Open Access
ARTICLE
Health Monitoring of Milling Tool Inserts Using CNN Architectures Trained by Vibration Spectrograms
Department of Mechanical Engineering, College of Engineering Pune, Pune, 411005, India
* Corresponding Author: Abhishek D. Patange. Email:
Computer Modeling in Engineering & Sciences 2023, 136(1), 177-199. https://doi.org/10.32604/cmes.2023.025516
Received 18 July 2022; Accepted 20 September 2022; Issue published 05 January 2023
Abstract
In-process damage to a cutting tool degrades the surface finish of the job shaped by machining and causes a significant financial loss. This stimulates the need for Tool Condition Monitoring (TCM) to assist detection of failure before it extends to the worse phase. Machine Learning (ML) based TCM has been extensively explored in the last decade. However, most of the research is now directed toward Deep Learning (DL). The “Deep” formulation, hierarchical compositionality, distributed representation and end-to-end learning of Neural Nets need to be explored to create a generalized TCM framework to perform efficiently in a high-noise environment of cross-domain machining. With this motivation, the design of different CNN (Convolutional Neural Network) architectures such as AlexNet, ResNet-50, LeNet-5, and VGG-16 is presented in this paper. Real-time spindle vibrations corresponding to healthy and various faulty configurations of milling cutter were acquired. This data was transformed into the time-frequency domain and further processed by proposed architectures in graphical form, i.e., spectrogram. The model is trained, tested, and validated considering different datasets and showcased promising results.Keywords
Cite This Article
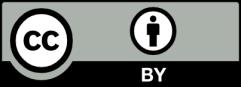
This work is licensed under a Creative Commons Attribution 4.0 International License , which permits unrestricted use, distribution, and reproduction in any medium, provided the original work is properly cited.