Open Access
ARTICLE
Logformer: Cascaded Transformer for System Log Anomaly Detection
1 Information Center, Yunnan Power Grid Company Limited, Kunming, 650034, China
2 Network and Data Security Key Laboratory of Sichuan Province, University of Electronic Science and Technology of China, Chengdu, 610054, China
* Corresponding Author: Chenghao Zhou. Email:
(This article belongs to the Special Issue: Information Security Practice and Experience: Advances and Challenges)
Computer Modeling in Engineering & Sciences 2023, 136(1), 517-529. https://doi.org/10.32604/cmes.2023.025774
Received 29 July 2022; Accepted 14 September 2022; Issue published 05 January 2023
Abstract
Modern large-scale enterprise systems produce large volumes of logs that record detailed system runtime status and key events at key points. These logs are valuable for analyzing performance issues and understanding the status of the system. Anomaly detection plays an important role in service management and system maintenance, and guarantees the reliability and security of online systems. Logs are universal semi-structured data, which causes difficulties for traditional manual detection and pattern-matching algorithms. While some deep learning algorithms utilize neural networks to detect anomalies, these approaches have an over-reliance on manually designed features, resulting in the effectiveness of anomaly detection depending on the quality of the features. At the same time, the aforementioned methods ignore the underlying contextual information present in adjacent log entries. We propose a novel model called Logformer with two cascaded transformer-based heads to capture latent contextual information from adjacent log entries, and leverage pre-trained embeddings based on logs to improve the representation of the embedding space. The proposed model achieves comparable results on HDFS and BGL datasets in terms of metric accuracy, recall and F1-score. Moreover, the consistent rise in F1-score proves that the representation of the embedding space with pre-trained embeddings is closer to the semantic information of the log.Keywords
Cite This Article
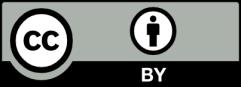
This work is licensed under a Creative Commons Attribution 4.0 International License , which permits unrestricted use, distribution, and reproduction in any medium, provided the original work is properly cited.