eNB elevation angle of 60° and GW elevation of 30°, the RTT delay is higher altitude than eNB elevation angle of 30° and GW elevation of 60°. From the observation of three elevation angles, the lower elevation angle has the higher RTT delay.
Figure 7: Round-trip-time delay as a function of altitude
The Doppler shift is experienced by the signals as they propagate through free space [70]. This is due to the constant relative changes in the speeds of the LEOs with respect to the earth, as neither is stationary and is instead traveling at high speeds. The equation for the Doppler shift as a function of the elevation angle can be expressed as:
where
As shown in Fig. 8, we can get the plot of the Doppler shift (
Figure 8: Doppler shift changes with elevation angle
4 Incorporating Promising Technologies
The SATCOM networks are made up of three important features that future networking systems will need to combine and take into account.
4.1 Non-Orthogonal Multiple Access
Non-orthogonal multiple access (NOMA) has the potential to provide higher throughput than traditional orthogonal multiple access (OMA), which has been thought of as a key technology for SATCOM networks [71]. NOMA uses transmitter precoding to distinguish power domain users. Multiple-user messages are precoded using superposition coding at the transmitter, and a single-user receiver must decode and eliminate conflicting streams before decoding its own message. NOMA in a SATCOM system can give users access continually with better spectral efficiency and system capacity. But the satellite channels are different from the terrestrial channels in that they have a huge time delay and a Doppler shift. Nowadays, the OMA technique is the most prevalent in SATCOM networks [72]. Frequency division multiple access/Time division multiple access/Code division multiple access may eliminate intra-beam interference and ease signal identification, but a single orthogonal resource block can only serve one user, limiting spectrum efficiency and satellite network capacity. OMA methods cannot handle the enormous rise in traffic demand for future SATCOM networks due to poor spectrum usage efficiency and restricted user numbers [73]. The main benefit of NOMA is that it has the potential to improve spectral efficiency in situations where there are a lot of users using the same time-frequency resources. This can be done by giving users channels that are closely aligned but have different channel strengths. Even though successive interference cancellation (SIC) reaches the capacity region of the scalar Gaussian broadcast channel, as the number of users goes up, the number of SIC layers goes up, and SIC error propagation gets worse [74].
Fig. 9 shows the channel capacity for OMA and NOMA schemes in finite blocklength theory (FBT) mode over the THz band. The channel capacity then begins to decline and diverge a little before continuing at 1.172 Mbps at 2.5 THz. For example, the capacity of both NOMA and OMA systems at 1.5 THz frequency is 2.333 and 2.113 Mbps, respectively. According to the findings in Fig. 9, at carrier frequencies less than 2.5 THz, such as 1.5 THz, switching from NOMA (2.333 Mbps) to OMA (2.113 Mbps) results in a channel capacity loss of nearly 0.220 Mbps. As a consequence, we get a
Figure 9: Channel capacity as a function of frequency for NOMA and OMA schemes
Fig. 10 shows the transmission latency for OMA and NOMA systems in FBT mode over the THz range. The transmission latency rises with increasing frequency, as seen in the graph. Both systems have a transmission latency of roughly 0.0348 ms for a considerably lower frequency of 0.2 THz. Until 4.41 THz, the transmission latency of the two systems increases slowly. After this point, the latency performance curves increase gradually, with NOMA having higher latency than OMA. For example, at an 18 THz frequency, the NOMA and OMA schemes have latencies of 8.522 and 6.522 ms, respectively. For example, moving from NOMA (8.522 ms) to OMA (6.522 ms) at a frequency of 18 THz results in a 2 ms latency. Because reduced latency is usually desirable, adopting OMA here would result in a
Figure 10: Transmission latency as a function of frequency for NOMA and OMA schemes
In addition to the traditional approaches of employing space division multiple access (SDMA) and NOMA, rate-splitting multiple access (RSMA) has emerged as a viable solution for managing interference in multi-user multi-antenna networks [75]. RSMA is supported by multi-antenna rate-splitting at the transmitter and successive interference cancellation at the receivers. Due to its versatility in reducing interference via common message decoding, RSMA acts as a generic multiple access and reduces to SDMA, NOMA, OMA, and multicasting when assigning powers to the various kinds of message streams. Si et al. [76] studied the innovative transmission strategy of RSMA to reduce interference in the forward link of a multigateway multibeam satellite system. The benefit of RSMA is that it offers grant-less and asynchronous transmission, which lowers the signaling cost. The drawback is that when a rake receiver is used, its user overload is limited. The overload may be improved by employing an advanced receiver, such as a SIC-based receiver [77].
Cooperative communication is essential not just for wireless relaying to improve transmission range but also to increase capacity. As for users situated towards the edge of a cell, they may experience signal attenuation owing to severe path loss from lengthy distances from the BS. As a result, it would be essential to establish relays with permanent relaying nodes, mobile relaying platforms, or both. The infrastructure of a communication network may include UAVs, LEOs, and LAPs as part of a cooperative network system. It is the point at which various hops may intelligently interact with one another to maximize spectrum and resource sharing.
As airborne mobile relays, UAVs aim to discover optimal places and advantageous channel conditions, such as line-of-sight (LoS) connections. This is done by dynamically altering location to improve cooperative communication even if the source-to-destination connection is obstructed. This helps delay-tolerant applications [78,79]. Several UAV regulatory agencies developed and implemented communication standards to standardize UAV communication. Satellites are another key aspect of cooperative communication networks [68]. The work in [80] proposed LEO’s use of threshold-and-forward to increase dependability. Multi-satellites may receive and forward user signals using threshold-and-forward. Satellites evaluate incoming signals up to a predetermined threshold. If the received signal exceeds the threshold, it is limited. Lower values are linearly processed and sent to the recipient. The receiver combines and decodes satellite signals. Simulation findings in [80] indicate that the threshold-and-forward protocol outperforms previous methods (like amplify-and-forward and decode-and-forward (DF) in multi-satellite cooperative scenarios).
A single satellite often transmits user communications to the gateway station in traditional satellite networks. Due to their lower altitudes, LEOs have restricted coverage, a short available time, the Doppler effect, and shadow fading in the satellite-ground connection. Because of this, it is challenging for a single satellite to provide high data transmission speeds and dependability. These issues may be resolved by using many satellite systems, and cooperative transmission technology can then achieve diversity to raise transmission dependability overall [80].
4.3 Cognitive Radio Networking
Cognitive radio (CR) networking refers to the sharing, usage, and optimization of spectral bandwidth and network resources [81]. It enables the efficient and effective use of resources between relay nodes (such as UAVs, LAPs, and satellites) and UEs. Application areas such as traffic surveillance, agricultural monitoring, border patrolling, and disaster management may exploit the integration of UAVs with CR [82]. The benefit is that UAV may reduce the high path-loss and blockage-prone issues experienced by mmWave by establishing LoS connections between mobile users and the nearest BS [83]. Not only may UAVs enhance coverage and transmission rates, but their superior signal and data processing capabilities also allow real-time decision making for various monitoring objectives [82]. They may also be used for marine communication and in emergency scenarios like natural disasters.
In [84], the integration of UAVs into CR networks was evaluated with a particular emphasis on the performance of cognitive URLLC and mMTC services based on NOMA. Massive MTCs need improved energy efficiency and connection probability, while URLLC services are primarily concerned with minimizing latency. By sharing unlicensed wireless spectrum with terrestrial BSs, cognitive UAVs may be utilized as secondary aerial broadcasters [85]. In [84], the analytical expressions of throughput, energy efficiency, and latency for the mMTC/URLLC-UAV device were explored. Using the Langrangian technique with Karush-Kuhn-Tucker conditions, the optimization challenge of maximizing energy efficiency to meet URLLC latency and mMTC throughput was also addressed. The numerical results of [84] showed that the suggested technique improves energy efficiency and meets the mMTC/URLLC-UAV device’s latency requirements.
In a licensed spectrum that is overloaded, the problem of MTC performance deteriorates. A spectrum shortage is thus one of the most crucial concerns in 6G development [86]. The continuously expanding data traffic in wireless communications has rendered the unlicensed spectrum band indispensable for large-scale and diversified MTC operations, since it permits the aggregation of multiple bands to increase the capacity of future wireless systems. As a result, the 3GPP established unlicensed (NR-U) spectrum access as a method of delivering critical technologies to assist future business and society [84]. The LTE conference has advocated employing an unlicensed band (LTE-U) as part of unlicensed activities to ameliorate the bandwidth shortage issue as an alternative approach. As a result, spectrum sensing CR technology has been built to access unlicensed portions of the spectrum with the assistance of substantial amounts of bandwidth [84].
One of the main problems with keeping a UAV-based cognitive network running is that it uses a lot of energy. This is also a major area of research, as attempts to figure out how to keep the UAV in the air for long periods of time and run all of its operations, such as on-board processing [87]. A UAV works because it has things like an antenna array, an amplifier, radio circuits, etc., on board. All of these processes need a power supply, and [88] looks at how to get the required levels of energy efficiency. Transmit antenna selection improves wireless transmission for UAV-based CR. Energy harvesting technologies will be used for a green CR network based on radio frequency to allow realistic propagation and reduce device battery utilization [88].
5 Application Oriented Satellite Networks
Applications in the networking system are discussed, including UAVs, IoRT, IoST, vehicular networks, and smart cities.
UAV or drone-assisted wireless communication, is gaining immense appeal due to its increased adaptability to many applications. Fig. 11 displays an example use of UAVs for SATCOM between BS and LEO satellites. UAVs may provide mobility, low-cost installation and maintenance, and a variable flying capability, which makes them a viable option to permanent access points or macro BSs, particularly for short-term deployments in emergency situations [89]. Yan et al. [90] indicated that the size and performance efficiency of UAVs are highly dependent on their application needs. UAVs have growing potential in B5G wireless communications to enable flexible aerial broadband wireless connections, which can also play a significant role in coordinating aerial-ground communications [91]. A big UAV may serve as a HAPs node, while a small UAV can serve as an LAPs node. The use of UAV-assisted correspondence may generally be categorized into two categories: air-to-air communications and air-to-ground communications. UAVs are outfitted with an onboard microprocessor, cameras, wings, a large number of sensors, a GPS, and a battery to offer seamless aerial network coverage [92].
