Open Access
ARTICLE
Image Semantic Segmentation for Autonomous Driving Based on Improved U-Net
College of Mechanical and Electrical Engineering, Qingdao University, Qingdao, 266071, China
* Corresponding Author: Hong Zhao. Email:
(This article belongs to the Special Issue: Computing Methods for Industrial Artificial Intelligence)
Computer Modeling in Engineering & Sciences 2023, 136(1), 787-801. https://doi.org/10.32604/cmes.2023.025119
Received 04 July 2022; Accepted 06 September 2022; Issue published 05 January 2023
Abstract
Image semantic segmentation has become an essential part of autonomous driving. To further improve the generalization ability and the robustness of semantic segmentation algorithms, a lightweight algorithm network based on Squeeze-and-Excitation Attention Mechanism (SE) and Depthwise Separable Convolution (DSC) is designed. Meanwhile, Adam-GC, an Adam optimization algorithm based on Gradient Compression (GC), is proposed to improve the training speed, segmentation accuracy, generalization ability and stability of the algorithm network. To verify and compare the effectiveness of the algorithm network proposed in this paper, the trained network model is used for experimental verification and comparative test on the Cityscapes semantic segmentation dataset. The validation and comparison results show that the overall segmentation results of the algorithm network can achieve 78.02% MIoU on Cityscapes validation set, which is better than the basic algorithm network and the other latest semantic segmentation algorithms network. Besides meeting the stability and accuracy requirements, it has a particular significance for the development of image semantic segmentation.Keywords
Cite This Article
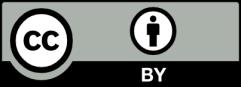
This work is licensed under a Creative Commons Attribution 4.0 International License , which permits unrestricted use, distribution, and reproduction in any medium, provided the original work is properly cited.