Open Access
ARTICLE
GraphCWGAN-GP: A Novel Data Augmenting Approach for Imbalanced Encrypted Traffic Classification
1 School of Electronics and Information Engineering, Nanjing University of Information Science and Technology, Nanjing, 210044, China
2 School of Tourism and Geography Science, Qingdao University, Qingdao, 266071, China
* Corresponding Author: Jiangtao Zhai. Email:
(This article belongs to the Special Issue: Cyberspace Intelligent Mapping and Situational Awareness)
Computer Modeling in Engineering & Sciences 2023, 136(2), 2069-2092. https://doi.org/10.32604/cmes.2023.023764
Received 14 May 2022; Accepted 14 September 2022; Issue published 06 February 2023
Abstract
Encrypted traffic classification has become a hot issue in network security research. The class imbalance problem of traffic samples often causes the deterioration of Machine Learning based classifier performance. Although the Generative Adversarial Network (GAN) method can generate new samples by learning the feature distribution of the original samples, it is confronted with the problems of unstable training and mode collapse. To this end, a novel data augmenting approach called GraphCWGAN-GP is proposed in this paper. The traffic data is first converted into grayscale images as the input for the proposed model. Then, the minority class data is augmented with our proposed model, which is built by introducing conditional constraints and a new distance metric in typical GAN. Finally, the classical deep learning model is adopted as a classifier to classify datasets augmented by the Condition GAN (CGAN), Wasserstein GAN-Gradient Penalty (WGAN-GP) and GraphCWGAN-GP, respectively. Compared with the state-of-the-art GAN methods, the GraphCWGAN-GP cannot only control the modes of the data to be generated, but also overcome the problem of unstable training and generate more realistic and diverse samples. The experimental results show that the classification precision, recall and F1-Score of the minority class in the balanced dataset augmented in this paper have improved by more than 2.37%, 3.39% and 4.57%, respectively.Keywords
Cite This Article
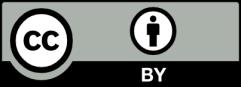