Open Access
EDITORIAL
Introduction to the Special Issue on New Trends in Statistical Computing and Data Science
1 Department of Mathematics, LMNO, University of Caen, Caen, 14032, France
2 School of Mathematical and Physical Sciences, Macquarie University, Sydney, 2109, Australia
* Corresponding Author: Christophe Chesneau. Email:
(This article belongs to the Special Issue: New Trends in Statistical Computing and Data Science)
Computer Modeling in Engineering & Sciences 2023, 136(2), 981-983. https://doi.org/10.32604/cmes.2023.028283
Received 09 December 2022; Accepted 12 December 2022; Issue published 06 February 2023
Abstract
This article has no abstract.The interface between computer science and statistics has developed considerably in recent years, with exponential progress in the fields of data analysis, stochastic modeling, machine learning, econometrics, simulation, algorithms, classification, and networks. Innovative discoveries in this field appear every day, opening new scientific horizons for the modern world. This is especially true in the post-2020 period, with the treatment of large volumes of data that feed the daily operations of large corporations, as well as the development of artificial intelligence, including advanced machine learning techniques, particularly “deep learning”.
The aim of the special issue titled “New Trends in Statistical Computing and Data Science” was to publish the most significant articles in these directions, that is, the current progress of statistical computing and data science. Novelty, high quality, and importance are the triptych of the special issue.
After a thorough peer review procedure implying top assistants and reviewers, 11 articles were chosen for the final special issue. These 11 articles, which were produced by academics from different countries, present the state of current research initiatives and developments in statistical computing and data science.
The first paper “Determination of Monitoring Control Value for Concrete Gravity Dam Spatial Deformation Based on POT Model” by Xie et al. [1] introduced a novel approach to calculating the monitoring control value for a concrete gravity dam using the deformations of numerous measuring points.
The second paper “A Novel Modified Alpha Power Transformed Weibull Distribution and Its Engineering Applications” by Alotaibi et al. [2] proposed a new modified version of the conventional Weibull distribution by introducing a new shape parameter and using the modified alpha power transformed method, with applications to real lifetime data sets.
In the third paper entitled “Ranked-Set Sampling Based Distribution Free Control Chart with Application in CSTR Process” by Almanjahie et al. [3], the authors suggested a nonparametric homogeneously weighted moving average for detecting shifts in the process location of a continuous and symmetric distribution based on the Wilcoxon signed-rank test with ranked set sampling control chart.
In their paper “A Two-Step Algorithm to Estimate Variable Importance for Multi-State Data: An Application to COVID-19”, Alafchi et al. [4] presented a two-step algorithm with an application to a contemporary COVID data set for assessing the significance of variables for multi-state data.
In their paper “A New Three-Parameter Inverse Weibull Distribution with Medical and Engineering Applications”, Alotaibi et al. [5] offered an innovative extension to the traditional inverse Weibull distribution that increases flexibility by introducing an additional shape parameter, with applications to medical and engineering data.
The sixth paper “Bayesian Computation for the Parameters of a Zero-Inflated Cosine Geometric Distribution with Application to COVID-19 Pandemic Data” by Junnumtuam et al. [6] created a brand-new discrete three-parameter distribution known as the zero-inflated cosine geometric distribution, and apply the resulting count model to a COVID-19 data set.
In the seventh paper “New Hybrid EWMA Charts for Efficient Process Dispersion Monitoring with Application in Automobile Industry” written by Liu et al. [7], the authors intended to create two new upper-sided HEWMA charts, known as the HEWMA1 and HEWMA2 charts, for monitoring shifts in process variance, and comparison shows that the proposed charts outperform the existing charts in terms of detection ability.
Ibrahim et al. [8], in their paper “Improving Date Fruit Classification Using CycleGAN-Generated Dataset”, used Deep Convolutional Generative Adversarial Networks (DCGAN) and CycleGAN to enhance our collected date fruit datasets.
In their paper “Wavelet Decomposition Impacts on Traditional Forecasting Time Series Models”, Shaikh et al. [9] looked into how wavelet algorithms affect more conventional time-series forecasting models, and it is noted that, on a performance basis, the wavelet combined models are superior to the different time series models.
In the tenth paper entitled “Time Synchronized Velocity Error for Trajectory Compression” written by Jiang et al. [10], the authors suggested a novel algorithm, SW-MSVE (Minimum Time Synchronized Velocity Error Based on Sliding Window), which can reduce the velocity error in compressed trajectory under the assumption of local optimization.
