Open Access
ARTICLE
Solving Geometry Problems via Feature Learning and Contrastive Learning of Multimodal Data
1 North China University of Water Resources and Electric Power, Zhengzhou, 450046, China
2 Henan University of Economics and Law, Zhengzhou, 450046, China
* Corresponding Author: Fucheng Guo. Email:
(This article belongs to the Special Issue: Humanized Computing and Reasoning in Teaching and Learning)
Computer Modeling in Engineering & Sciences 2023, 136(2), 1707-1728. https://doi.org/10.32604/cmes.2023.023243
Received 15 April 2022; Accepted 23 September 2022; Issue published 06 February 2023
Abstract
This paper presents an end-to-end deep learning method to solve geometry problems via feature learning and contrastive learning of multimodal data. A key challenge in solving geometry problems using deep learning is to automatically adapt to the task of understanding single-modal and multimodal problems. Existing methods either focus on single-modal or multimodal problems, and they cannot fit each other. A general geometry problem solver should obviously be able to process various modal problems at the same time. In this paper, a shared feature-learning model of multimodal data is adopted to learn the unified feature representation of text and image, which can solve the heterogeneity issue between multimodal geometry problems. A contrastive learning model of multimodal data enhances the semantic relevance between multimodal features and maps them into a unified semantic space, which can effectively adapt to both single-modal and multimodal downstream tasks. Based on the feature extraction and fusion of multimodal data, a proposed geometry problem solver uses relation extraction, theorem reasoning, and problem solving to present solutions in a readable way. Experimental results show the effectiveness of the method.Keywords
Cite This Article
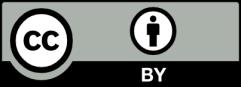