Figure 11: Application of UAVs
Numerous studies have been conducted to highlight the advantages and potential of integrating UAVs into the next wireless networks. The difficulties in channel modeling, propagation loss, and link budget design for UAV-aided communication systems were noted by the authors in [90,91]. The authors of [92] examined the lingering problems with UAVs in civil applications, charging issues, and ways to increase UAV intelligence. It has also been shown how cutting-edge technology, such as an artificial intelligence (AI)-driven machine learning (ML) technique, may be integrated to tackle UAVs’ power backup issues and mount more potent cameras for taking high-resolution photos. The authors of [93] provided examples of how UAV-based networks are used to build the Internet of Things (IoT). By converting analog systems into digital real-time systems with the use of UAVs, particularly in distant geographic areas and for a broad variety of applications, the IoT might bring about substantial changes. According to the authors of [94], a network made up of many UAVs known as “UAV-based swarm networks” may provide a reliable wireless connection and cutting-edge intelligence. It may also be seen as a network that effectively supports the main 5G network services. To satisfy the requirements of B5G heterogeneous wireless networks, the authors in [95] studied the fusion of modern technologies such as NOMA, MIMO, CR, mmWave, etc., in UAV-aided communication networks. Advances in drone-control technologies enable operators with minimum technical experience to easily deploy and operate drones. UAVs fly lower in all directions and maneuver more easily than crewed planes. Security concerns are increased by drones [96].
Intelligent reflecting surface (IRS) is a relatively new wireless communication method that utilizes an array of reflective surfaces that are changed to direct the signal wave towards a particular user or receiver [97,98]. In contrast, relaying is used to transmit data from one source to another through a secondary source [99,100]. IRS is a radical and game-changing technique for achieving future spectrum and energy efficiency in wireless communications. An IRS consists of a large number of low-cost passive components, each of which may independently reflect the incoming signal with a programmable phase shift to achieve three-dimensional passive beamforming without transmitting radio-frequency chains [101,102]. Therefore, IRS installed on a UAV is an effective option for SATCOM and ground users [103–106].
The influence of the achievable rate on optimal transmission power is seen in Fig. 12. In all scenarios, the optimal transmission power tends to grow as the achievable rate increases. A comparison of the performance of the DF relay and IRS approaches in UAVs is shown. When the DF relay approach was used in a UAV, the highest achievable transmission power was
Figure 12: The optimal transmission power is required in order to achieve rate
In Fig. 13, we compare the energy efficiency at different rates that can be reached. The better a system works, the more energy efficient it will be. The DF relay scheme and the IRS method are compared in terms of how well they use energy in UAVs. At a rate of 1 bps/Hz, a UAV built with DF relay saves 3.08105 Kb/J of energy, while a UAV built with IRS saves only 1.98 Kb/J of energy. Using the IRS method, this makes the UAV
Figure 13: Energy efficiency as a function of the achievable rate
In Fig. 14, we show how the optimal transmission power changes with the number of IRS components. When the DF relay system was installed in a UAV, an optimal transmission power of 97.44 kW was measured for all N values. Upon deploying an IRS system on a UAV, a sloping terrain was discovered. The optimum transmission power for the IRS-implemented UAV was 413.5 kW at a N value of 4014. Using an IRS-implemented UAV as opposed to a DF relay-implemented UAV increased power consumption by
Figure 14: The optimal transmission power is required for IRS composed of N elements
Fig. 15 compares the energy efficiency of various IRS scenarios with varying numbers of N components. We compare how well a UAV that uses a DF relay system and one that uses an IRS scheme use energy. A UAV with a DF relay system has a constant energy efficiency of 0.031 Kb/J for all values of N. When the IRS system was put into a UAV, it was found that the energy efficiency got better as the value of N went up. When N is 19230, the energy efficiency of the IRS UAV is 0.033 Kb/J. When the UAV was set up with an IRS system, this made it
Figure 15: Energy efficiency as a function of the IRS composed of N elements
Internet of Remote Things (IoRT) over satellites is enabled by (i) interoperability between satellite systems and sensors/actuators and (ii) IPv6 support over satellites [107]. Interoperability between satellites and sensors/actuators (for example, in an environment such as a smart grid) is one of the fundamental enabling components for IoRT. This environment might gather data from a large number of sensors and deliver it back to the ground station for data management. Again, the control data could be broadcast from ground stations to the satellite, which could then be delivered to the actuator nodes [108]. For IoT to operate as gateways in terms of collecting and transmitting data from IoT sensors, their deployment and mobility patterns might be determined depending on an application’s need to cover a certain region. In such a scenario, authorized users may access the Internet through a 5G network and monitor the frequency of events and the interval at which IoT sensors gather data. This kind of solution may reduce operating expenses [109]. The main reason is that IoRT proved to be more sophisticated than originally anticipated. To begin with, there are several IoRT connection possibilities, but this diversity is more confusing than beneficial. Because of IoRT’s fast expansion, it is confronted with security and privacy challenges [110].
Internet of Space Things (IoST) is expected to be the foundation for a wide range of new transformative applications that go beyond traditional IoT [111,112]. CubeSats in IoST are not just a backhaul network that provides wireless connections; they also do their own active and passive sensing. IoST’s physical architecture is made up of IoST hubs, on-earth and near-earth sensing devices, and the CubeSat network. The IoST hubs discuss to the CubeSats and hold a lot of the control framework for the whole network. The CubeSats, on the other hand, work in the exosphere (above 500 km) and make up the network in space to efficiently receive, send, and relay data. In a more general way, IoST part of the development process can be divided into three groups based on their functionalities: (i) monitoring and reconnaissance, (ii) in-space backhaul, and (iii) cyber-physical integration [111].
In [113], authors combined cloud and space communications to improve information management. The work can aid IoST applications. Software developers and domain specialists define application requirements. Functional and non-functional needs exist. Process management handles applicable requirements, whereas scalability management handles non-functional ones. Monitoring, predictive, adaptive, and autonomic management knowledge patterns comprise process management knowledge. Semantic management involves data scalability and integration. Big data scalability management includes detection, prediction, and process trends. Process management considers manageability, extensibility, and maintainability.
A problem of the standard IoST cloud design is not only the high communication channel load with two-way traffic created by the IoT system but also the delay caused by the IoT information burst transmission via the data channel via routers and other network equipment. Modern SATCOM systems facilitate the data transmission of IoST systems based mostly on cloud technology. IoST systems’ cloud architecture has the problem of requiring the transmission of all information from IoST to cloud computing data centers and vice versa, which causes SATCOM systems to operate inefficiently [114].
For a long time, navigation and positioning have been the primary uses for satellite networks. However, in more recent years, these systems have been put to use in other fields, including smart transportation, environmental monitoring, etc. The use of SATCOMs may be a useful addition to traditional methods of contact on earth. This means that in the future, intelligent transportation systems will rely heavily on satellite-terrestrial networks, which connect satellite networks with terrestrial networks [115,116]. A major problem for satellite-terrestrial networks is the ever-increasing demand for low-delay, high-access communications, coupled with the ever-shrinking bandwidth and network resources available to meet that need. In order to address problems and provide services for vehicles, multi-beam satellites have the ability to produce many spatially separated beams and exhibit very high frequency reuse [117].
There are two facets to the constraints: (i) high propagation delay and (ii) the balance between communication and computation resources is managed, which is crucial in SATCOM. In SATCOM, for instance, the service flow transiting via the space/air network node may dramatically minimize transmission hops, hence conserving communication resources on the ground at the expense of high computer resource consumption in space/air [118].
Smart cities-by integrating information and communication technology (ICT) and IoT, there has been increased progress in recent years in the fields of mobile/wearable computing, IoT, and cloud computing, enabling seamless connection of both the cyber and physical spaces in a city, making the hybrid computing of humans, machines, and smart elements a new trend [119,120]. With initiatives already in place, it is envisioned that context-based sensing can be carried out in smart cities and that data can be gathered in real-time. For instance, users may be presented with situational information on air quality, noise level, light intensity, temperature, wind speed, and other factors. Once again, many forms of infrastructure, such as potholes, broken traffic signals, dangerous structures, work zones, etc., might be supplied to users as information. In addition to these, Hornillo-Mellado et al. [121,122] may inform about city dynamics such as traffic pattern, congestion on roads, flow of people, concert, public gathering, etc. This may make it possible to control and watch over the city and civilization. Urban context actuation, which seeks to impose action or influence in the urban setting, is another component. In essence, it seeks to improve various smart city systems (such as better logistics, intelligent transportation, and so forth) [119].
The goal of a smart city is to gradually transform a city’s complicated internal operations so that they operate more effectively and efficiently. Such a system may be feasible in the near future because of faster data rates made available by modernizing network infrastructures, which were not previously achievable prior to the invention and development of hybrid networks. In order to enable vehicle-to-everything communications, smart healthcare, and smart homes for its smart residents, a smart city requires network resources in large magnitudes. It can use AI to make wise judgments for the more intelligent construction of infrastructure. There would be elements of a smart city in each of them to enable multiple communication paradigms. A hierarchical design of a smart city scenario is shown in Fig. 16. This fundamental need for today’s metropolitan regions is harmoniously supported by the employment of UAVs to assist B5G networks.
Figure 16: The hierarchical architecture of smart city
With the advancement of SATCOM, satellites are getting miniaturized and a large number of satellites are being deployed, which results in congestion [123,124]. To minimize bandwidth congestion, the THz (0.1–10 THz) band is being considered as an alternative to free-space optical communication. THz radiation wavelengths are longer than infrared waves, and so THz waves (wavelengths in the cm range) have less scattering and better penetration depths than infrared ones (range is in
1. Path-loss: Path-loss issues include poor propagation, excessive penetration, blockages and scattering losses, limited coverage ranges, and the necessity for robust transmission directionality [126,127]. This is amplified in the THz band. The path-loss in the THz band is very sensitive to humidity and transmitter-to-receiver distance [128,129]. To circumvent this, non-LoS communication is desired; however, in the case of THz, non-LoS communication is limited to the surface material and second order reflections [130,131]. The deployment of highly directed dynamic massive MIMO antennas is a possible solution.