Finally, Algarni et al. [11] in “A New Rayleigh Distribution: Properties and Estimation Based on Progressive Type-II Censored Data with an Application”, developed a fresh extension of the conventional Rayleigh distribution, with an application to one real data for failure times of software and fair comparisons with other existing models.
As a final remark, we wish this special issue can help to disseminate new ideas, both in terms of statistical methods and data processing, and inspires future generations for new and important discoveries.
Acknowledgement: We want to express our gratitude to the authors for their great work on this special edition.
Funding Statement: The authors received no specific funding.
Conflicts of Interest: The authors declare that they have no conflicts of interest to report regarding the present study.
References
1. Xie, Z., Yu, T. (2023). Determination of monitoring control value for concrete gravity dam spatial deformation based on POT model. Computer Modeling in Engineering & Sciences, 135(3), 2119–2135. DOI 10.32604/cmes.2023.025070. [Google Scholar] [CrossRef]
2. Alotaibi, R., Okasha, H., Nassar, M., Elshahhat, A. (2023). A novel modified alpha power transformed weibull distribution and its engineering applications. Computer Modeling in Engineering & Sciences, 135(3), 2065–2089. DOI 10.32604/cmes.2023.023408. [Google Scholar] [CrossRef]
3. Almanjahie, I. M., Rasheed, Z., Khan, M., Anwar, S. M., Cheema, A. N. (2023). Ranked-set sampling based distribution free control chart with application in CSTR process. Computer Modeling in Engineering & Sciences, 135(3), 2091–2118. DOI 10.32604/cmes.2023.022201. [Google Scholar] [CrossRef]
4. Alafchi, B., Tapak, L., Doosti, H., Chesneau, C., Roshanaei, G. (2023). A two-step algorithm to estimate variable importance for multi-state data: An application to COVID-19. Computer Modeling in Engineering & Sciences, 135(3), 2047–2064. DOI 10.32604/cmes.2022.022647. [Google Scholar] [CrossRef]
5. Alotaibi, R., Okasha, H., Rezk, H., Nassar, M. (2023). A new three-parameter inverse weibull distribution with medical and engineering applications. Computer Modeling in Engineering & Sciences, 135(2), 1255–1274. DOI 10.32604/cmes.2022.022623. [Google Scholar] [CrossRef]
6. Junnumtuam, S., Niwitpong, S., Niwitpong, S. (2023). Bayesian computation for the parameters of a zero-inflated cosine geometric distribution with application to COVID-19 pandemic data. Computer Modeling in Engineering & Sciences, 135(2), 1229–1254. DOI 10.32604/cmes.2022.022098. [Google Scholar] [CrossRef]
7. Liu, X., Khan, M., Rasheed, Z., Anwar, S. M., Arslan, M. (2022). New hybrid EWMA charts for efficient process dispersion monitoring with application in automobile industry. Computer Modeling in Engineering & Sciences, 131(2), 1171–1195. DOI 10.32604/cmes.2022.019199. [Google Scholar] [CrossRef]
8. Ibrahim, D. M., Elshennawy, N. M. (2022). Improving date fruit classification using CycleGAN-Generated dataset. Computer Modeling in Engineering & Sciences, 131(1), 331–348. DOI 10.32604/cmes.2022.016419. [Google Scholar] [CrossRef]
9. Shaikh, W. A., Shah, S. F., Pandhiani, S. M., Solangi, M. A. (2022). Wavelet Decomposition impacts on traditional forecasting time series models. Computer Modeling in Engineering & Sciences, 130(3), 1517–1532. DOI 10.32604/cmes.2022.017822. [Google Scholar] [CrossRef]
10. Jiang, H., Han, D., Liu, H., Han, J. (2022). Time synchronized velocity error for trajectory compression. Computer Modeling in Engineering & Sciences, 130(2), 1193–1219. DOI 10.32604/cmes.2022.017663. [Google Scholar] [CrossRef]
11. Algarni, A., Almarashi, A. M. (2022). A new rayleigh distribution: properties and estimation based on progressive type-II censored data with an application. Computer Modeling in Engineering & Sciences, 130(1), 379–396. DOI 10.32604/cmes.2022.017714. [Google Scholar] [CrossRef]
Cite This Article
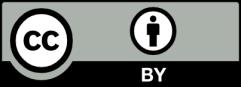
This work is licensed under a Creative Commons Attribution 4.0 International License , which permits unrestricted use, distribution, and reproduction in any medium, provided the original work is properly cited.