2. Transceiver design and signal generator: Designing wide-bandwidth THz transceivers is a difficulty. The THz gap indicates the frequency range is too high for traditional oscillators and too low for optical photon emitters. THz transceivers are power- and distance-limited. Silicon germanium (SiGe), gallium nitride (GaN), gallium arsenide (GaAs), and indium phosphide (InP) are prominent THz signal producers and detectors [132]. GaN, GaAs, and InP-based transceivers have limited transmission distances [133]. Novel THz band transceiver architecture is needed [134].
3. Network architecture: THz frequency range is huge, and various carrier frequency windows have distinct transmission distance properties. Overhauling the existing deployed MAC protocols and network deployment strategies is necessary to provide widespread ultra-high-rate access in complicated contexts [135]. The MAC channel coding technique requires further investigation. Current error-detection and error-correction codes perform at these frequencies [136]. Forward error correcting codes will also improve with high path loss and weak diffusion signals.
4. Synchronization: THz communication systems are prone to synchronization issues because they use ultra-short pulses and high-speed analog-to-digital converters (ADCs). Size and energy expenditure prevent most THz nodes from using high-speed ADCs. Furthermore, ADCs require more synchronization effort to attain the THz Nyquist rate [137].
To achieve broader wireless coverage with ultra-fast data rates, SATCOMs are expected to be integrated with future wireless technologies. Massive-MIMO has been recently deployed in 5G networks and has the potential to be used in LEO satellite [138,139]. mmWave-massive MIMO is a technology that can potentially integrate the high available mmWave bandwidth with the high gains from massive MIMO antenna arrays. Utilizing mmWave-massive MIMO in SATCOM, next generation satellite networks can be proposed to benefit from extreme infrastructure densification (small cells). The combination of large quantities of new bandwidth with many antennas on a very large scale can bring a plethora of high-speed services to feed high bandwidth applications. Using mmWave-massive MIMO raises difficulties such as energy efficiency, channel and field measurements, testing, and system performance validation. In addition, the areas of modulation, signal waveform, multiple access schemes, front haul design, antenna array architecture, and precoding are being proposed for mmWave-massive MIMO systems [140,141]. An integrated performance evaluation and characterization in real-life situations and applications remain an open question [142,143]. Massive MIMO technology raises major concerns that must be resolved.
1. Hardware impairment: Massive MIMO uses several antennas to decrease noise, fading, and interference. In massive MIMO, multiple antennas increase complexity and hardware costs. Massive MIMO needs low-cost, compact components to decrease computational complexity and hardware size [144].
2. Channel estimation: Channel estimate becomes more difficult as the number of antennas on both ends of the communication connection rises [145,146].
3. Training symbols: The number of transmitting antennas affects the training symbols for channel estimation. Throughput decreases as the number of training symbols increases [146].
4. Interference: With more spatial antennas, loop interference becomes worse [146].
The benefits of SATCOM include service continuity over uncovered and under-covered areas, service ubiquity, and service scalability [147–149]. However, because satellite networks have greater obstacles than terrestrial networks in terms of resource management, network control, network security, spectrum management, and energy utilization; various challenges must be overcome before these benefits can be realized. During this time, artificial intelligence has been steadily growing as a research field, which includes machine learning (ML), deep learning (DL), and reinforcement learning [150–153]. In [154], authors described the introduction of ML-based mechanisms into satellite network operation centers, such as interference detection, configuration of flexible payloads, and congestion prediction. The interference detection method requires raw in-phase and in-quadrature samples of a transponder, while the congestion prediction method is based on historical user demand data. Additionally, flexible payload configuration takes into account both the theoretical model of the payload and certain rate requirements over the coverage area. The proposed ML-based techniques perform well numerically, as evidenced by the numerical results: the interference detector reduces false detection probability by 44%, the flexible payload optimizer reduces unmet capacity by 32%, and the traffic predictor reduces prediction error by 10% when compared to other approaches. As a result, ML-based SATCOM will be a viable option in the future. However, there are certain difficult implementation challenges in SATCOM.
1. Algorithm: Once a SATCOM issue is identified, the goal is to find viable ML algorithms for comparable challenges in other domains (i.e., computer vision, medical applications, etc.) and adapt them to the specified problem [154].
2. AI chipset: Implementing ML approaches in SATCOM systems requires selecting the best AI chip design to fulfill service needs [155].
3. Multibeam satellite system: Some spot beams have a larger demand than the available capacity, leaving the demand waiting, whereas others have a lesser demand, leaving the supplied capacity unused. Flexible on-board distribution of satellite resources throughout the service coverage region is needed to enhance multi-beam SATCOM [147,154].
4. Computing problem: AI processing power consumption nearly follows Moore’s Law as clock speed increases. The multi-core era speeds up Moore’s Law when DL has arrived. AI’s requirement for brute-force processing boosts power usage by
5. Disappearing gradient: Backward-propagation gradient iteration in DL is a problem for hardware acceleration design, affecting wireless transmission latency and end-to-end communications [156].
The frequency availability in LEO mobile satellite systems is investigated in [157], and a unique wideband spectrum compressed signal detection technique is presented to collect active primary users’ sub-bands and their locations to avoid during frequency allocation. In addition, the authors proposed a new wideband spectrum compressed sensing (CS) approach based on the discrete sine transform, which greatly enhances spectrum detection and recovery accuracy when compared to a traditional discrete Fourier transform-based wideband spectrum CS system. Moreover, the architecture of several satellite cooperative sensing according to OR and MAJ decision fusion rules is described using inter-satellite connections to provide spatial diversity against wireless fading. However, CS-based sampling presents various practical challenges.
1. Sampling issue: The L/S frequency availability is critical for global communication in the LEO mobile satellite network, but it has several major technical challenges, including a high sampling rate for wideband sensing, limited power and computing resources for processing load, and frequency-selective wireless fading [158,159].
2. Sampling circuit: To implement the CS framework in reality, CS sampling circuits (also known as CS samplers) are required. Typically, these samplers operate at sub-Nyquist rates, freeing up the ADC’s operating speed [160].
3. Time-consuming: This application is time-consuming and restricted by the number of sensors used [160].
Within the realm of quantum technology, satellite quantum communications are developing as a more effective technique for distributing entirely secure keys across great distances, thereby playing a significant part in the construction of a large-scale quantum network [161–164]. In [165], the author used recent achievements in free-space quantum communications to calculate the ultimate limits of secret bits that may be distributed through satellites. This research covers a wide range of actual scenarios, including downlink and uplink setups, as well as satellites at varying altitudes and zenith angles. There are effects of diffraction, extinction, and background noise, as well as fading due to point-of-view errors and atmospheric turbulence. In addition, the author presents the configurable finite-size secret key rates that protocols with continuous variable quantum key distribution (QKD) may achieve for both downlink and uplink, demonstrating that this method is feasible in all situations. Moreover, the author shows that a sun-synchronous satellite’s QKD rate can surpass a terrestrial chain of perfect quantum repeaters in a simulation. Quantum communication will enable new applications such as ultra-secure communication and a new generation of high-performance computers in the future, thanks to the unique qualities of quantum entanglement [166–168].
Many difficulties that classical communication networks face can be solved with quantum computing, but the knowledge gap between the two has yet to be addressed [169]. The authors presented cloud-based cellular networks (cloud radio access networks (C-RAN)) directions, analyses, and prospective quantum solutions. This study examined the impact of quantum entanglement phenomena on a traditional cloud network, focusing on the use of an entanglement theory to reduce the traditional cloud network’s increased signaling cost delay. This study promises a delay reduction when using a quantum approach in wireless cloud networks by modeling the latency of both paradigms. The time delay for varying numbers of entangled photons is shown in Fig. 17. It is worth noting that as the number of entangled photons grows, so does the time delay. We can see from the graph that quantum entanglement-based C-RAN has a shorter latency than traditional C-RAN. Despite notable advancements over the last several decades, there are still considerable obstacles facing large-scale SATCOM networks.
Figure 17: Time delays between classical C-RAN and quantum entanglement based C-RAN compared for various number of entangled photons
1. System performance: Satellite QKD is more complicated than terrestrial. Satellite overpasses limit transmission duration and cause varying channel losses inside and between passes. The achievable secret key is limited by the reduced transmission time [170,171].
2. Security: Future quantum computers might break asymmetric encryption, threatening our crucial infrastructure in space. QKD offers a long-term and secure solution to quantum computer threats [172].
3. Impact of the atmosphere: A quantum signal passing through the earth’s atmosphere is influenced by turbulence and background noise throughout the day. Both have an impact on key and entanglement distribution rates [171].
4. Synchronization: Satellite QKD requires correlated signal transmission and reception. Long distances, relative motion, and arrival uncertainty are problems. High loss complicates issues. Dispersion is not an issue for vacuum propagation, but the atmosphere may have only a minor impact on space-ground communications, according to [171].
5. Device: QKD is perfectly safe only when it uses single-photon sources and detectors. In real life, there are never any perfect devices. So, flaws in the device can lead to security holes or side channels that can make practical QKD less secure [173].
This study talks about the importance and future of low altitude small satellite aerial vehicles, which are used to make sure that communications between the air and the ground go smoothly for the B5G networks. To reach the general goals of 5G and later wireless networks, the current architecture of aerial networks needs to be looked at again. This study shows the detailed architecture of low altitude aerial networks and the challenges of managing resources. We have also looked at how promising communication technologies and low altitude aerial networks can work together to provide strong network coverage. We talk about the ways that low altitude aerial networks can be controlled and monitored in a way that is easy for users, so that wireless broadband connections can be taken to a new level. We conclude by outlining several promising avenues for further study in aerial-ground communications, including THz, massive MIMO, ML, compressed sensing, and quantum communications.
Funding Statement: The authors received no specific funding for this study.
Conflicts of Interest: The authors declare that they have no conflicts of interest to report regarding the present study.
References
1. Popovski, P., Trillingsgaard, K. F., Simeone, O., Durisi, G. (2018). 5G wireless network slicing for eMBB, URLLC, and mMTC: A communication-theoretic view. IEEE Access, 6, 55765–55779. [Google Scholar]
2. Sabuj, S. R., Khan, M. R. H., Ziad, M. O. (2020). An analytical investigation of finite blocklength information theory. 2020 IEEE Region 10 Symposium (TENSYMP), Dhaka, Bangladesh, IEEE. [Google Scholar]
3. Guo, S., Lu, B., Wen, M., Dang, S., Saeed, N. (2022). Customized 5G and beyond private networks with integrated URLLC, eMBB, mMTC, and positioning for industrial verticals. IEEE Communications Standards Magazine, 6(1), 52–57. [Google Scholar]
4. Ge, C., Wang, N., Selinis, I., Cahill, J., Kavanagh, M. et al. (2019). QoE-assured live streaming via satellite backhaul in 5G networks. IEEE Transactions on Broadcasting, 65(2), 381–391. [Google Scholar]
5. Hu, M., Yang, R., Hu, Y., Cai, C., Dong, Y. et al. (2022). QoE-aware software defined multicast in LEO satellite networks. IEEE Transactions on Aerospace and Electronic Systems. DOI 10.1109/TAES.2022.3169732. [Google Scholar] [CrossRef]
6. Sumona, A. S., Kundu, M. K., Badrudduza, A. (2022). Security analysis in multicasting over shadowed rician and α-μ fading channels: A dual-hop hybrid satellite terrestrial relaying network. IET Communications, 16(1), 43–57. [Google Scholar]
7. Bai, L., Zhu, L., Zhang, X., Zhang, W., Yu, Q. (2018). Multi-satellite relay transmission in 5G: Concepts, techniques, and challenges. IEEE Network, 32(5), 38–44. DOI 10.1109/MNET.2018.1800038. [Google Scholar] [CrossRef]
8. Cioni, S., de Gaudenzi, R., Herrero, O. D. R., Girault, N. (2018). On the satellite role in the era of 5G massive machine type communications. IEEE Network, 32(5), 54–61. DOI 10.1109/MNET.2018.1800024. [Google Scholar] [CrossRef]
9. Lin, X., Hofström, B., Wang, Y. P. E., Masini, G., Maattanen, H. L. et al. (2021). 5G new radio evolution meets satellite communications: Opportunities, challenges, and solutions. In: 5G and beyond, pp. 517–531. Springer. https://link.springer.com/chapter/10.1007/978-3-030-58197-8_18. [Google Scholar]
10. Giambene, G., Kota, S., Pillai, P. (2018). Satellite-5G integration: A network perspective. IEEE Network, 32(5), 25–31. DOI 10.1109/MNET.2018.1800037. [Google Scholar] [CrossRef]
11. Boero, L., Bruschi, R., Davoli, F., Marchese, M., Patrone, F. (2018). Satellite networking integration in the 5G ecosystem: Research trends and open challenges. IEEE Network, 32(5), 9–15. DOI 10.1109/MNET.2018.1800052. [Google Scholar] [CrossRef]
12. Di, B., Song, L., Li, Y., Poor, H. V. (2019). Ultra-dense LEO: Integration of satellite access networks into 5G and beyond. IEEE Wireless Communications, 26(2), 62–69. DOI 10.1109/MWC.2019.1800301. [Google Scholar] [CrossRef]
13. Kodheli, O., Lagunas, E., Maturo, N., Sharma, S. K., Shankar, B. et al. (2021). Satellite communications in the new space era: A survey and future challenges. IEEE Communications Surveys & Tutorials, 23(1), 70–109. DOI 10.1109/COMST.2020.3028247. [Google Scholar] [CrossRef]
14. Leyva-Mayorga, I., Soret, B., Röper, M., Wübben, D., Matthiesen, B. et al. (2020). LEO small-satellite constellations for 5G and beyond-5G communications. IEEE Access, 8, 184955–184964. DOI 10.1109/ACCESS.2020.3029620. [Google Scholar] [CrossRef]
15. Lalbakhsh, A., Pitcairn, A., Mandal, K., Alibakhshikenari, M., Esselle, K. P. et al. (2022). Darkening low-earth orbit satellite constellations: A review. IEEE Access, 10, 24383–24394. DOI 10.1109/ACCESS.2022.3155193. [Google Scholar] [CrossRef]
Pritchard-Kelly, R., Costa, J. (2022). Low earth orbit satellite systems: Comparisons with geostationary and other satellite systems, and their significant advantages. Journal of Telecommunications and the Digital Economy, 10(1), 1–22. DOI 10.18080/jtde.v10n1.552. [Google Scholar] [CrossRef]
17. de Gaudenzi, R., Luise, M., Sanguinetti, L. (2022). The open challenge of integrating satellites into (beyond-) 5G cellular networks. IEEE Network, 36(2), 168–174. DOI 10.1109/MNET.011.2100116. [Google Scholar] [CrossRef]
18. Darwish, T., Kurt, G. K., Yanikomeroglu, H., Bellemare, M., Lamontagne, G. (2022). LEO satellites in 5G and beyond networks: A review from a standardization perspective. IEEE Access, 10, 35040–35060. DOI 10.1109/ACCESS.2022.3162243. [Google Scholar] [CrossRef]
19. Rodrigues, T. K., Kato, N. (2022). Network slicing with centralized and distributed reinforcement learning for combined satellite/ground networks in a 6G environment. IEEE Wireless Communications, 29(1), 104–110. DOI 10.1109/MWC.001.2100287. [Google Scholar] [CrossRef]
20. Liu, H., Zhang, H., Yang, K., Li, J. (2022). Virtualized high throughput satellite gateway with a global bandwidth management method. Mobile Information Systems, 2022, 1–11. DOI 10.1155/2022/6257885. [Google Scholar] [CrossRef]
21. Sheng, M., Wang, Y., Li, J., Liu, R., Zhou, D. et al. (2017). Toward a flexible and reconfigurable broadband satellite network: Resource management architecture and strategies. IEEE Wireless Communications, 24(4), 127–133. DOI 10.1109/MWC.2017.1600173. [Google Scholar] [CrossRef]
22. Dong, F., Li, H., Gong, X., Liu, Q., Wang, J. (2015). Energy-efficient transmissions for remote wireless sensor networks: An integrated HAP/satellite architecture for emergency scenarios. Sensors, 15(9), 22266–22290. DOI 10.3390/s150922266. [Google Scholar] [CrossRef]
23. Sadique, J. J., Ullah, S. E., Islam, M. R., Raad, R., Kouzani, A. Z. et al. (2021). Transceiver design for full-duplex UAV based zero-padded OFDM system with physical layer security. IEEE Access, 9, 59432–59445. DOI 10.1109/ACCESS.2021.3073488. [Google Scholar] [CrossRef]
24. Oishi, A. T., Rahman, L. F., Siddika, M. J., Arnab, S., Sabuj, S. R. (2021). Application of unmanned aerial vehicles in wireless networks: Mobile edge computing and caching. In: Secure edge computing, pp. 201–218. CRC Press. [Google Scholar]
25. Al-Farabi, M., Chowdhury, M., Readuzzaman, M., Hossain, M., Sabuj, S. R. et al. (2020). Smart environment monitoring system using unmanned aerial vehicle in bangladesh. EAI Endorsed Transactions on Smart Cities, 5(14), 1–10. DOI 10.4108/eai.18-8-2020.165995. [Google Scholar] [CrossRef]
26. Dong, F., Han, H., Gong, X., Wang, J., Li, H. (2016). A constellation design methodology based on QoS and user demand in high-altitude platform broadband networks. IEEE Transactions on Multimedia, 18(12), 2384–2397. DOI 10.1109/TMM.2016.2595260. [Google Scholar] [CrossRef]
27. Matsushita, Y., Yoshimura, Y., Hanada, T., Itaya, Y., Fukushima, T. (2022). Risk assessment of a large constellation of satellites in low-earth orbit. Transactions of the Japan Society for Aeronautical and Space Sciences, Aerospace Technology Japan, 20, 10–15. DOI 10.2322/tastj.20.10. [Google Scholar] [CrossRef]
28. Liang, X., Niu, H., Liu, A., Gao, Z., Zhang, Y. (2022). Joint STO and DFO estimation for sefdm in low-earth-orbit satellite communications. IEEE Transactions on Aerospace and Electronic Systems, 58(4), 3725–3729. DOI 10.1109/TAES.2021.3140186. [Google Scholar] [CrossRef]
29. Deng, R., Di, B., Zhang, H., Kuang, L., Song, L. (2021). Ultra-dense LEO satellite constellations: How many LEO satellites do we need? IEEE Transactions on Wireless Communications, 20(8), 4843–4857. DOI 10.1109/TWC.2021.3062658. [Google Scholar] [CrossRef]
30. Arum, S. C., Grace, D., Mitchell, P. D. (2022). Delivering extended cellular coverage and capacity using high-altitude platforms. Electronics, 11(9), 1508. DOI 10.3390/electronics11091508. [Google Scholar] [CrossRef]
31. Arif, M., Kim, W., Haroon, M. S. (2022). Coverage probability of aerial heterogeneous networks disrupting dual connectivity in the presence of jammers. AEU-International Journal of Electronics and Communications, 145(5), 154073. DOI 10.1016/j.aeue.2021.154073. [Google Scholar] [CrossRef]
32. Li, Z., Yan, Y., Xia, X., Xia, L., Meng, D. et al. (2022). A survey of coverage issues in UAV networks. 2022 24th International Conference on Advanced Communication Technology (ICACT), PyeongChang Kwangwoon_Do, South Korea, IEEE. [Google Scholar]
33. Al-Hourani, A., Kandeepan, S., Jamalipour, A. (2014). Modeling air-to-ground path loss for low altitude platforms in urban environments. 2014 IEEE Global Communications Conference, Austin, TX, USA, IEEE. [Google Scholar]
34. Richharia, M. (2017). Satellite communication systems: Design principles. London, UK: Macmillan International Higher Education. [Google Scholar]
35. Welti, C. R. (2012). Satellite basics for everyone: An illustrated guide to satellites for non-technical and technical people. Bloomington, Indiana, USA: iUniverse. [Google Scholar]
36. Jo, K. Y. (2011). Satellite communications network design and analysis. Norwood, Massachusetts, USA: Artech House. [Google Scholar]
37. Lu, F., Jiang, Y., Wang, R., Wang, H., Zhao, P. et al. (2022). System demonstrations of Ka-band 5-Gbps data transmission for satellite applications. International Journal of Satellite Communications and Networking, 40(3), 204–217. DOI 10.1002/sat.1434. [Google Scholar] [CrossRef]
38. Leng, T., Duan, P., Hu, D., Cui, G., Wang, W. (2022). Cooperative user association and resource allocation for task offloading in hybrid GEO-LEO satellite networks. International Journal of Satellite Communications and Networking, 40(3), 230–243. DOI 10.1002/sat.1436. [Google Scholar] [CrossRef]
39. Kumar, U., Garg, M. (2022). A note on an enhanced dynamic authentication scheme for mobile satellite communication systems. International Journal of Satellite Communications and Networking, 40(5), 317–329. DOI 10.1002/sat.1443. [Google Scholar] [CrossRef]
40. Tong, J., Wang, C., Zhao, X., Cui, G., Wang, W. (2022). Cooperative multigroup multicast beamforming for cache-enabled ultra-dense low earth orbit satellite constellation networks. International Journal of Satellite Communications and Networking, 40(5), 343–356. DOI 10.1002/sat.1445. [Google Scholar] [CrossRef]
41. Öztürk, F., Kara, A. (2022). Exclusion zone minimization and optimal operational mode selection for co-existent geostationary and non-geostationary satellites. International Journal of Satellite Communications and Networking, 40(3), 191–203. DOI 10.1002/sat.1433. [Google Scholar] [CrossRef]
42. Guo, Y., Kong, H., Huang, Q., Lin, M., Zhu, W. P. et al. (2021). Performance analysis for the forward link of multiuser satellite communication systems. International Journal of Satellite Communications and Networking, 39(5), 560–569. DOI 10.1002/sat.1401. [Google Scholar] [CrossRef]
43. Lagunas, E., Tsinos, C. G., Sharma, S. K., Chatzinotas, S. (2020). 5G cellular and fixed satellite service spectrum coexistence in C-band. IEEE Access, 8, 72078–72094. DOI 10.1109/ACCESS.2020.2985012. [Google Scholar] [CrossRef]
44. Rikkinen, K., Kyosti, P., Leinonen, M. E., Berg, M., Parssinen, A. (2020). THz radio communication: Link budget analysis toward 6G. IEEE Communications Magazine, 58(11), 22–27. DOI 10.1109/MCOM.001.2000310. [Google Scholar] [CrossRef]
45. Wu, Y., Hu, G., Jin, F., Zu, J. (2019). A satellite handover strategy based on the potential game in LEO satellite networks. IEEE Access, 7, 133641–133652. DOI 10.1109/ACCESS.2019.2941217. [Google Scholar] [CrossRef]
46. Wu, Z., Jin, F., Luo, J., Fu, Y., Shan, J. et al. (2016). A graph-based satellite handover framework for LEO satellite communication networks. IEEE Communications Letters, 20(8), 1547–1550. DOI 10.1109/LCOMM.2016.2569099. [Google Scholar] [CrossRef]
47. Perez-Neira, A. I., Vazquez, M. A., Shankar, M. B., Maleki, S., Chatzinotas, S. (2019). Signal processing for high-throughput satellites: Challenges in new interference-limited scenarios. IEEE Signal Processing Magazine, 36(4), 112–131. DOI 10.1109/MSP.2019.2894391. [Google Scholar] [CrossRef]
48. Wang, P., Zhang, J., Zhang, X., Yan, Z., Evans, B. G. et al. (2019). Convergence of satellite and terrestrial networks: A comprehensive survey. IEEE Access, 8, 5550–5588. DOI 10.1109/ACCESS.2019.2963223. [Google Scholar] [CrossRef]
49. Chuan, L. S., Sun, R. T., Hon, Y. P. (2015). Ka band satellite communications design analysis and optimization. In: Defense, Science and Technology Agency (DSTA) horizons, pp. 70–78. Singapore: Defence, Science & Technology Agency. [Google Scholar]
50. Cho, Y., Kim, H., Tettey, D. K., Lee, K. J., Jo, H. S. (2019). Modeling method for interference analysis between IMT-2020 and satellite in the mmWave band. 2019 IEEE Globecom Workshops (GC Wkshps), Waikoloa, HI, USA, IEEE. [Google Scholar]
51. Cho, Y., Kim, H. K., Nekovee, M., Jo, H. S. (2020). Coexistence of 5G with satellite services in the millimeter-wave band. IEEE Access, 8, 163618–163636. DOI 10.1109/ACCESS.2020.3022044. [Google Scholar] [CrossRef]
52. Cho, Y., Kim, H. K., Jo, H. S. (2019). Coexistence between 5G mobile communication and fixed-satellite service in the millimeter wave band. The Journal of Korean Institute of Electromagnetic Engineering and Science, 30(9), 742–753. DOI 10.5515/KJKIEES.2019.30.9.742. [Google Scholar] [CrossRef]
53. Polese, M., Jornet, J. M., Melodia, T., Zorzi, M. (2020). Toward end-to-end, full-stack 6G terahertz networks. IEEE Communications Magazine, 58(11), 48–54. DOI 10.1109/MCOM.001.2000224. [Google Scholar] [CrossRef]
54. Sabuj, S. R., Rubaiat, M., Iqbal, M., Mobashera, M., Malik, A. et al. (2022). Machine-type communications in noma-based terahertz wireless networks. International Journal of Intelligent Networks, 3(3), 31–47. DOI 10.1016/j.ijin.2022.04.002. [Google Scholar] [CrossRef]
55. Sabuj, S. R., Khan, A. M. S., Hamamura, M. (2020). Application of non-orthogonal multiple access for machine type communication in sub-terahertz band. Computer Networks, 182(3), 107508. DOI 10.1016/j.comnet.2020.107508. [Google Scholar] [CrossRef]
56. Tultul, N. A., Farha, S., Hossain, S. S., Hossain, M. A., Sabuj, S. R. (2020). Device-to-device communication in terahertz frequency band: Enhancement of energy efficiency. 2020 IEEE Region 10 Conference (TENCON), Osaka, Japan, IEEE. [Google Scholar]
57. Tarboush, S., Sarieddeen, H., Chen, H., Loukil, M. H., Jemaa, H. et al. (2021). Teramimo: A channel simulator for wideband ultra-massive MIMO terahertz communications. IEEE Transactions on Vehicular Technology, 70(12), 12325–12341. DOI 10.1109/TVT.2021.3123131. [Google Scholar] [CrossRef]
58. Tan, J., Dai, L. (2021). Wideband beam tracking in thz massive MIMO systems. IEEE Journal on Selected Areas in Communications, 39(6), 1693–1710. DOI 10.1109/JSAC.2021.3071817. [Google Scholar] [CrossRef]
59. Greda, L. A., Dreher, A. (2007). Tx-terminal phased array for satellite communication at Ka-band. 2007 European Microwave Conference, Munich, Germany, IEEE. [Google Scholar]
60. Lin, Z., Lin, M., Wang, J. B., Huang, Y., Zhu, W. P. (2018). Robust secure beamforming for 5G cellular networks coexisting with satellite networks. IEEE Journal on Selected Areas in Communications, 36(4), 932–945. DOI 10.1109/JSAC.2018.2824760. [Google Scholar] [CrossRef]
61. Hassan, S. S., Kim, D. H., Tun, Y. K., Tran, N. H., Saad, W. et al. (2022). Seamless and energy efficient maritime coverage in coordinated 6G space-air-sea non-terrestrial networks. arXiv preprint arXiv:2201.08605. [Google Scholar]
62. Guidotti, A., Amatetti, C., Arnal, F., Chamaillard, B., Vanelli-Coralli, A. (2022). Location-assisted precoding in 5G LEO systems: Architectures and performances. 2022 Joint European Conference on Networks and Communications & 6G Summit (EuCNC/6G Summit), Grenoble, France. [Google Scholar]
63. Li, W., Law, C. L., Ong, J. T., Dubey, V. K. (2001). Ka-band lms channel model with rain attenuation and other atmospheric impairments in equatorial zone. IEICE Transactions on Communications, 84(12), 3265–3273. [Google Scholar]
64. Samad, M. A., Diba, F. D., Choi, D. Y. (2021). A survey of rain fade models for earth-space telecommunication links-taxonomy, methods, and comparative study. Remote Sensing, 13(10), 1965. DOI 10.3390/rs13101965. [Google Scholar] [CrossRef]
65. Ahmad, A., Cheema, A. A., Finlay, D. (2020). A survey of radio propagation channel modelling for low altitude flying base stations. Computer Networks, 171, 107122. DOI 10.1016/j.comnet.2020.107122. [Google Scholar] [CrossRef]
66. Huang, J., Cao, Y., Raimundo, X., Cheema, A., Salous, S. (2019). Rain statistics investigation and rain attenuation modeling for millimeter wave short-range fixed links. IEEE Access, 7, 156110–156120. DOI 10.1109/ACCESS.2019.2949437. [Google Scholar] [CrossRef]
67. Schneider, M., Hartwanger, C., Wolf, H. (2011). Antennas for multiple spot beam satellites. CEAS Space Journal, 2(1), 59–66. DOI 10.1007/s12567-011-0012-z. [Google Scholar] [CrossRef]
68. Lee, Y., Choi, J. P. (2019). Performance evaluation of high-frequency mobile satellite communications. IEEE Access, 7, 49077–49087. DOI 10.1109/ACCESS.2019.2909885. [Google Scholar] [CrossRef]
69. Guidotti, A., Vanelli-Coralli, A., Foggi, T., Colavolpe, G., Caus, M. et al. (2019). LTE-based satellite communications in LEO mega-constellations. International Journal of Satellite Communications and Networking, 37(4), 316–330. DOI 10.1002/sat.1258. [Google Scholar] [CrossRef]
70. Guidotti, A., Vanelli-Coralli, A., Conti, M., Andrenacci, S., Chatzinotas, S. et al. (2019). Architectures and key technical challenges for 5G systems incorporating satellites. IEEE Transactions on Vehicular Technology, 68(3), 2624–2639. DOI 10.1109/TVT.2019.2895263. [Google Scholar] [CrossRef]
71. Gao, Z., Liu, A., Liang, X. (2020). The performance analysis of downlink noma in LEO satellite communication system. IEEE Access, 8, 93723–93732. DOI 10.1109/ACCESS.2020.2995261. [Google Scholar] [CrossRef]
72. An, K., Yan, X., Liang, T., Lu, W. (2019). Noma based satellite communication networks: Architectures, techniques and challenges. 2019 IEEE 19th International Conference on Communication Technology (ICCT), Xi’an, China, IEEE. [Google Scholar]
73. Arcidiacono, A., Finocchiaro, D., de Gaudenzi, R., del Rio-Herrero, O., Cioni, S. et al. (2021). Is satellite ahead of terrestrial in deploying NOMA for massive machine-type communications? Sensors, 21(13), 4290. DOI 10.3390/s21134290. [Google Scholar] [CrossRef]
74. Mao, Y., Dizdar, O., Clerckx, B., Schober, R., Popovski, P. et al. (2022). Rate-splitting multiple access: Fundamentals, survey, and future research trends. IEEE Communications Surveys & Tutorials, 1. DOI 10.1109/COMST.2022.3191937. [Google Scholar] [CrossRef]
75. Mao, Y., Clerckx, B., Li, V. O. (2018). Rate-splitting multiple access for downlink communication systems: Bridging, generalizing, and outperforming SDMA and NOMA. EURASIP Journal on Wireless Communications and Networking, 2018(1), 1–54. DOI 10.1186/s13638-018-1104-7. [Google Scholar] [CrossRef]
76. Si, Z. W., Yin, L., Clerckx, B. (2022). Rate-splitting multiple access for multigateway multibeam satellite systems with feeder link interference. IEEE Transactions on Communications, 70(3), 2147–2162. DOI 10.1109/TCOMM.2022.3144487. [Google Scholar] [CrossRef]
77. Yan, C., Yuan, Z., Li, W., Yuan, Y. (2019). Non-orthogonal multiple access schemes for 5G. ZTE Communications, 14(4), 11–16. [Google Scholar]
78. Yin, S., Zhao, Y., Li, L., Yu, F. R. (2020). UAV-assisted cooperative communications with time-sharing information and power transfer. IEEE Transactions on Vehicular Technology, 69(2), 1554–1567. DOI 10.1109/TVT.2019.2956167. [Google Scholar] [CrossRef]
79. Gevaert, C. M., Sliuzas, R., Persello, C., Vosselman, G. (2018). Evaluating the societal impact of using drones to support urban upgrading projects. ISPRS International Journal of Geo-Information, 7(3), 91. DOI 10.3390/ijgi7030091. [Google Scholar] [CrossRef]
80. Jia, D., Jia, J., Li, G. (2020). Threshold forwarding protocol of cooperative LEO satellites communication system. 2020 IEEE 20th International Conference on Communication Technology (ICCT), Nanning, China, IEEE. [Google Scholar]
81. Khan, M., Rahman, M. T., Sabuj, S. R. (2018). A transmit antenna selection technique in random cognitive radio network. TENCON 2018-2018 IEEE Region 10 Conference, Jeju, South Korea, IEEE. [Google Scholar]
82. Dias Santana, G. M., Cristo, R. S. D., Lucas Jaquie Castelo Branco, K. R. (2021). Integrating cognitive radio with unmanned aerial vehicles: An overview. Sensors, 21(3), 830. DOI 10.3390/s21030830. [Google Scholar] [CrossRef]
83. Sadique, J. J., Sabuj, S. R., Ullah, S. E., Joarder, S. K., Hamamura, M. (2022). UAV-aided transceiver design for secure downlink OW-DFTs-OFDM: A multi-user mmwave application. IEEE Access, 10, 34577–34590. DOI 10.1109/ACCESS.2022.3162628. [Google Scholar] [CrossRef]
84. Sabuj, S. R., Ahmed, A., Cho, Y., Lee, K. J., Jo, H. S. (2021). Cognitive UAV-aided URLLC and MMTC services: Analyzing energy efficiency and latency. IEEE Access, 9, 5011–5027. DOI 10.1109/ACCESS.2020.3048436. [Google Scholar] [CrossRef]
85. Sabuj, S. R., Asiedu, D. K. P., Lee, K. J., Jo, H. S. (2022). Delay optimization in mobile edge computing: Cognitive UAV-assisted EMBB and MMTC services. IEEE Transactions on Cognitive Communications and Networking, 8(2), 1019–1033. DOI 10.1109/TCCN.2022.3149089. [Google Scholar] [CrossRef]
86. Rahul, A. R., Sabuj, S. R., Akbar, M., Jo, H. S., Hossain, M. A. et al. (2021). An optimization based approach to enhance the throughput and energy efficiency for cognitive unmanned aerial vehicle networks. Wireless Networks, 27(1), 475–493. DOI 10.1007/s11276-020-02450-9. [Google Scholar] [CrossRef]
87. Hu, H., Da, X., Huang, Y., Zhang, H., Ni, L. et al. (2019). Se and EE optimization for cognitive UAV network based on location information. IEEE Access, 7, 162115–162126. DOI 10.1109/ACCESS.2019.2951702. [Google Scholar] [CrossRef]
88. Fotouhi, A., Qiang, H., Ding, M., Hassan, M., Giordano, L. G. et al. (2019). Survey on UAV cellular communications: Practical aspects, standardization advancements, regulation, and security challenges. IEEE Communications Surveys & Tutorials, 21(4), 3417–3442. DOI 10.1109/COMST.2019.2906228. [Google Scholar] [CrossRef]
89. Ahmed, S., Chowdhury, M. Z., Sabuj, S. R., Alam, M. I., Jang, Y. M. (2021). Energy-efficient uav relaying robust resource allocation in uncertain adversarial networks. IEEE Access, 9, 59920–59934. DOI 10.1109/ACCESS.2021.3073015. [Google Scholar] [CrossRef]
90. Yan, C., Fu, L., Zhang, J., Wang, J. (2019). A comprehensive survey on UAV communication channel modeling. IEEE Access, 7, 107769–107792. DOI 10.1109/ACCESS.2019.2933173. [Google Scholar] [CrossRef]
91. Khawaja, W., Guvenc, I., Matolak, D. W., Fiebig, U. C., Schneckenburger, N. (2019). A survey of air-to-ground propagation channel modeling for unmanned aerial vehicles. IEEE Communications Surveys & Tutorials, 21(3), 2361–2391. DOI 10.1109/COMST.2019.2915069. [Google Scholar] [CrossRef]
92. Shakhatreh, H., Sawalmeh, A. H., Al-Fuqaha, A., Dou, Z., Almaita, E. et al. (2019). Unmanned aerial vehicles (UAVsA survey on civil applications and key research challenges. IEEE Access, 7, 48572–48634. DOI 10.1109/ACCESS.2019.2909530. [Google Scholar] [CrossRef]
93. Motlagh, N. H., Taleb, T., Arouk, O. (2016). Low-altitude unmanned aerial vehicles-based Internet of Things services: Comprehensive survey and future perspectives. IEEE Internet of Things Journal, 3(6), 899–922. DOI 10.1109/JIOT.2016.2612119. [Google Scholar] [CrossRef]
94. Li, B., Fei, Z., Zhang, Y. (2018). UAV communications for 5G and beyond: Recent advances and future trends. IEEE Internet of Things Journal, 6(2), 2241–2263. DOI 10.1109/JIOT.2018.2887086. [Google Scholar] [CrossRef]
95. Agiwal, M., Roy, A., Saxena, N. (2016). Next generation 5G wireless networks: A comprehensive survey. IEEE Communications Surveys & Tutorials, 18(3), 1617–1655. DOI 10.1109/COMST.2016.2532458. [Google Scholar] [CrossRef]
96. Ray, P. P. (2022). A review on 6G for space-air-ground integrated network: Key enablers, open challenges, and future direction. Journal of King Saud University-Computer and Information Sciences, 34(9), 6949–6976. [Google Scholar]
97. Björnson, E., Özdogan, Ö., Larsson, E. G. (2020). Reconfigurable intelligent surfaces: Three myths and two critical questions. IEEE Communications Magazine, 58(12), 90–96. DOI 10.1109/MCOM.001.2000407. [Google Scholar] [CrossRef]
98. Björnson, E., Özdogan, Ö., Larsson, E. G. (2019). Intelligent reflecting surface versus decode-and-forward: How large surfaces are needed to beat relaying? IEEE Wireless Communications Letters, 9(2), 244–248. DOI 10.1109/LWC.2019.2950624. [Google Scholar] [CrossRef]
99. Sabuj, S., Das, S., Hossain, M. J. (2014). Performance analysis of ofdm based cooperative communication over nakagami fading channel. International Journal of Hybrid Information Technology, 7(4), 321–330. DOI 10.14257/ijhit.2014.7.4.27. [Google Scholar] [CrossRef]
100. Chisty, K., Islam, S., Ullah, S., Sabuj, S. (2014). Scrambled voice frequency signal transmission in an amplify and forward relaying based STBC encoded cooperative MIMO-OFDM system. International Journal of Signal Processing, Image Processing and Pattern Recognition, 7(2), 143–152. DOI 10.14257/ijsip.2014.7.2.14. [Google Scholar] [CrossRef]
101. Al-Jarrah, M., Al-Dweik, A., Alsusa, E., Iraqi, Y., Alouini, M. S. (2021). On the performance of irs-assisted multi-layer uav communications with imperfect phase compensation. IEEE Transactions on Communications, 69(12), 8551–8568. DOI 10.1109/TCOMM.2021.3113008. [Google Scholar] [CrossRef]
102. Cao, H., Zhu, W., Feng, W., Fan, J. (2022). Robust beamforming based on graph attention networks for IRS-assisted satellite IoT communications. Entropy, 24(3), 326. DOI 10.3390/e24030326. [Google Scholar] [CrossRef]
103. Zheng, B., Lin, S., Zhang, R. (2022). Intelligent reflecting surface-aided LEO satellite communication: Cooperative passive beamforming and distributed channel estimation. arXiv preprint arXiv:2201.02913. [Google Scholar]
104. Pang, X., Sheng, M., Zhao, N., Tang, J., Niyato, D. et al. (2021). When UAV meets IRS: Expanding air-ground networks via passive reflection. IEEE Wireless Communications, 28(5), 164–170. DOI 10.1109/MWC.010.2000528. [Google Scholar] [CrossRef]
105. Mao, M., Cao, N., Li, R., Shi, R. (2021). IRS-assisted low altitude passive aerial relaying. Computer Communications, 175(3), 150–155. DOI 10.1016/j.comcom.2021.05.001. [Google Scholar] [CrossRef]
106. Khan, M. R. H., Ziad, M. O., Chowa, S. M., Mehedi, M. M. (2021). A comparative study of intelligent reflecting surface and relay in satellite communication. Brac University, Dhaka, Bangladesh.http://dspace.bracu.ac.bd/xmlui/handle/10361/14788. [Google Scholar]
107. de Sanctis, M., Cianca, E., Araniti, G., Bisio, I., Prasad, R. (2015). Satellite communications supporting Internet of Remote Things. IEEE Internet of Things Journal, 3(1), 113–123. DOI 10.1109/JIOT.2015.2487046. [Google Scholar] [CrossRef]
108. Kim, M. G., Jo, H. S. (2022). Problem and solution for NB-IoT uplink in low earth orbit satellite communication. 2022 International Conference on Electronics, Information, and Communication (ICEIC), Jeju, South Korea, IEEE. [Google Scholar]
109. Marchese, M., Moheddine, A., Patrone, F. (2019). IoT and UAV integration in 5G hybrid terrestrial-satellite networks. Sensors, 19(17), 3704. DOI 10.3390/s19173704. [Google Scholar] [CrossRef]
110. Guidotti, A., Conti, M., Vanelli-Coralli, A. (2021). Beamforming in LEO constellations for NB-IoT services in 6G communications. arXiv preprint arXiv: 2103.13348. [Google Scholar]
111. Akyildiz, I. F., Kak, A. (2019). The internet of space things/cubesats: A ubiquitous cyber-physical system for the connected world. Computer Networks, 150(3), 134–149. DOI 10.1016/j.comnet.2018.12.017. [Google Scholar] [CrossRef]
112. Kak, A., Akyildiz, I. F. (2021). Towards automatic network slicing for the Internet of Space Things. IEEE Transactions on Network and Service Management, 19(1), 392–412. DOI 10.1109/TNSM.2021.3117692. [Google Scholar] [CrossRef]
113. Priyadarshini, I., Bhola, B., Kumar, R., So-In, C. (2022). A novel cloud architecture for Internet of Space Things (IoST). IEEE Access, 10, 15118–15134. DOI 10.1109/ACCESS.2022.3144137. [Google Scholar] [CrossRef]
114. Ilchenko, M., Narytnyk, T., Prisyazhny, V., Kapshtyk, S., Matvienko, S. (2021). The Internet of Things space infrastructure. Current state and development prospects. In: Internet of things. London, UK: IntechOpen Limited. [Google Scholar]
115. Nguyen, T. K., Nguyen, C. T., Le, H. D., Pham, A. T. (2021). Tcp performance over satellite-based hybrid FSO/RF vehicular networks: Modeling and analysis. IEEE Access, 9, 108426–108440. DOI 10.1109/ACCESS.2021.3101903. [Google Scholar] [CrossRef]
116. Zhen, L., Wang, Y., Yu, K., Lu, G., Mumtaz, Z. et al. (2021). Reliable uplink synchronization maintenance for satellite-ground integrated vehicular networks: A high-order statistics-based timing advance update approach. IEEE Transactions on Intelligent Transportation Systems, 1–14. DOI 10.1109/TITS.2021.3131816. [Google Scholar] [CrossRef]
117. He, Y., Wang, Y., Yu, F. R., Lin, Q., Li, J. et al. (2021). Efficient resource allocation for multi-beam satellite-terrestrial vehicular networks: A multi-agent actor-critic method with attention mechanism. IEEE Transactions on Intelligent Transportation Systems, 23(3), 2727–2738. DOI 10.1109/TITS.2021.3128209. [Google Scholar] [CrossRef]
118. Niu, Z., Shen, X. S., Zhang, Q., Tang, Y. (2020). Space-air-ground integrated vehicular network for connected and automated vehicles: Challenges and solutions. Intelligent and Converged Networks, 1(2), 142–169. DOI 10.23919/ICN.2020.0009. [Google Scholar] [CrossRef]
119. Wang, J., Wang, Y., Zhang, D., Lv, Q., Chen, C. (2019). Crowd-powered sensing and actuation in smart cities: Current issues and future directions. IEEE Wireless Communications, 26(2), 86–92. DOI 10.1109/MWC.2019.1800030. [Google Scholar] [CrossRef]
120. Priyadarsini, M., Mittal, P., Bera, P. (2020). Smart city renovation using SDN framework. 2020 International Conference on Communication Systems & NETworkS (COMSNETS), Bengaluru, India, IEEE. [Google Scholar]
121. Hornillo-Mellado, S., Martín-Clemente, R., Baena-Lecuyer, V. (2020). Prediction of satellite shadowing in smart cities with application to IoT. Sensors, 20(2), 475. DOI 10.3390/s20020475. [Google Scholar] [CrossRef]
122. Sharma, S. K., Chatzinotas, S., Jayakody, D., Anpalagan, A. (2021). Communication technologies for networked smart cities. Communication Technologies for Networked Smart Cities, 1–345. The Institution of Engineering and Technology, UK.https://www.amazon.com/Communication-Technologies-Networked-Cities-Telecommunications/dp/1839530294. [Google Scholar]
123. Akyildiz, I. F., Han, C., Hu, Z., Nie, S., Jornet, J. M. (2022). Terahertz band communication: An old problem revisited and research directions for the next decade. IEEE Transactions on Communications, 70(6), 4250–4285. DOI 10.1109/TCOMM.2022.3171800. [Google Scholar] [CrossRef]
124. Marcus, M. J., Kürner, T. (2022). Spectrum for THz communications. In: THz communications, pp. 515–527. Cham, Switzerland: Springer. [Google Scholar]
125. Jahid, A., Alsharif, M. H., Hall, T. J. (2022). A contemporary survey on free space optical communication: Potentials, technical challenges, recent advances and research direction. Journal of Network and Computer Applications, 200(9), 103311. DOI 10.1016/j.jnca.2021.103311. [Google Scholar] [CrossRef]
126. Rasti, M., Taskou, S. K., Tabassum, H., Hossain, E. (2022). Evolution toward 6G multi-band wireless networks: A resource management perspective. IEEE Wireless Communications, 29(4), 118–125. [Google Scholar]
127. Xing, Y., Rappaport, T. S. (2021). Terahertz wireless communications: Co-sharing for terrestrial and satellite systems above 100 GHz. IEEE Communications Letters, 25(10), 3156–3160. DOI 10.1109/LCOMM.2021.3088270. [Google Scholar] [CrossRef]
128. Raza, A., Ijaz, U., Ishfaq, M. K., Ahmad, S., Liaqat, M. et al. (2022). Intelligent reflecting surface-assisted terahertz communication towards B5G and 6G: State-of-the-art. Microwave and Optical Technology Letters, 64(5), 858–866. DOI 10.1002/mop.33185. [Google Scholar] [CrossRef]
129. Kumar, R., Arnon, S. (2022). Snr optimization for LEO satellite at sub-THz frequencies. IEEE Transactions on Antennas and Propagation, 70(6), 4449–4458. DOI 10.1109/TAP.2022.3140217. [Google Scholar] [CrossRef]
130. Eishima, T., Inoue, S., Yonemoto, A., Sudo, J., Hosonuma, T. et al. (2022). RF and optical hybrid LEO communication system for non-terrestrial network. 2022 IEEE International Conference on Space Optical Systems and Applications (ICSOS), Kyoto City, Japan, IEEE. [Google Scholar]
131. Ndiaye, M., Saley, A. M., Niane, K., Raimy, A. (2022). Future 6G communication networks: Typical iot network topology and terahertz frequency challenges and research issues. 2022 2nd International Conference on Innovative Research in Applied Science, Engineering and Technology (IRASET), Meknes, Morocco, IEEE. [Google Scholar]
132. Akyildiz, I. F., Jornet, J. M., Han, C. (2014). Terahertz band: Next frontier for wireless communications. Physical Communication, 12(7), 16–32. DOI 10.1016/j.phycom.2014.01.006. [Google Scholar] [CrossRef]
133. Solyman, A. A., Elhaty, I. A. (2021). Potential key challenges for terahertz communication systems. International Journal of Electrical & Computer Engineering, 11(4), 3403–3409. DOI 10.11591/ijece.v11i4.pp3403-3409. [Google Scholar] [CrossRef]
134. Tekbıyık, K., Ekti, A. R., Kurt, G. K., Görçin, A. (2019). Terahertz band communication systems: Challenges, novelties and standardization efforts. Physical Communication, 35(7), 100700. DOI 10.1016/j.phycom.2019.04.014. [Google Scholar] [CrossRef]
135. Gao, X., Dai, L., Zhang, Y., Xie, T., Dai, X. et al. (2016). Fast channel tracking for terahertz beamspace massive MIMO systems. IEEE Transactions on Vehicular Technology, 66(7), 5689–5696. DOI 10.1109/TVT.2016.2614994. [Google Scholar] [CrossRef]
136. Liu, K., Jia, S., Wang, S., Pang, X., Li, W. et al. (2018). 100 Gbit/s THz photonic wireless transmission in the 350-GHz band with extended reach. IEEE Photonics Technology Letters, 30(11), 1064–1067. DOI 10.1109/LPT.2018.2830342. [Google Scholar] [CrossRef]
137. Han, C., Akyildiz, I. F., Gerstacker, W. H. (2017). Timing acquisition and error analysis for pulse-based terahertz band wireless systems. IEEE Transactions on Vehicular Technology, 66(11), 10102–10113. DOI 10.1109/TVT.2017.2750707. [Google Scholar] [CrossRef]
138. Li, K. X., Wang, J., Gao, X., Tsinos, C. G., Ottersten, B. (2022). Uplink transmit design for massive MIMO LEO satellite communications. arXiv preprint arXiv:2201.12940. [Google Scholar]
139. Shen, B., Wu, Y., An, J., Xing, C., Zhao, L. et al. (2022). Random access with massive MIMO-OTFS in LEO satellite communications. arXiv preprint arXiv: 2202.13058. [Google Scholar]
140. You, L., Qiang, X., Li, K. X., Tsinos, C. G., Wang, W. et al. (2022). Hybrid analog/digital precoding for downlink massive MIMO LEO satellite communications. IEEE Transactions on Wireless Communications, 70(8), 5543–5557. DOI 10.1109/TCOMM.2022.3182757. [Google Scholar] [CrossRef]
141. Busari, S. A., Huq, K. M. S., Mumtaz, S., Dai, L., Rodriguez, J. (2017). Millimeter-wave massive MIMO communication for future wireless systems: A survey. IEEE Communications Surveys & Tutorials, 20(2), 836–869. DOI 10.1109/COMST.2017.2787460. [Google Scholar] [CrossRef]
142. You, L., Qiang, X., Tsinos, C. G., Liu, F., Wang, W. et al. (2022). Beam squint-aware integrated sensing and communications for hybrid massive MIMO LEO satellite systems. arXiv preprint arXiv: 2203.00235. [Google Scholar]
143. Riera-Palou, F., Femenias, G., Caus, M., Shaat, M., Pérez-Neira, A. I. (2022). Scalable cell-free massive MIMO networks with LEO satellite support. IEEE Access, 10, 37557–37571. DOI 10.1109/ACCESS.2022.3164097. [Google Scholar] [CrossRef]
144. Papadopoulos, H., Wang, C., Bursalioglu, O., Hou, X., Kishiyama, Y. (2016). Massive MIMO technologies and challenges towards 5G. IEICE Transactions on Communications, 99(3), 602–621. DOI 10.1587/transcom.2015EBI0002. [Google Scholar] [CrossRef]
145. Borges, D., Montezuma, P., Dinis, R., Beko, M. (2021). Massive MIMO techniques for 5G and beyond-opportunities and challenges. Electronics, 10(14), 1667. DOI 10.3390/electronics10141667. [Google Scholar] [CrossRef]
146. Chataut, R., Akl, R. (2020). Massive mimo systems for 5G and beyond networks-overview, recent trends, challenges, and future research direction. Sensors, 20(10), 2753. DOI 10.3390/s20102753. [Google Scholar] [CrossRef]
147. Fourati, F., Alouini, M. S. (2021). Artificial intelligence for satellite communication: A review. Intelligent and Converged Networks, 2(3), 213–243. DOI 10.23919/ICN.2021.0015. [Google Scholar] [CrossRef]
148. Chen, H., Xiao, M., Pang, Z. (2022). Satellite-based computing networks with federated learning. IEEE Wireless Communications, 29(1), 78–84. DOI 10.1109/MWC.008.00353. [Google Scholar] [CrossRef]
149. Takahashi, Y., Saito, M., Oshima, N., Yamada, K. (2022). Trajectory reconstruction for nanosatellite in very low earth orbit using machine learning. Acta Astronautica, 194(1), 301–308. DOI 10.1016/j.actaastro.2022.02.010. [Google Scholar] [CrossRef]
150. Razmi, N., Matthiesen, B., Dekorsy, A., Popovski, P. (2022). Ground-assisted federated learning in LEO satellite constellations. IEEE Wireless Communications Letters, 11(4), 717–721. [Google Scholar]
151. Gu, Y., Wu, Z., Li, X., Tian, R., Ma, S. et al. (2022). Modulation format identification in a satellite to ground optical wireless communication systems using a convolution neural network. Applied Sciences, 12(7), 3331. DOI 10.3390/app12073331. [Google Scholar] [CrossRef]
152. Islam, T. N., Sabuj, S. R. (2022). Compensation layer-added federated learning receiver: Design and implementation. Internet Technology Letters, e389. DOI 10.1002/itl2.389. [Google Scholar] [CrossRef]
153. So, J., Hsieh, K., Arzani, B., Noghabi, S., Avestimehr, S. et al. (2022). Fedspace: An efficient federated learning framework at satellites and ground stations. arXiv preprint arXiv: 2202.01267. [Google Scholar]
154. Vázquez, M. Á., Henarejos, P., Pappalardo, I., Grechi, E., Fort, J. et al. (2021). Machine learning for satellite communications operations. IEEE Communications Magazine, 59(2), 22–27. DOI 10.1109/MCOM.001.2000367. [Google Scholar] [CrossRef]
155. Ortiz-Gomez, F. G., Lei, L., Lagunas, E., Martinez, R., Tarchi, D. et al. (2022). Machine learning for radio resource management in multibeam geo satellite systems. Electronics, 11(7), 992. DOI 10.3390/electronics11070992. [Google Scholar] [CrossRef]
156. Tong, W., Li, G. Y. (2022). Nine challenges in artificial intelligence and wireless communications for 6G. IEEE Wireless Communications, 29(4), 140–145. [Google Scholar]
157. Li, F., Li, G., Li, Z., Wang, Y., Zhang, G. (2016). A novel approach to wideband spectrum compressive sensing based on DST for frequency availability in LEO mobile satellite systems. Mathematical Problems in Engineering, 2016, 1–13. DOI 10.1155/2016/8397201. [Google Scholar] [CrossRef]
158. Shivanna, G. K., Prasantha, H. S. (2022). Two-dimensional satellite image compression using compressive sensing. International Journal of Electrical & Computer Engineering, 12(1), 311. DOI 10.11591/ijece.v12i1.pp311-319. [Google Scholar] [CrossRef]
159. Wan, Z., Gao, Z., Masouros, C., Ng, D. W. K., Chen, S. (2022). Integrated sensing and communication with mmwave massive MIMO: A compressed sampling perspective. arXiv preprint arXiv: 2201.05766. [Google Scholar]
160. Daponte, P., de Vito, L., Picariello, F., Rapuano, S., Tudosa, I. (2018). Compressed sensing technologies and challenges for aerospace and defense RF source localization. 2018 5th IEEE International Workshop on Metrology for AeroSpace (MetroAeroSpace), IEEE. [Google Scholar]
161. Zhu, D., Zhu, H., Wang, Z., Zhang, Y. (2021). Three-level quantum satellite communication framework and its applications. International Journal of Satellite Communications and Networking, 39(5), 473–485. DOI 10.1002/sat.1392. [Google Scholar] [CrossRef]
162. Chiti, F., Fantacci, R., Picchi, R., Pierucci, L. (2022). Mobile control plane design for quantum satellite backbones. IEEE Network, 36(1), 91–97. DOI 10.1109/MNET.012.2100167. [Google Scholar] [CrossRef]
163. Kurochkin, V., Khmelev, A., Mayboroda, V., Bakhshaliev, R., Duplinsky, A. et al. (2022). Elements of satellite quantum network. International Conference on Micro- and Nano-Electronics 2021, 12157T. Zvenigorod, Russian Federation, SPIE. [Google Scholar]
164. Xu, M., Niyato, D., Xiong, Z., Kang, J., Cao, X. et al. (2022). Quantum-secured space-air-ground integrated networks: Concept, framework, and case study. arXiv preprint arXiv: 2204.08673. [Google Scholar]
165. Pirandola, S. (2021). Satellite quantum communications: Fundamental bounds and practical security. Physical Review Research, 3(2), 023130. DOI 10.1103/PhysRevResearch.3.023130. [Google Scholar] [CrossRef]
166. Wang, C., Rahman, A. (2022). Quantum-enabled 6G wireless networks: Opportunities and challenges. IEEE Wireless Communications, 29(1), 58–69. DOI 10.1109/MWC.006.00340. [Google Scholar] [CrossRef]
167. Mamiya, A., Tanaka, K., Yokote, S., Sasaki, M., Fujiwara, M. et al. (2022). Satellite-based QKD for global quantum cryptographic network construction. 2022 IEEE International Conference on Space Optical Systems and Applications (ICSOS), Kyoto City, Japan, IEEE. [Google Scholar]
168. Harney, C., Pirandola, S. (2022). Analytical methods for high-rate global quantum networks. PRX Quantum, 3(1), 010349. DOI 10.1103/PRXQuantum.3.010349. [Google Scholar] [CrossRef]
169. Khalif, B. N., Hasan, J. A., Alhumaima, R. S., Al-Raweshidy, H. S. (2019). Performance analysis of quantum based cloud radio access networks. IEEE Access, 8, 18123–18133. DOI 10.1109/ACCESS.2019.2925902. [Google Scholar] [CrossRef]
170. Bedington, R., Arrazola, J. M., Ling, A. (2017). Progress in satellite quantum key distribution. npj Quantum Information, 3(1), 1–13. DOI 10.1038/s41534-017-0031-5. [Google Scholar] [CrossRef]
171. Sidhu, J. S., Joshi, S. K., Gündoğan, M., Brougham, T., Lowndes, D. et al. (2021). Advances in space quantum communications. IET Quantum Communication, 2(4), 182–217. DOI 10.1049/qtc2.12015. [Google Scholar] [CrossRef]
172. Khan, I. (2018). Quantum communication in space-challenges and opportunities. Applied Industrial Optics: Spectroscopy, Imaging and Metrology, AM5A.2. DOI 10.1364/AIO.2018.AM5A.2. [Google Scholar] [CrossRef]
173. Zhang, Q., Xu, F., Chen, Y. A., Peng, C. Z., Pan, J. W. (2018). Large scale quantum key distribution: Challenges and solutions. Optics Express, 26(18), 24260–24273. DOI 10.1364/OE.26.024260. [Google Scholar] [CrossRef]
Cite This Article
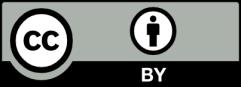
This work is licensed under a Creative Commons Attribution 4.0 International License , which permits unrestricted use, distribution, and reproduction in any medium, provided the original work is properly cited.